Deep Learning based Semantic Communications - An Initial Investigation.
GLOBECOM(2020)
摘要
Recently, deep learned enabled end-to-end (E2E) communication systems have been developed to merge all physical layer blocks in the traditional communication systems, which makes joint transceiver optimization possible. Powered by deep learning, natural language processing (NIP) has achieved great success in analyzing and understanding large amounts of language texts. Inspired by research results in both areas, we aim to provide a new view on communication systems from the semantic level. Particularly, we propose a deep learning based semantic communication system, named DeepSC, for text transmission. Based on the Transformer, the DeepSC aims at maximizing the system capacity and minimizing the semantic errors by recovering the meaning of sentences, rather than bit- or symbol-errors in traditional communications. Compared with the traditional communication system without considering semantic information exchange, the proposed DeepSC is more robust to channel variation and can achieve better performance, especially in the low signal-to-noise ratio (SNR) regime, as demonstrated by the extensive simulation results.
更多查看译文
关键词
deep learning,semantic communications,end-to-end communication systems,physical layer blocks,communication system,joint transceiver optimization possible,natural language processing,language texts,semantic level,semantic communication system,DeepSC,system capacity,semantic errors,semantic information exchange
AI 理解论文
溯源树
样例
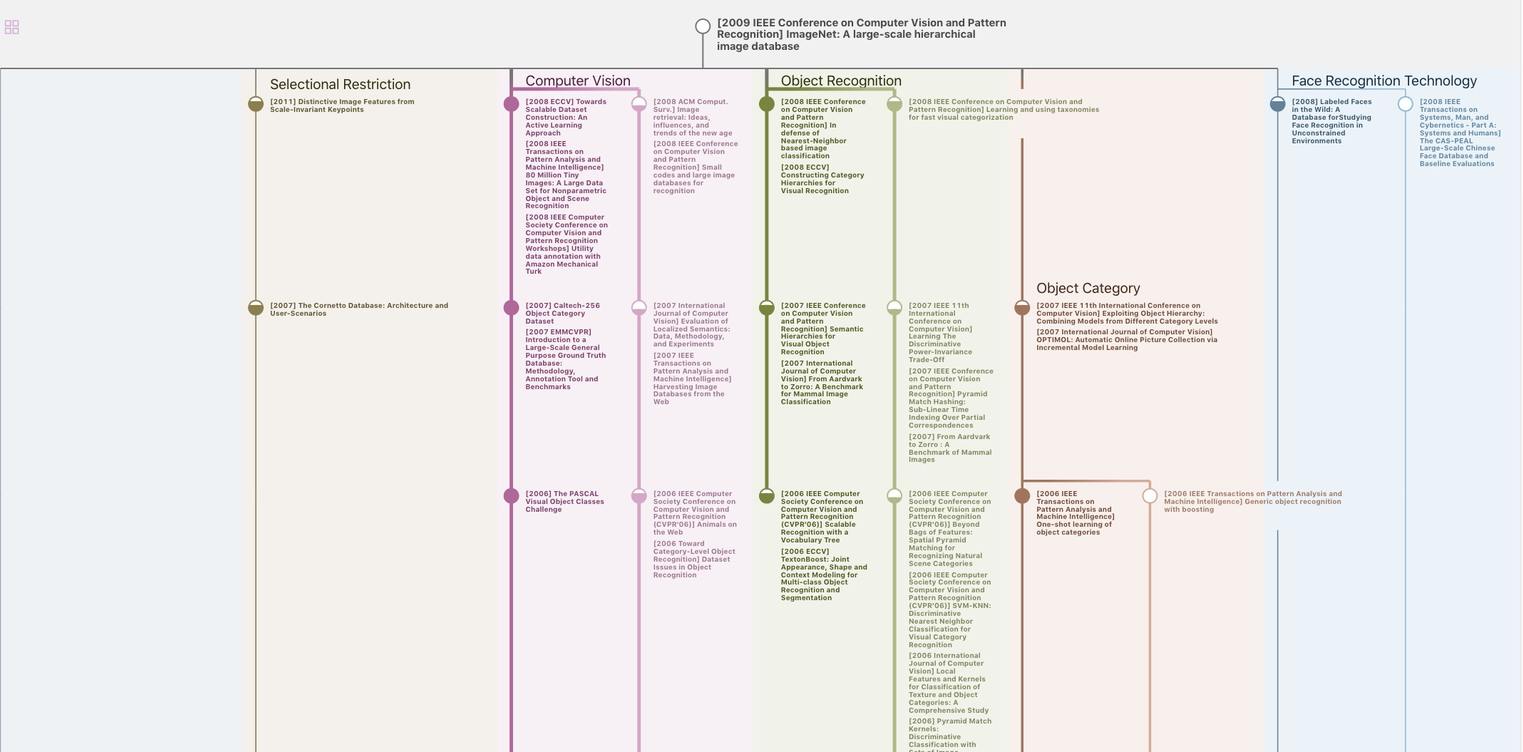
生成溯源树,研究论文发展脉络
Chat Paper
正在生成论文摘要