SLGPT: Using Transfer Learning to Directly Generate Simulink Model Files and Find Bugs in the Simulink Toolchain
PROCEEDINGS OF EVALUATION AND ASSESSMENT IN SOFTWARE ENGINEERING (EASE 2021)(2021)
摘要
Finding bugs in a commercial cyber-physical system (CPS) development tool such as Simulink is hard as its codebase contains millions of lines of code and complete formal language specifications are not available. While deep learning techniques promise to learn such language specifications from sample models, deep learning needs a large number of training data to work well. SLGPT addresses this problem by using transfer learning to leverage the powerful Generative Pre-trained Transformer 2 (GPT-2) model, which has been pre-trained on a large set of training data. SLGPT adapts GPT-2 to Simulink with both randomly generated models and models mined from open-source repositories. SLGPT produced Simulink models that are both more similar to open-source models than its closest competitor, DeepFuzzSL, and found a super-set of the Simulink development toolchain bugs found by DeepFuzzSL.
更多查看译文
关键词
Cyber-physical system development, Simulink, tool chain bugs, deep learning, programming language modeling, GPT-2
AI 理解论文
溯源树
样例
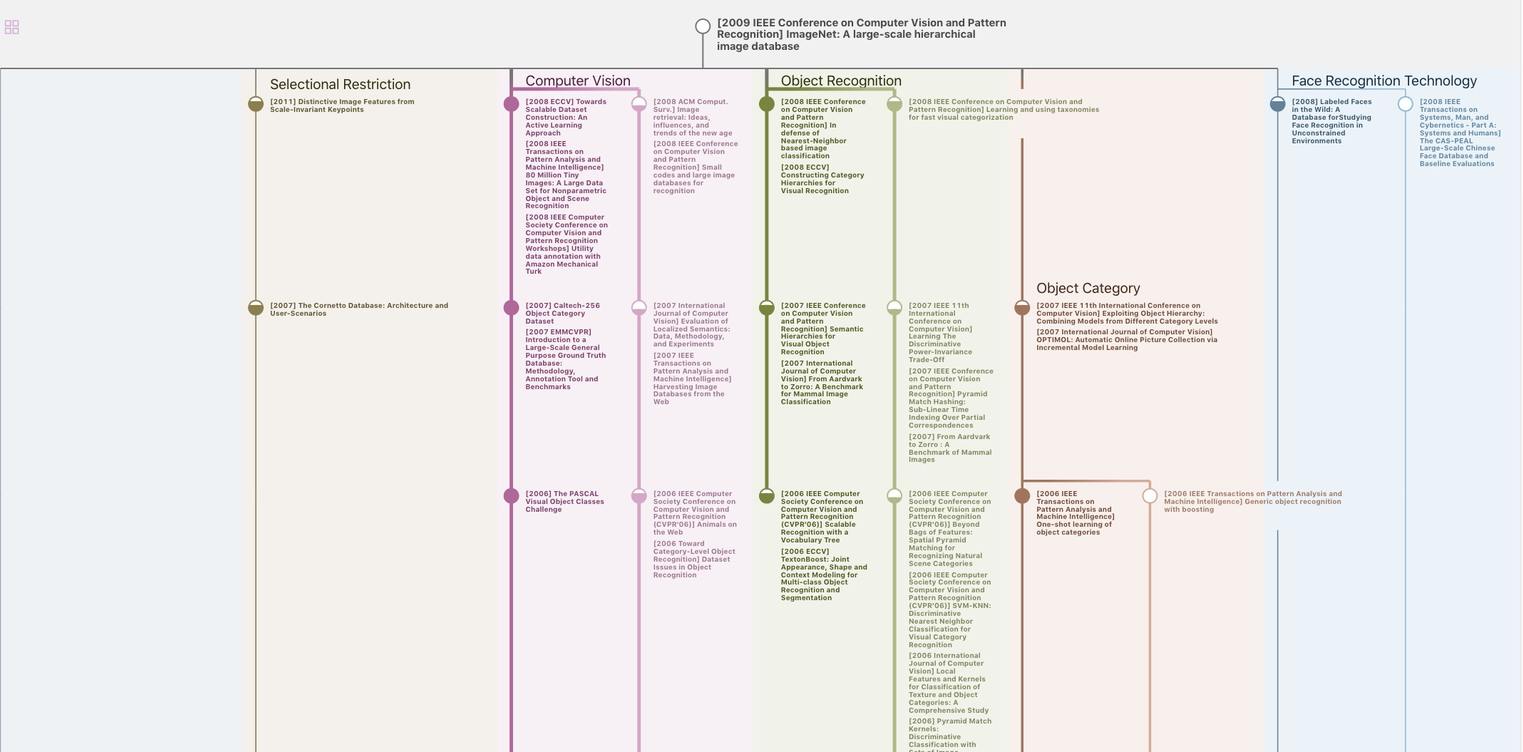
生成溯源树,研究论文发展脉络
Chat Paper
正在生成论文摘要