Private Cross-Silo Federated Learning for Extracting Vaccine Adverse Event Mentions
MACHINE LEARNING AND PRINCIPLES AND PRACTICE OF KNOWLEDGE DISCOVERY IN DATABASES, PT II(2021)
摘要
Federated Learning (FL) is quickly becoming a goto distributed training paradigm for users to jointly train a global model without physically sharing their data. Users can indirectly contribute to, and directly benefit from a much larger aggregate data corpus used to train the global model. However, literature on successful application of FL in real-world problem settings is somewhat sparse. In this paper, we describe our experience applying a FL based solution to the Named Entity Recognition (NER) task for an adverse event detection application in the context of mass scale vaccination programs. We present a comprehensive empirical analysis of various dimensions of benefits gained with FL based training. Furthermore, we investigate effects of tighter Differential Privacy (DP) constraints in highly sensitive settings where federation users must enforce DP to ensure strict privacy guarantees. We show that DP can severely cripple the global model's prediction accuracy, thus disincentivizing users from participating in the federation. In response, we demonstrate how recent innovation in personalization methods can help significantly recover the lost accuracy.
更多查看译文
关键词
federated learning,vaccine,cross-silo
AI 理解论文
溯源树
样例
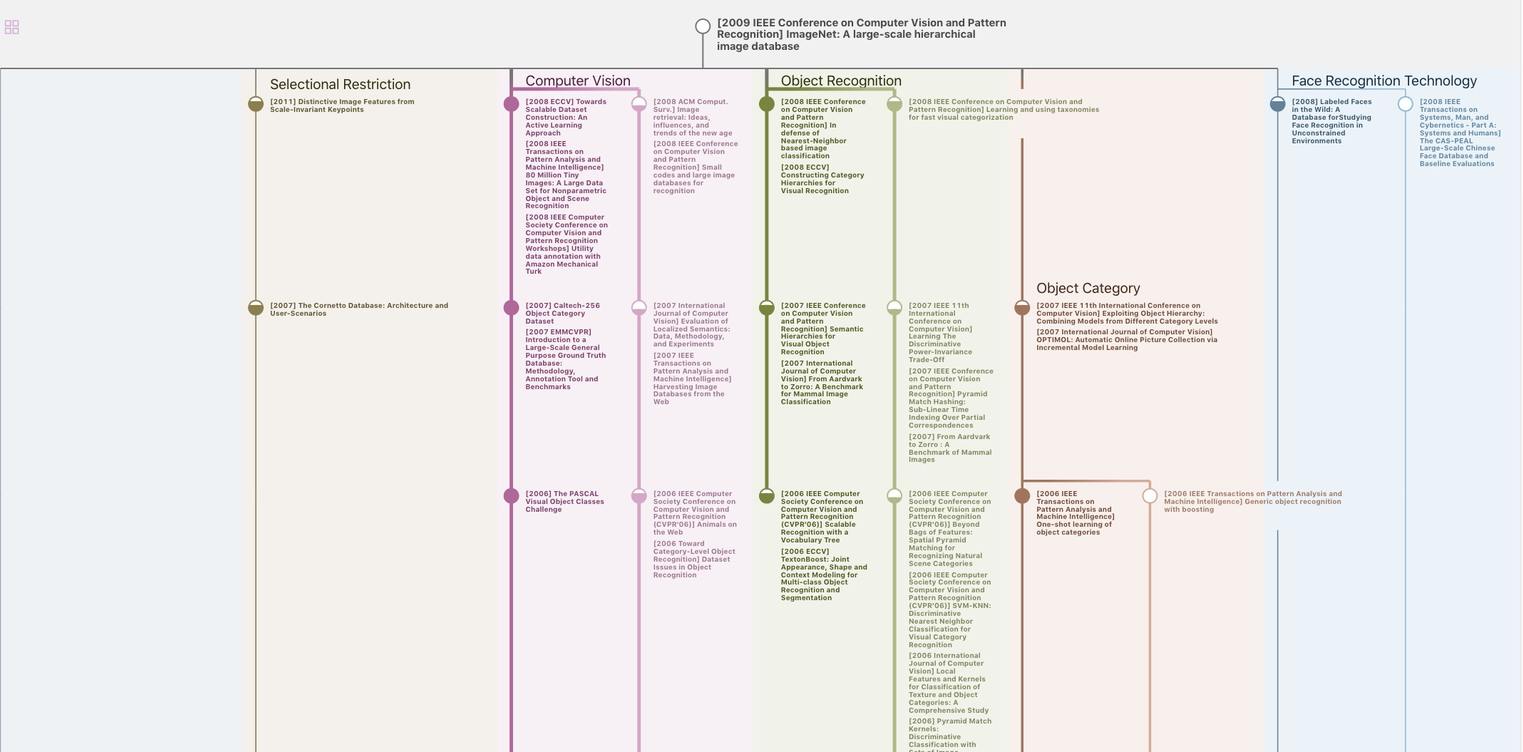
生成溯源树,研究论文发展脉络
Chat Paper
正在生成论文摘要