Video Question-Answering Techniques, Benchmark Datasets And Evaluation Metrics Leveraging Video Captioning: A Comprehensive Survey
IEEE ACCESS(2021)
摘要
While describing visual data is a trivial task for humans, it is an intricate task for a computer. This is even more challenging if the visual data is a video. Comprehending a video and describing it is called Video Captioning. This involves understanding the semantics of a video and then generating human-like descriptions of the video. It requires the collaboration of both research communities of computer vision and natural language processing. The captions generated by video captioning can be further utilized for video retrieval, summarization, question-answering, etc. Video Question-Answering (video-QA) involves querying the system to obtain an answer in response. This paper presents a brief survey of the video captioning techniques and a comprehensive review of existing techniques, datasets, and evaluation metrics for the task of video-QA. Video-QA techniques rely on the attention mechanism to generate relevant results. The presented survey shows that recent works on Memory Networks, Generative Adversarial Networks, and Reinforced Decoders, have the capability to handle the complexities and challenges of video-QA. Additionally, the graph-based methods, although less explored, give very promising results. In this article, we have discussed the emerging research directions and various application areas of video-QA.
更多查看译文
关键词
Visualization, Annotations, Task analysis, Knowledge discovery, Feature extraction, Semantics, Ontologies, Video question answering, video captioning, video description generation, natural language processing, deep learning, computer vision, LSTM, CNN, attention model, memory network
AI 理解论文
溯源树
样例
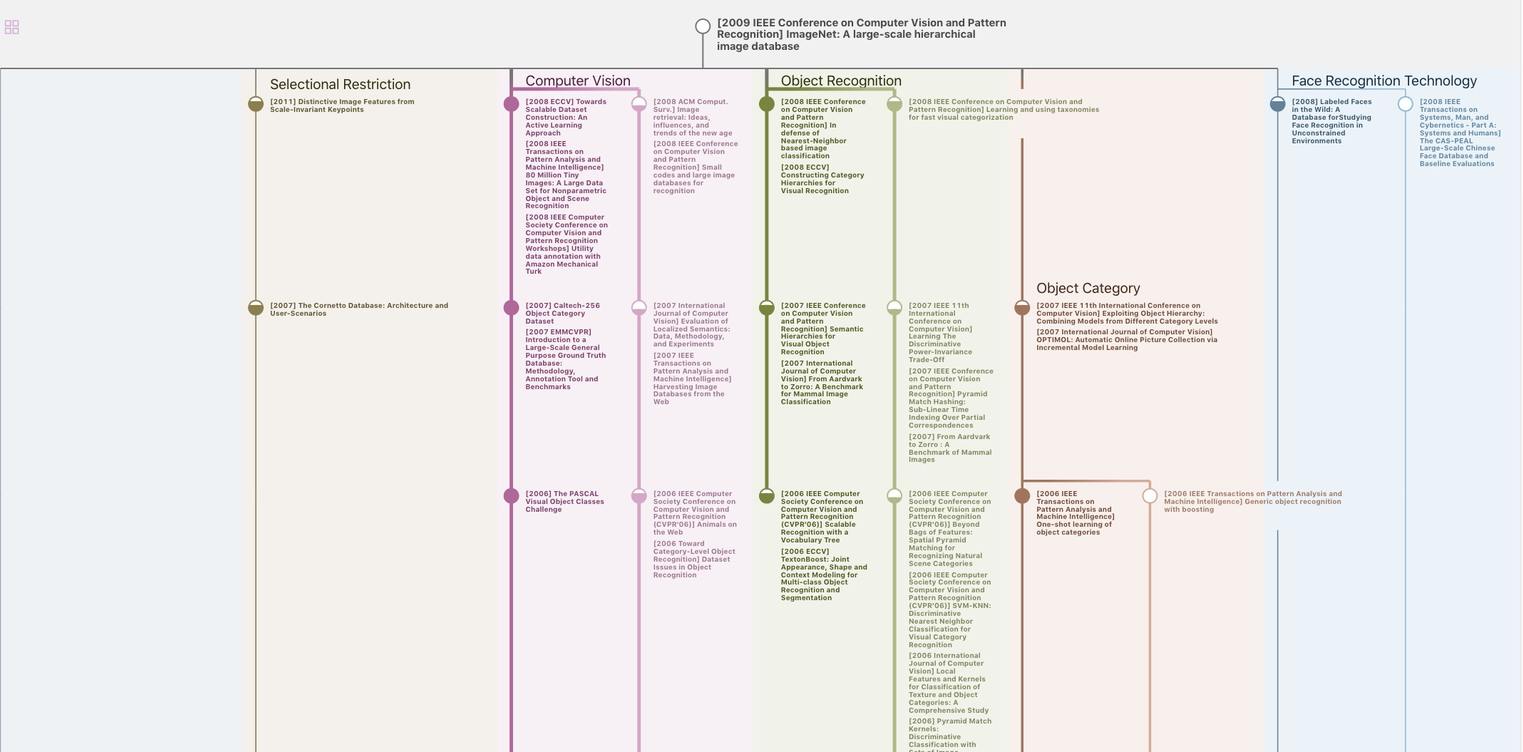
生成溯源树,研究论文发展脉络
Chat Paper
正在生成论文摘要