When Deep Classifiers Agree: Analyzing Correlations Between Learning Order and Image Statistics.
European Conference on Computer Vision(2022)
摘要
Although a plethora of architectural variants for deep classification has been introduced over time, recent works have found empirical evidence towards similarities in their training process. It haswrapfig been hypothesized that neural networks converge not only to similar representations, but also exhibit a notion of empirical agreement on which data instances are learned first. Following in the latter works’ footsteps, we define a metric to quantify the relationship between such classification agreement over time, and posit that the agreement phenomenon can be mapped to core statistics of the investigated dataset. We empirically corroborate this hypothesis across the CIFAR10, Pascal, ImageNet and KTH-TIPS2 datasets. Our findings indicate that agreement seems to be independent of specific architectures, training hyper-parameters or labels, albeit follows an ordering according to image statistics.
更多查看译文
关键词
Neural network learning dynamics,Deep classifier agreement,Data instance ordering,Image dataset statistics
AI 理解论文
溯源树
样例
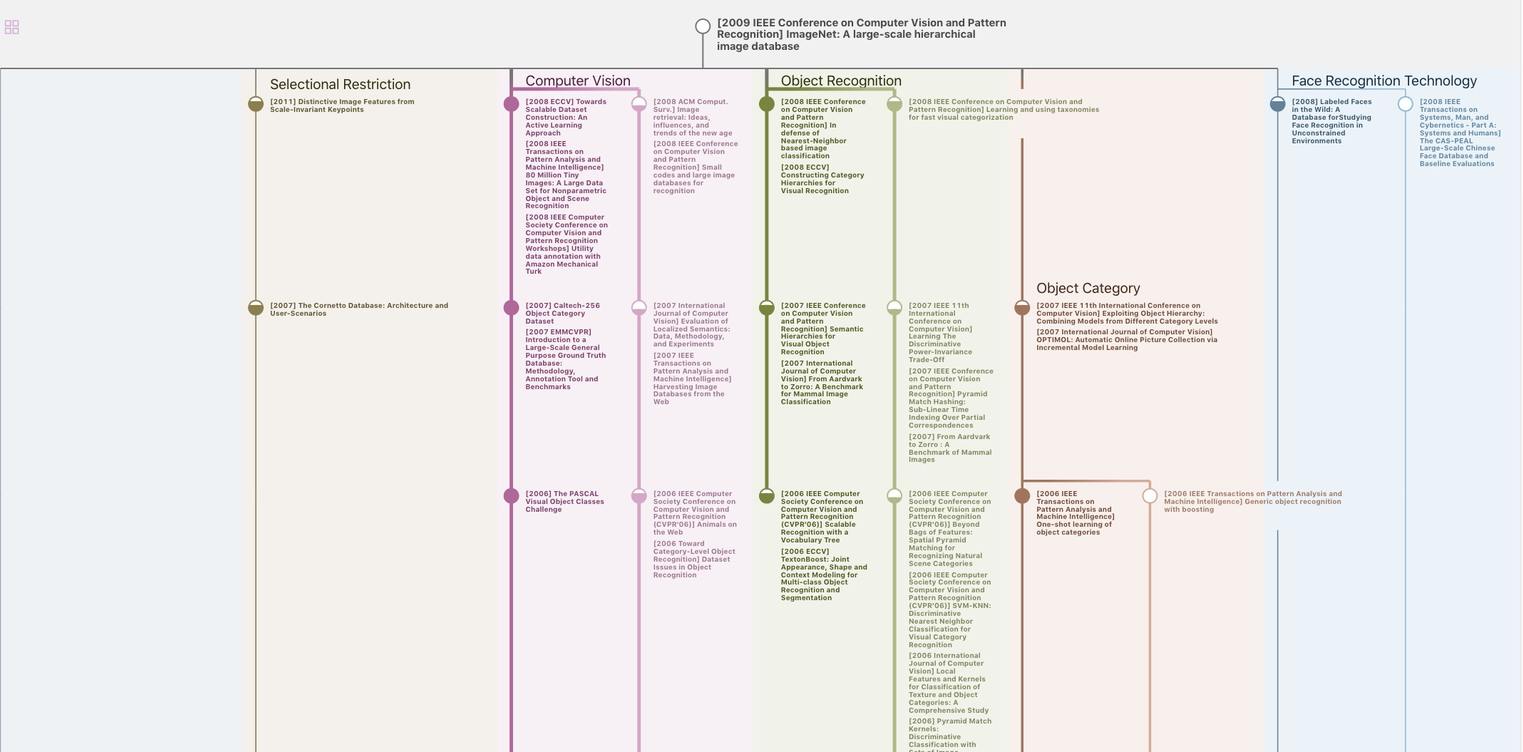
生成溯源树,研究论文发展脉络
Chat Paper
正在生成论文摘要