TRANSMASK: A COMPACT AND FAST SPEECH SEPARATION MODEL BASED ON TRANSFORMER
2021 IEEE INTERNATIONAL CONFERENCE ON ACOUSTICS, SPEECH AND SIGNAL PROCESSING (ICASSP 2021)(2021)
摘要
Speech separation is an important problem in speech processing, which targets to separate and generate clean speech from a mixed audio containing speech from different speakers. Empowered by the deep learning technologies over sequence-to-sequence domain, recent neural speech separation models are now capable of generating highly clean speech audios. To make these models more practical by reducing the model size and inference time while maintaining high separation quality, we propose a new transformer-based speech separation approach, called TransMask. By fully unleashing the power of self-attention on long-term dependency reception, we demonstrate the size of TransMask is more than 60% smaller and the inference is more than 2 times faster than state-of-the-art solutions. TransMask fully utilizes the parallelism during inference, and achieves nearly linear inference time within reasonable input audio lengths. It also outperforms existing solutions on output speech audio quality, achieving SDR above 16 over Librimix benchmark.
更多查看译文
关键词
speech separation, transformer, deep learning
AI 理解论文
溯源树
样例
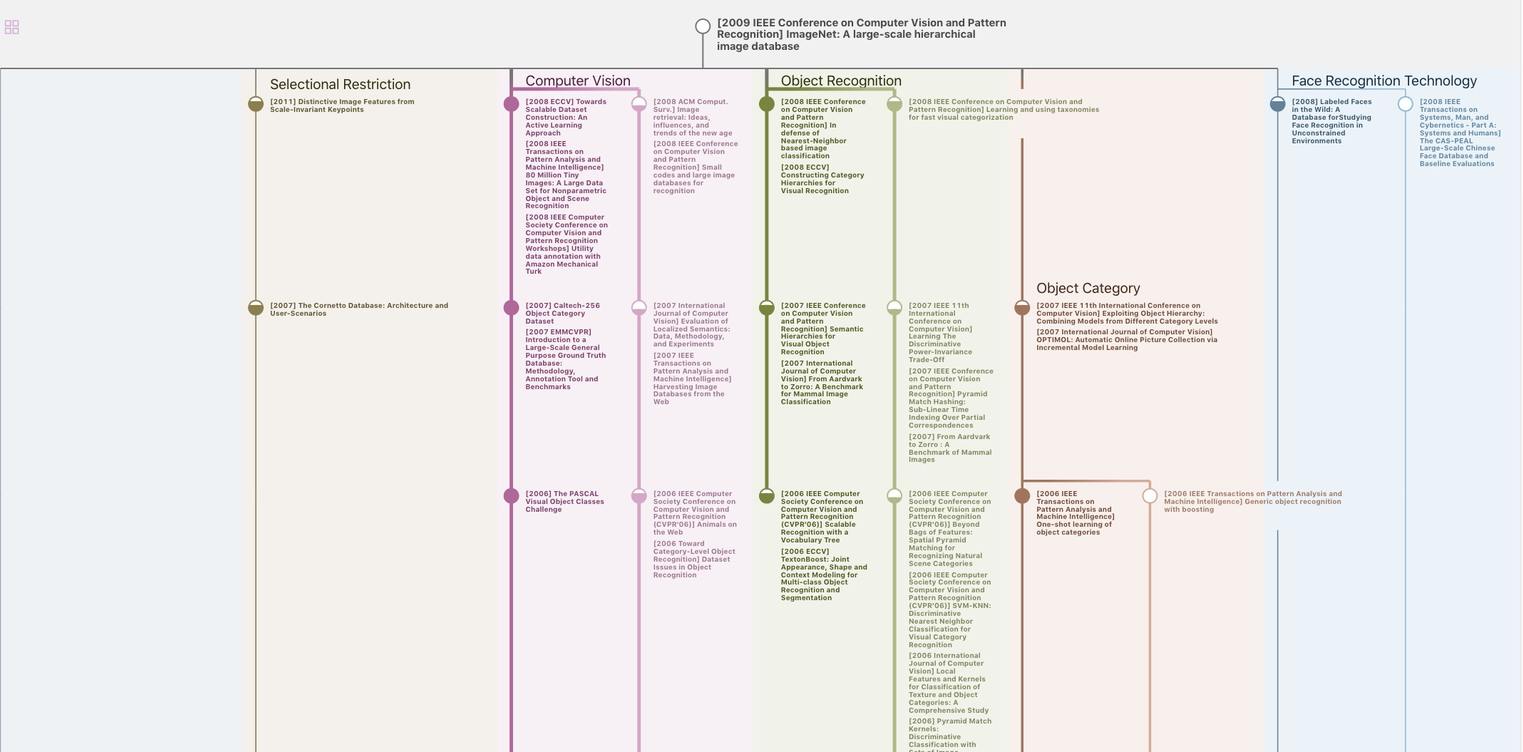
生成溯源树,研究论文发展脉络
Chat Paper
正在生成论文摘要