Infeasibility detection with primal-dual hybrid gradient for large-scale linear programming
SIAM JOURNAL ON OPTIMIZATION(2024)
摘要
We study the problem of detecting infeasibility of large-scale linear programming problems using the primal -dual hybrid gradient (PDHG) method of Chambolle and Pock [J. Math. Imaging Vision, 40 (2011), pp. 120--145]. The literature on PDHG has focused chiefly on problems with at least one optimal solution. We show that when the problem is infeasible or unbounded, the iterates diverge at a controlled rate toward a well-defined ray. In turn, the direction of such a ray recovers infeasibility certificates. Based on this fact, we propose a simple way to extract approximate infeasibility certificates from the iterates of PDHG. We study three sequences that converge to certificates: the difference of iterates, the normalized iterates, and the normalized average. All of them are easy to compute and suitable for large-scale problems. We show that the normalized iterates and normalized averages achieve a convergence rate of O \bigl(k-1\bigr) . This rate is general and applies to any fixed-point iteration of a nonexpansive operator. Thus, it is a result of independent interest that goes well beyond our setting. Finally, we show that, under nondegeneracy assumptions, the iterates of PDHG identify the active set of an auxiliary feasible problem in finite time, which ensures that the difference of iterates exhibits eventual linear convergence. These results provide a theoretical justification for infeasibility detection in the newly developed linear programming solver PDLP.
更多查看译文
关键词
linear programming,infeasibility detection,primal-dual algorithms
AI 理解论文
溯源树
样例
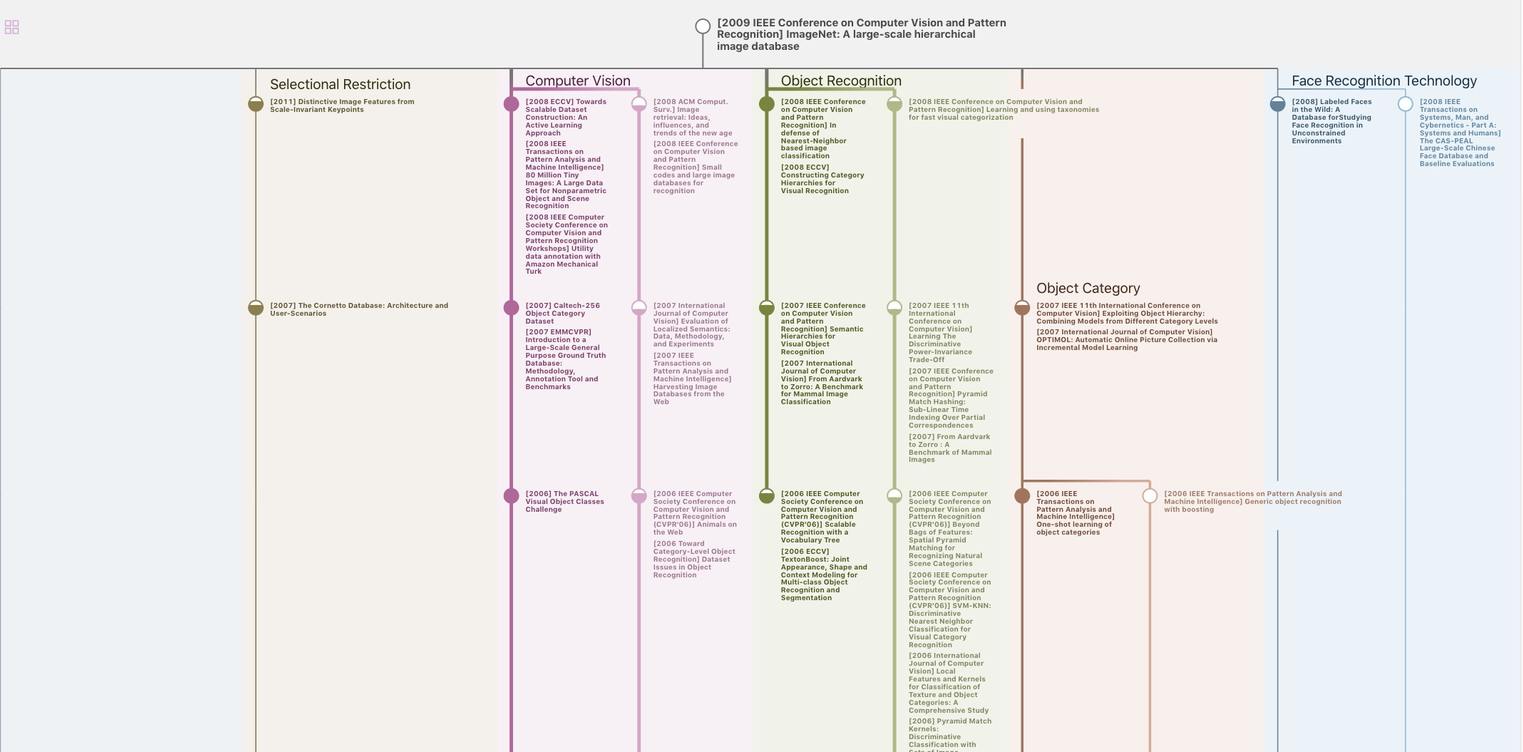
生成溯源树,研究论文发展脉络
Chat Paper
正在生成论文摘要