Smart PAC-learners
Theoretical Computer Science(2011)
摘要
The PAC-learning model is distribution-independent in the sense that the learner must reach a learning goal with a limited number of labeled random examples without any prior knowledge of the underlying domain distribution. In order to achieve this, one needs generalization error bounds that are valid uniformly for every domain distribution. These bounds are (almost) tight in the sense that there is a domain distribution which does not admit a generalization error being significantly smaller than the general bound. Note however that this leaves open the possibility to achieve the learning goal faster if the underlying distribution is “simple”. Informally speaking, we say a PAC-learner L is “smart” if, for a “vast majority” of domain distributions D, L does not require significantly more examples to reach the “learning goal” than the best learner whose strategy is specialized to D. In this paper, focusing on sample complexity and ignoring computational issues, we show that smart learners do exist. This implies (at least from an information-theoretical perspective) that full prior knowledge of the domain distribution (or access to a huge collection of unlabeled examples) does (for a vast majority of domain distributions) not significantly reduce the number of labeled examples required to achieve the learning goal.
更多查看译文
关键词
Machine learning,PAC-learning,Smart PAC-learner,Semi-supervised learning,Learning under a fixed distribution,Minimax theorem,Value of unlabeled data
AI 理解论文
溯源树
样例
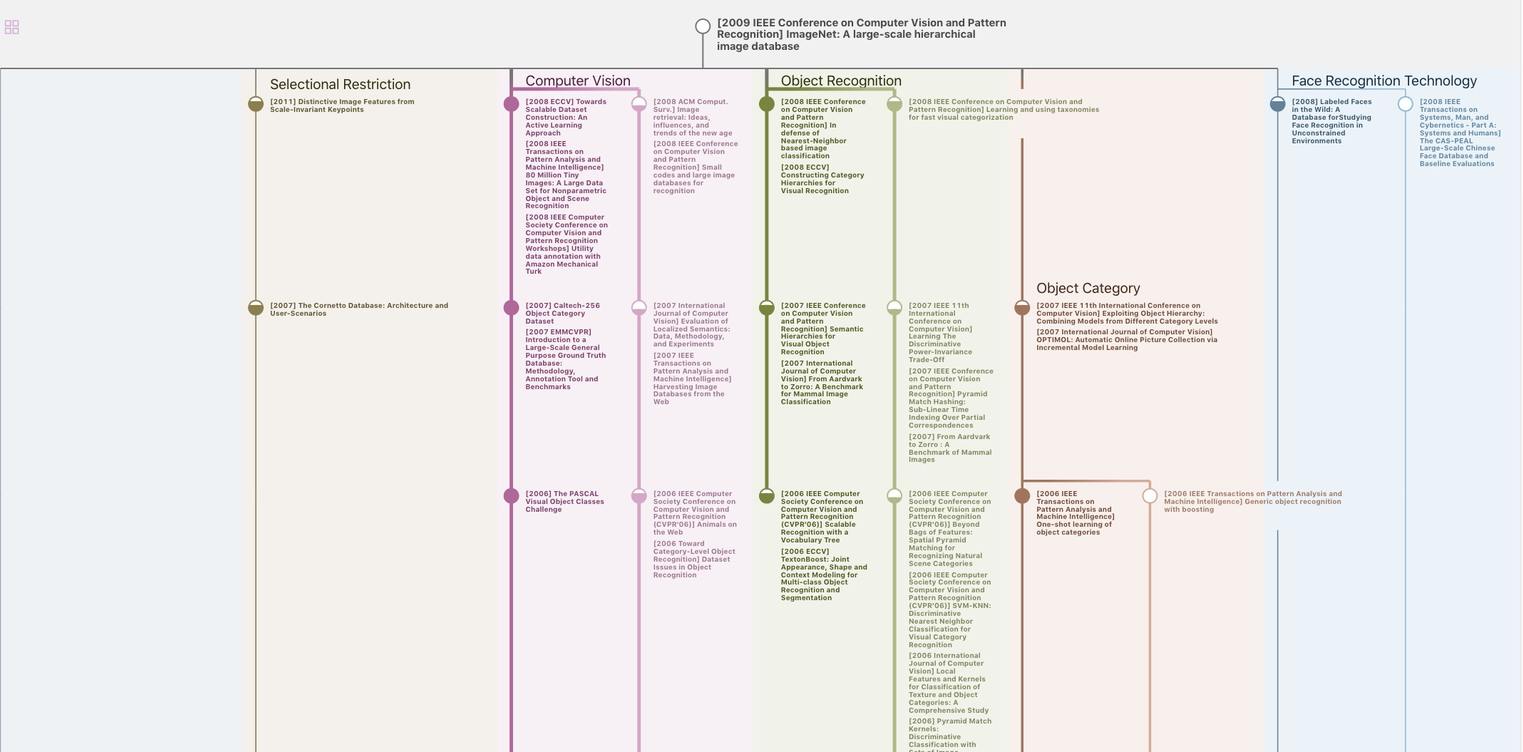
生成溯源树,研究论文发展脉络
Chat Paper
正在生成论文摘要