MetaKernel: Learning Variational Random Features With Limited Labels
IEEE TRANSACTIONS ON PATTERN ANALYSIS AND MACHINE INTELLIGENCE(2024)
摘要
Few-shot learning deals with the fundamental and challenging problem of learning from a few annotated samples, while being able to generalize well on new tasks. The crux of few-shot learning is to extract prior knowledge from related tasks to enable fast adaptation to a new task with a limited amount of data. In this paper, we propose meta-learning kernels with random Fourier features for few-shot learning, we call MetaKernel. Specifically, we propose learning variational random features in a data-driven manner to obtain task-specific kernels by leveraging the shared knowledge provided by related tasks in a meta-learning setting. We treat the random feature basis as the latent variable, which is estimated by variational inference. The shared knowledge from related tasks is incorporated into a context inference of the posterior, which we achieve via a long-short term memory module. To establish more expressive kernels, we deploy conditional normalizing flows based on coupling layers to achieve a richer posterior distribution over random Fourier bases. The resultant kernels are more informative and discriminative, which further improves the few-shot learning. To evaluate our method, we conduct extensive experiments on both few-shot image classification and regression tasks. A thorough ablation study demonstrates that the effectiveness of each introduced component in our method. The benchmark results on fourteen datasets demonstrate MetaKernel consistently delivers at least comparable and often better performance than state-of-the-art alternatives.
更多查看译文
关键词
Task analysis,Kernel,Adaptation models,Prototypes,Optimization,Neural networks,Memory modules,Meta learning,few-shot learning,normalizing flow,variational inference,random features
AI 理解论文
溯源树
样例
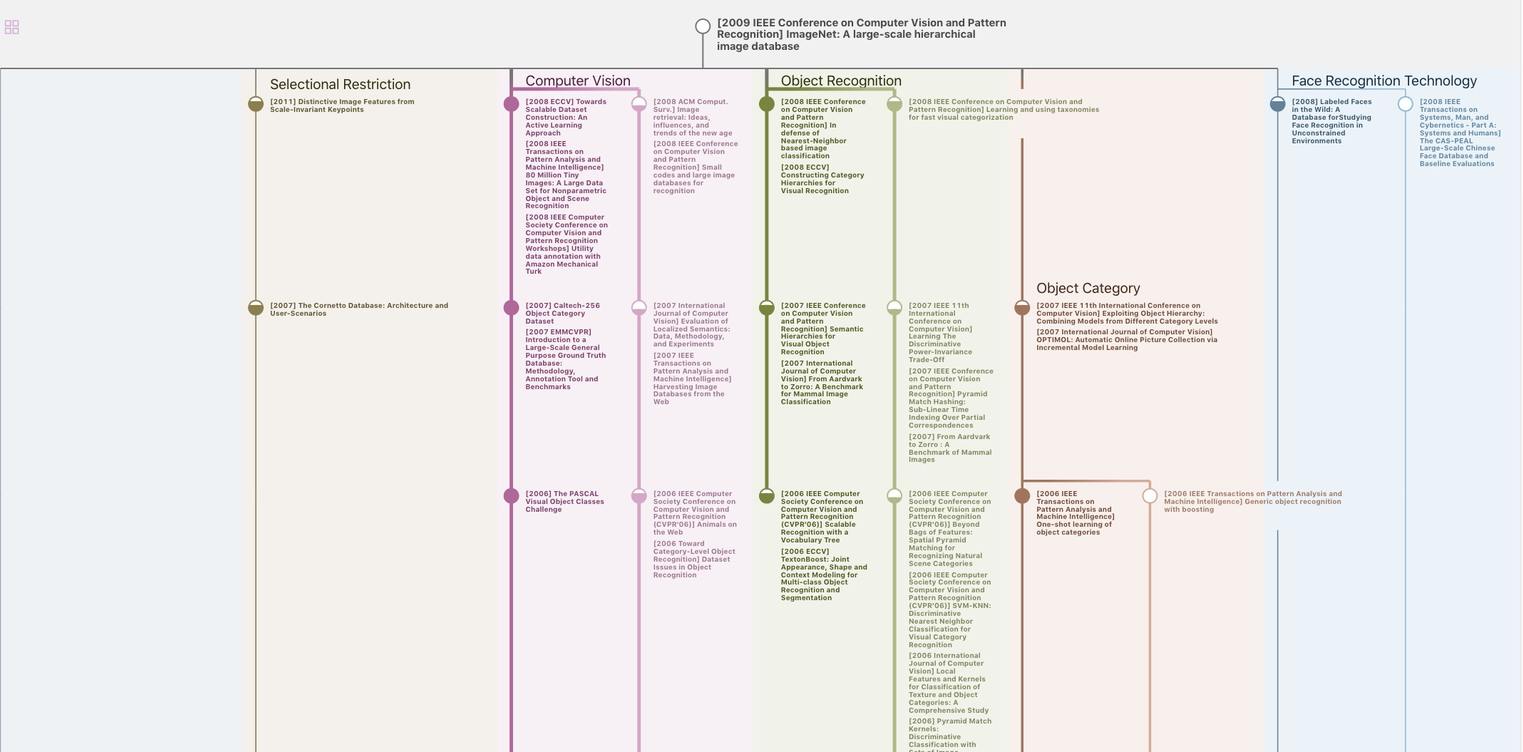
生成溯源树,研究论文发展脉络
Chat Paper
正在生成论文摘要