Explaining Any Time Series Classifier
2020 IEEE Second International Conference on Cognitive Machine Intelligence (CogMI)(2020)
摘要
We present a method to explain the decisions of black box models for time series classification. The explanation consists of factual and counterfactual shapelet-based rules revealing the reasons for the classification, and of a set of exemplars and counter-exemplars highlighting similarities and differences with the time series under analysis. The proposed method first generates exemplar and counter-exemplar time series in the latent feature space and learns a local latent decision tree classifier. Then, it selects and decodes those respecting the decision rules explaining the decision. Finally, it learns on them a shapelet-tree that reveals the parts of the time series that must, and must not, be contained for getting the returned outcome from the black box. A wide experimentation shows that the proposed method provides faithful, meaningful and interpretable explanations.
更多查看译文
关键词
Explainable AI,Time Series Classification,Shapelet-based Rules,Exemplars and Counter-Exemplars
AI 理解论文
溯源树
样例
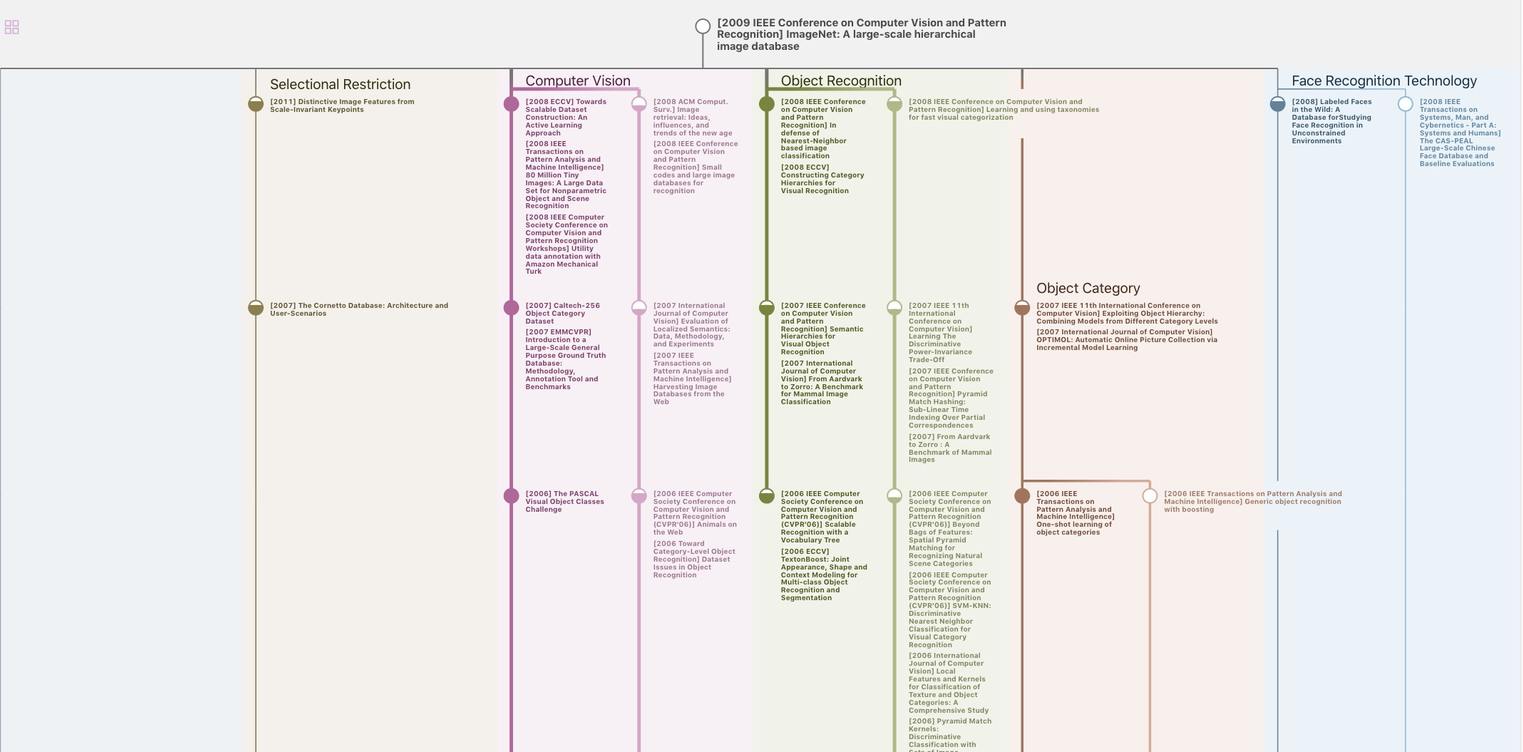
生成溯源树,研究论文发展脉络
Chat Paper
正在生成论文摘要