Part-Level Car Parsing and Reconstruction in Single Street View Images
IEEE Transactions on Pattern Analysis and Machine Intelligence(2022)
摘要
Part information has been proven to be resistant to occlusions and viewpoint changes, which are main difficulties in car parsing and reconstruction. However, in the absence of datasets and approaches incorporating car parts, there are limited works that benefit from it. In this paper, we propose the first part-aware approach for joint part-level car parsing and reconstruction in single street view images. Without labor-intensive part annotations on real images, our approach simultaneously estimates pose, shape, and semantic parts of cars. There are two contributions in this paper. First, our network introduces dense part information to facilitate pose and shape estimation, which is further optimized with a novel 3D loss. To obtain part information in real images, a class-consistent method is introduced to implicitly transfer part knowledge from synthesized images. Second, we construct the first high-quality dataset containing 348 car models with physical dimensions and part annotations. Given these models, 60K synthesized images with randomized configurations are generated. Experimental results demonstrate that part knowledge can be effectively transferred with our class-consistent method, which significantly improves part segmentation performance on real street views. By fusing dense part information, our pose and shape estimation results achieve the state-of-the-art performance on the ApolloCar3D and outperform previous approaches by large margins in terms of both A3DP-Abs and A3DP-Rel.
更多查看译文
关键词
Algorithms,Automobiles
AI 理解论文
溯源树
样例
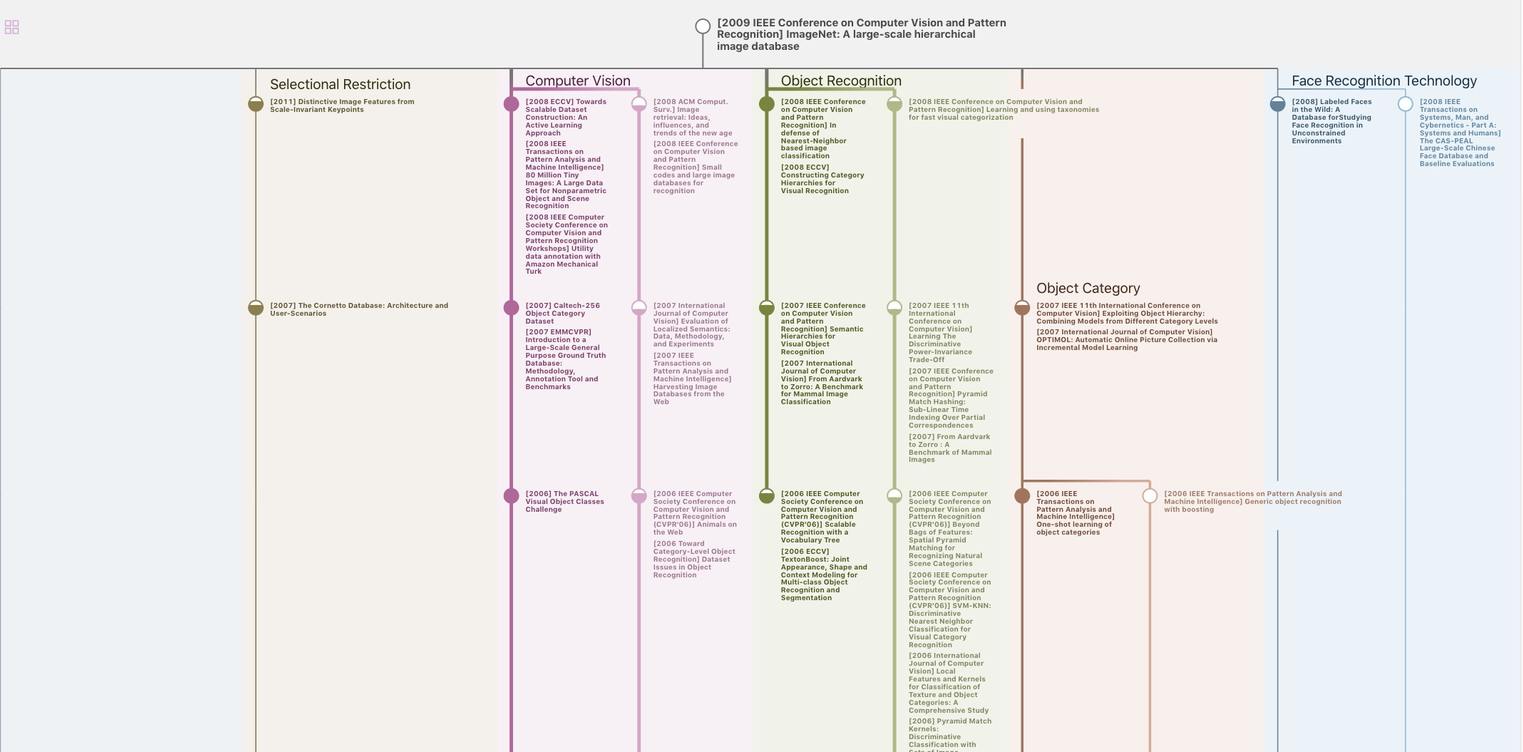
生成溯源树,研究论文发展脉络
Chat Paper
正在生成论文摘要