AdaEmb-Encoder - Adaptive Embedding Spatial Encoder-Based Deduplication for Backing Up Classifier Training Data.
2020 IEEE 39th International Performance Computing and Communications Conference (IPCCC)(2020)
摘要
The advent of the AI era has made it increasingly important to have an efficient backup system to protect training data from loss. Furthermore, a backup of the training data makes it possible to update or retrain the learned model as more data are collected. However, a huge backup overhead will result if a complete copy of all daily collected training data is always made to backup storage, especially because the data typically contain highly redundant information that makes no contribution to model learning. Deduplication is a common technique in modern backup systems to reduce data redundancy. However, existing deduplication methods are invalid for training data. Hence, this paper proposes a novel deduplication strategy for the training data used for learning in a deep neural network classifier. Experimental results showed that the proposed deduplication strategy achieved 93% backup storage space reduction with only 1.3% loss of classification accuracy.
更多查看译文
关键词
Training,Image recognition,Neural networks,Redundancy,Training data,Data models,Task analysis
AI 理解论文
溯源树
样例
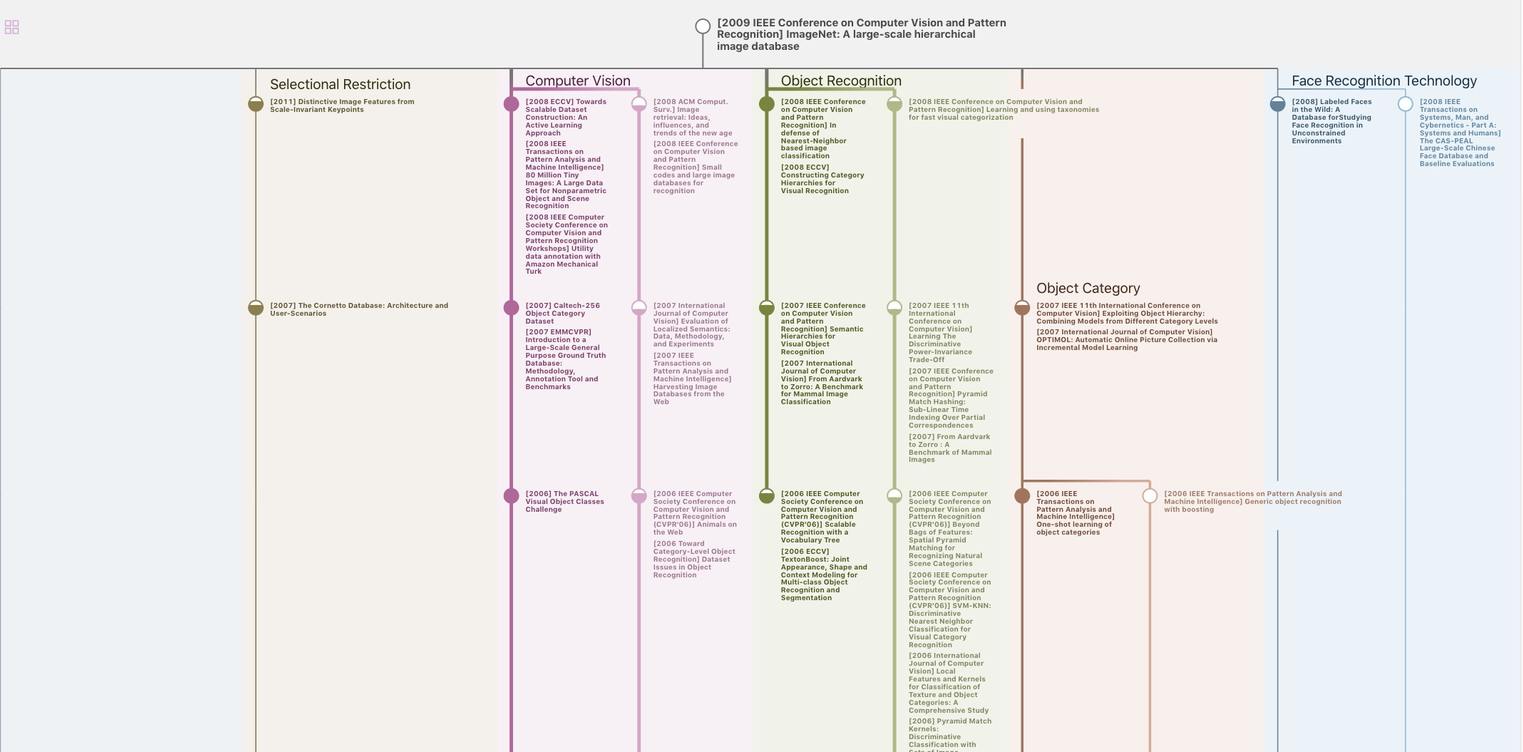
生成溯源树,研究论文发展脉络
Chat Paper
正在生成论文摘要