DyGCN: Efficient Dynamic Graph Embedding With Graph Convolutional Network.
IEEE transactions on neural networks and learning systems(2024)
摘要
Graph embedding, aiming to learn low-dimensional representations (aka. embeddings) of nodes in graphs, has received significant attention. In recent years, there has been a surge of efforts, among which graph convolutional networks (GCNs) have emerged as an effective class of models. However, these methods mainly focus on the static graph embedding. In the present work, an efficient dynamic graph embedding approach is proposed, called dynamic GCN (DyGCN), which is an extension of the GCN-based methods. The embedding propagation scheme of GCN is naturally generalized to a dynamic setting in an efficient manner, which propagates the change in topological structure and neighborhood embeddings along the graph to update the node embeddings. The most affected nodes are updated first, and then their changes are propagated to further nodes, which in turn are updated. Extensive experiments on various dynamic graphs showed that the proposed model can update the node embeddings in a time-saving and performance-preserving way.
更多查看译文
AI 理解论文
溯源树
样例
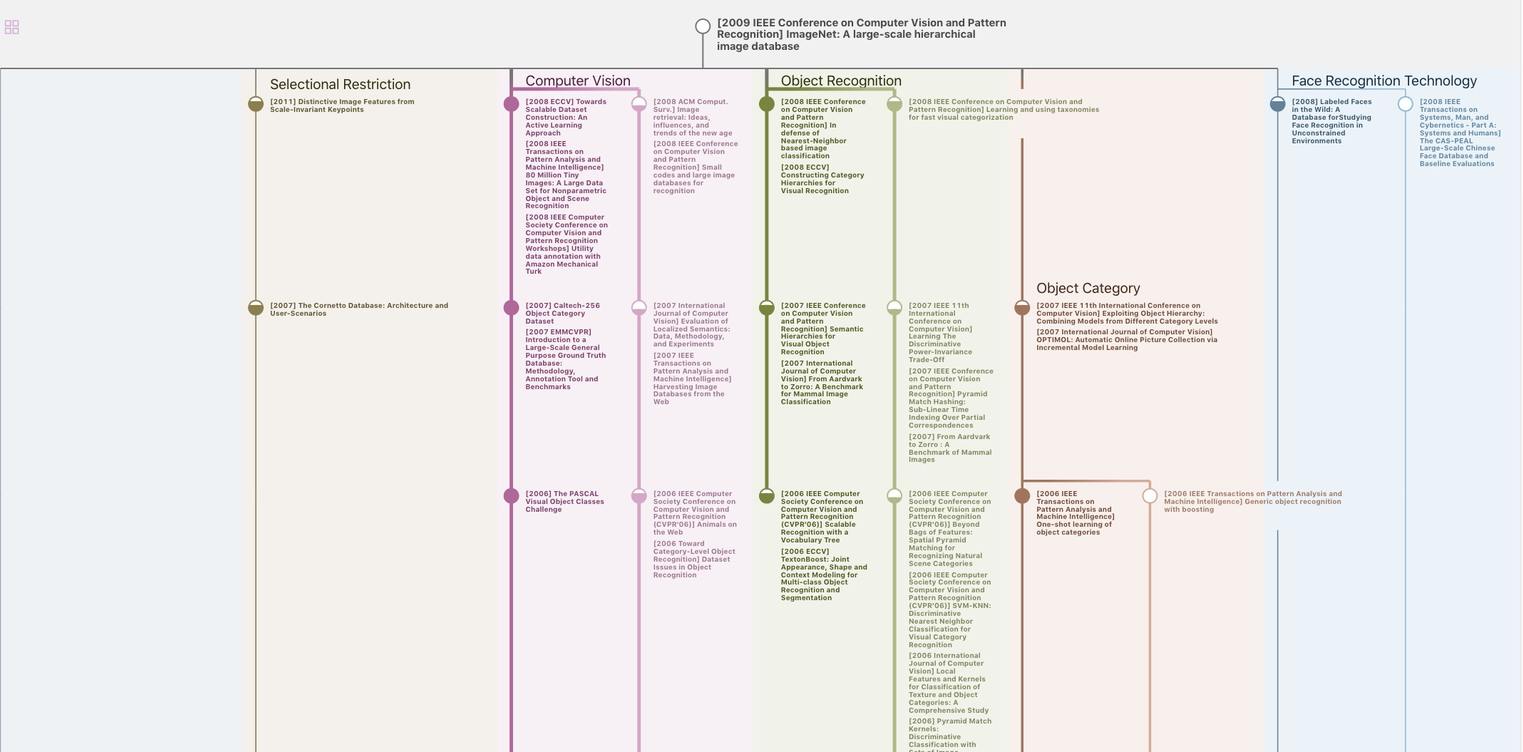
生成溯源树,研究论文发展脉络
Chat Paper
正在生成论文摘要