LEARNING TO ESTIMATE KERNEL SCALE AND ORIENTATION OF DEFOCUS BLUR WITH ASYMMETRIC CODED APERTURE
2021 IEEE INTERNATIONAL CONFERENCE ON ACOUSTICS, SPEECH AND SIGNAL PROCESSING (ICASSP 2021)(2021)
摘要
Consistent in-focus input imagery is an essential precondition for machine vision systems to perceive the dynamic environment. A defocus blur severely degrades the performance of vision systems. To tackle this problem, we propose a deep-learning-based framework estimating the kernel scale and orientation of the defocus blur to adjust lens focus rapidly. Our pipeline utilizes 3D ConvNet for a variable number of input hypotheses to select the optimal slice from the input stack. We use random shuffle and Gumbel-softmax to improve network performance. We also propose to generate synthetic defocused images with various asymmetric coded apertures to facilitate training. Experiments are conducted to demonstrate the effectiveness of our framework.
更多查看译文
关键词
Blur kernel estimation, focus adjustment, asymmetric coded aperture
AI 理解论文
溯源树
样例
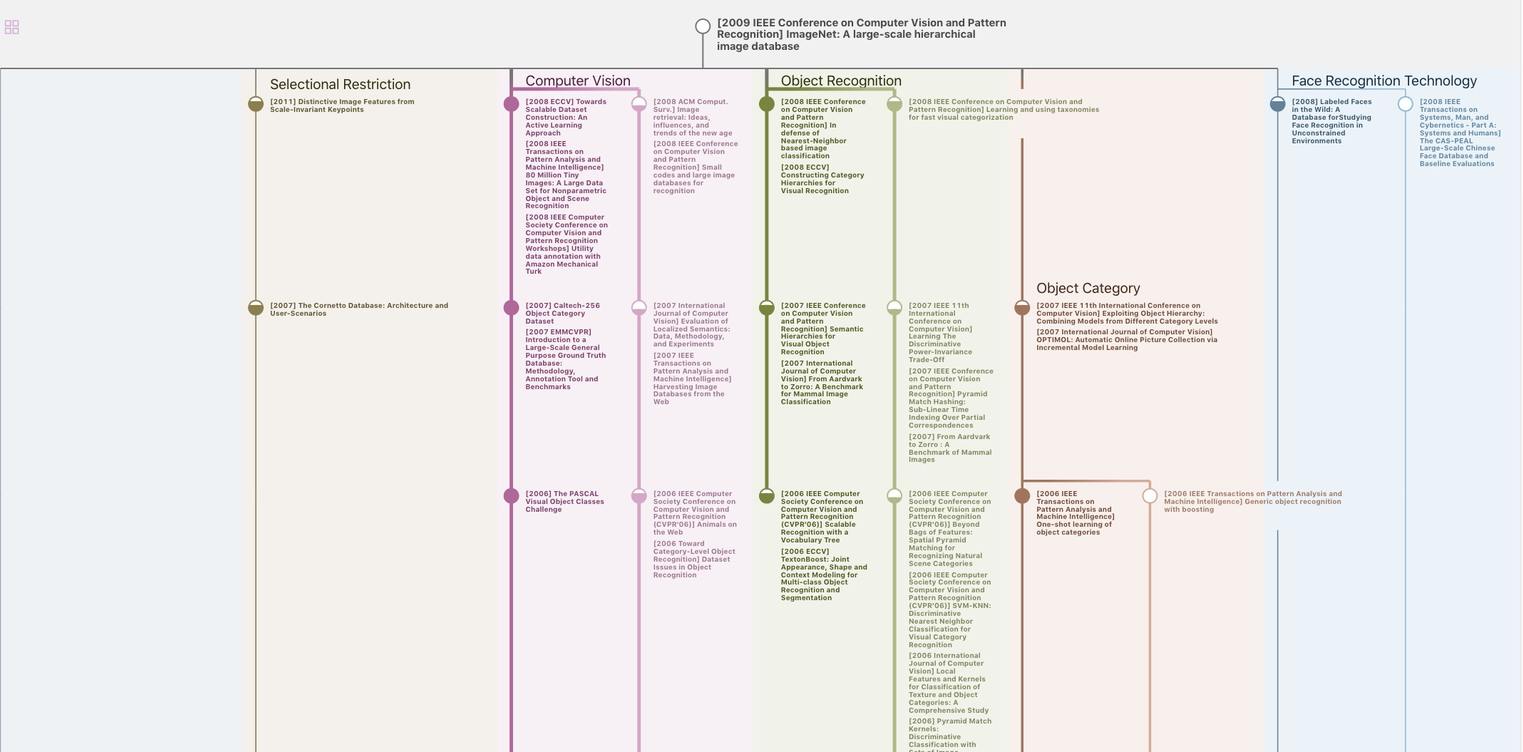
生成溯源树,研究论文发展脉络
Chat Paper
正在生成论文摘要