SYSTEMS AND METHODS FOR ROBUST LARGE-SCALE MACHINE LEARNING
user-5bd69975530c70d56f390249(2018)
摘要
The present disclosure provides a new scalable coordinate descent (SCD) algorithm and associated system for generalized linear models whose convergence behavior is always the same, regardless of how much SCD is scaled out and regardless of the computing environment. This makes SCD highly robust and enables it to scale to massive datasets on low-cost commodity servers. According to one aspect, by using a natural partitioning of parameters into blocks, updates can be performed in parallel a block at a time without compromising convergence. Experimental results on a real advertising dataset are used to demonstrate SCD's cost effectiveness and scalability.
更多查看译文
关键词
Coordinate descent,Cost effectiveness,Scalability,Server,Convergence (routing),Distributed computing,Computer science,Generalized linear model
AI 理解论文
溯源树
样例
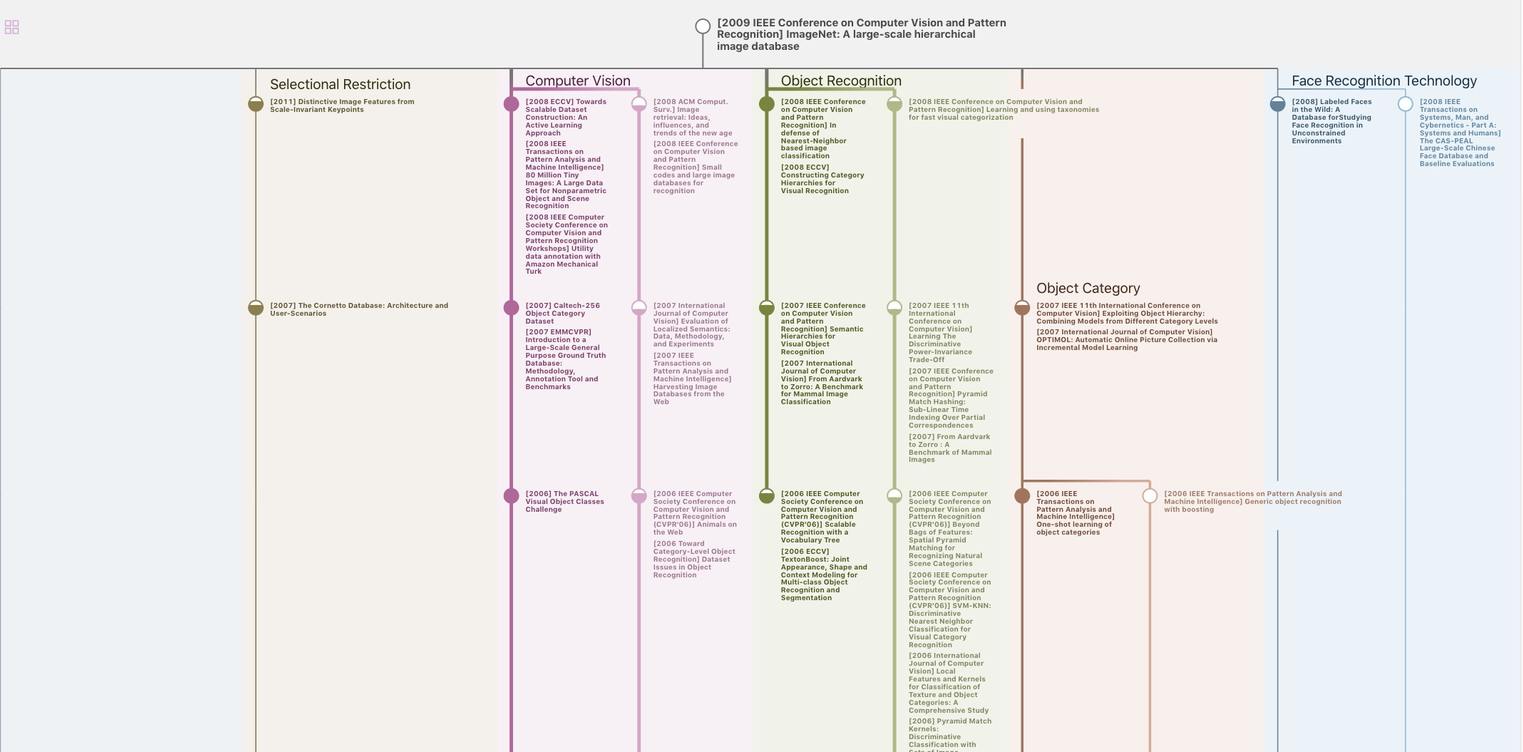
生成溯源树,研究论文发展脉络
Chat Paper
正在生成论文摘要