Serverless Data Science - Are We There Yet? A Case Study of Model Serving
PROCEEDINGS OF THE 2022 INTERNATIONAL CONFERENCE ON MANAGEMENT OF DATA (SIGMOD '22)(2022)
摘要
Machine learning (ML) is an important part of modern data science applications. Data scientists today have to manage the end-to-end ML life cycle that includes both model training and model serving, the latter of which is essential, as it makes their works available to end-users. Systems of model serving require high performance, low cost, and ease of management. Cloud providers are already offering model serving choices, including managed services and self-rented servers. Recently, serverless computing, whose advantages include high elasticity and a fine-grained cost model, brings another option for model serving. Our goal in this paper is to examine the viability of serverless as a mainstream model serving platform. To this end, we first conduct a comprehensive evaluation of the performance and cost of serverless against other model serving systems on Amazon Web Service and Google Cloud Platform. We find that serverless outperforms many cloud-based alternatives. Further, there are settings under which it even achieves better performance than GPU-based systems. Next, we present the design space of serverless model serving, which comprises multiple dimensions, including cloud platforms, serving runtimes, and other function-specific parameters. For each dimension, we analyze the impact of different choices and provide suggestions for data scientists to better utilize serverless model serving. Finally, we discuss challenges and opportunities in building a more practical serverless model serving system.
更多查看译文
关键词
data science, serverless computing, model serving, design space
AI 理解论文
溯源树
样例
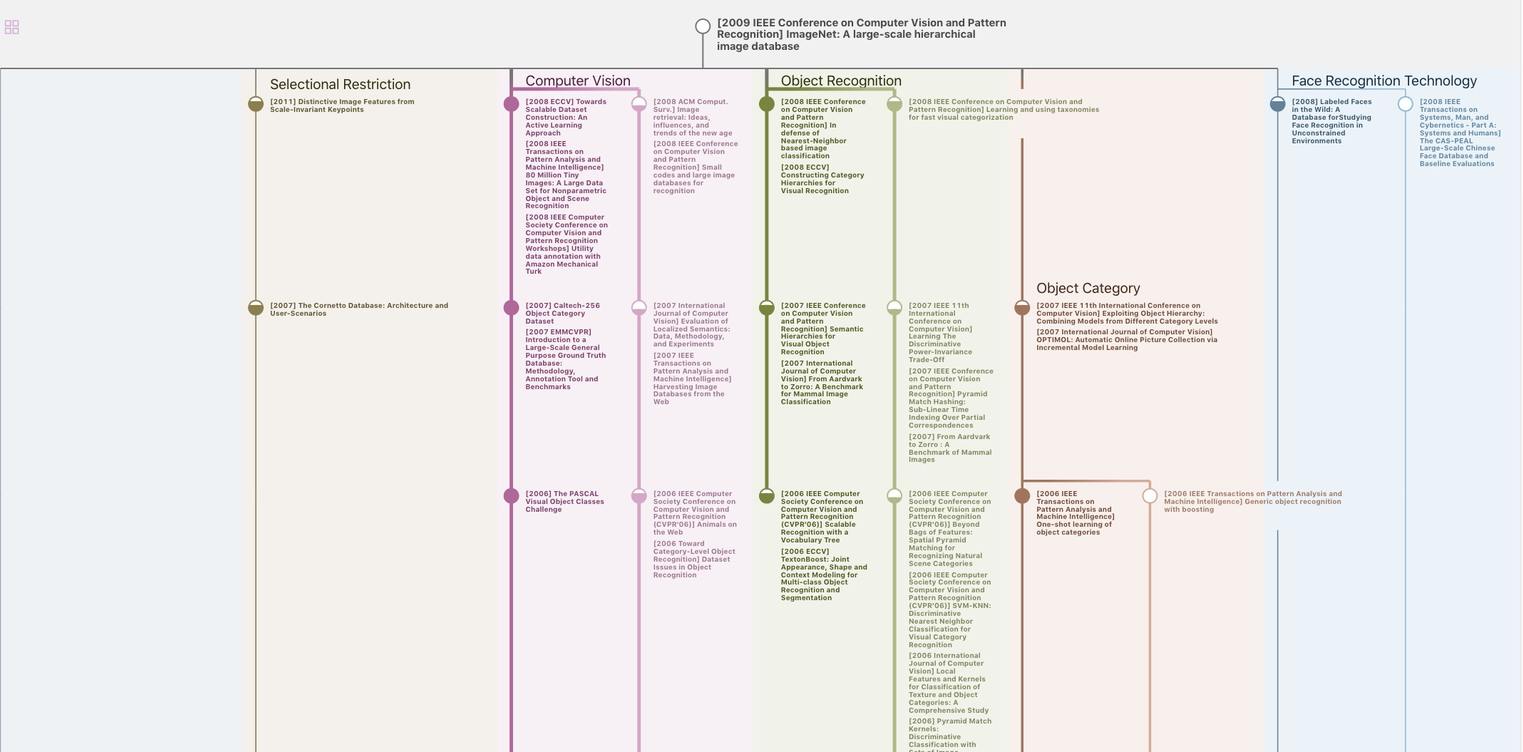
生成溯源树,研究论文发展脉络
Chat Paper
正在生成论文摘要