Parameter-Free Locally Accelerated Conditional Gradients
INTERNATIONAL CONFERENCE ON MACHINE LEARNING, VOL 139(2021)
摘要
Projection-free conditional gradient (CG) methods are the algorithms of choice for constrained optimization setups in which projections are often computationally prohibitive but linear optimization over the constraint set remains computationally feasible. Unlike in projection-based methods, globally accelerated convergence rates are in general unattainable for CG. However, a very recent work on Locally accelerated CG (LaCG) has demonstrated that local acceleration for CG is possible for many settings of interest. The main downside of LaCG is that it requires knowledge of the smoothness and strong convexity parameters of the objective function. We remove this limitation by introducing a novel, Parameter-Free Locally accelerated CG (PF-LaCG) algorithm, for which we provide rigorous convergence guarantees. Our theoretical results are complemented by numerical experiments, which demonstrate local acceleration and showcase the practical improvements of PF-LaCG over non-accelerated algorithms, both in terms of iteration count and wall-clock time.
更多查看译文
关键词
accelerated conditional gradients,parameter-free
AI 理解论文
溯源树
样例
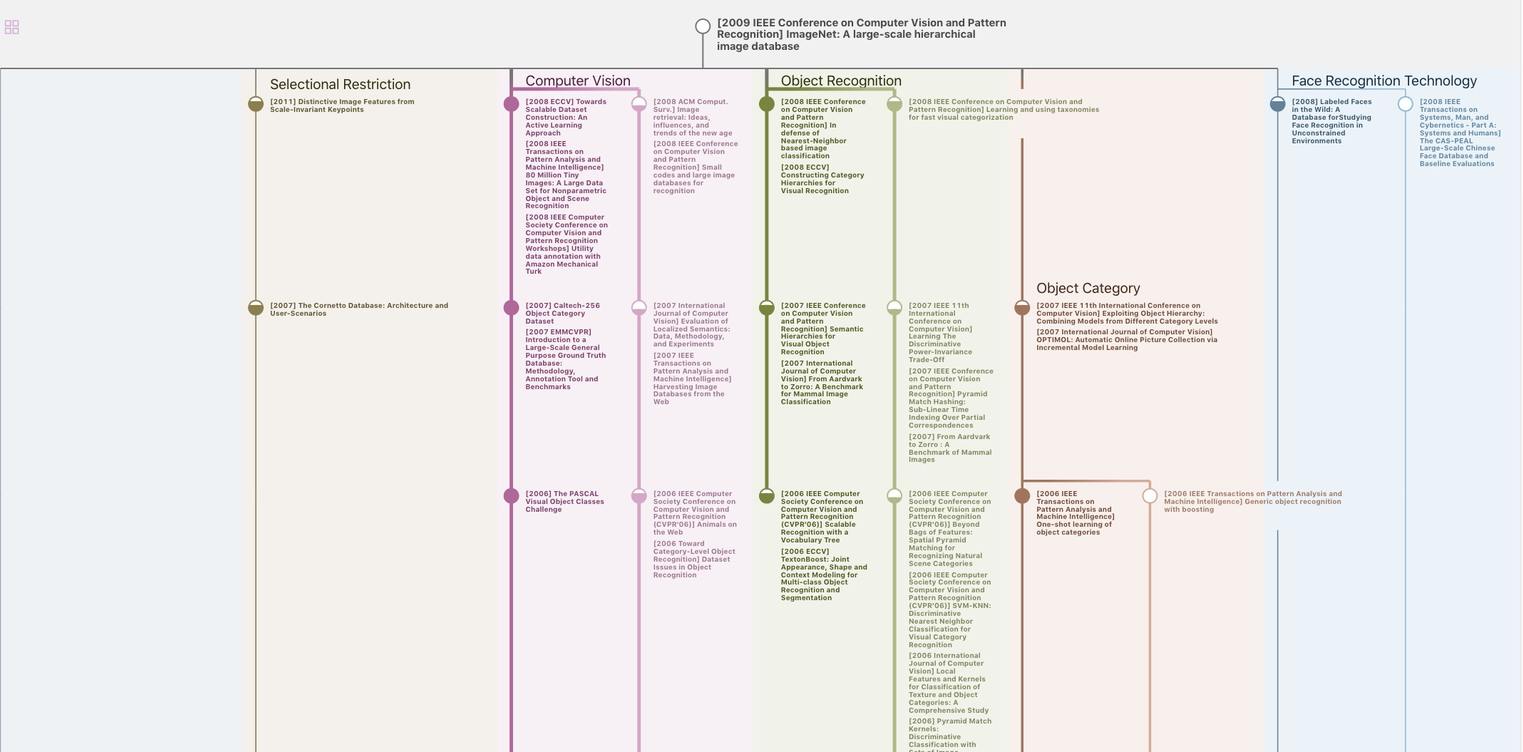
生成溯源树,研究论文发展脉络
Chat Paper
正在生成论文摘要