A formal proof of PAC learnability for decision stumps
CPP '21: 10th ACM SIGPLAN International Conference on Certified Programs and Proofs Virtual Denmark January, 2021(2021)
摘要
We present a formal proof in Lean of probably approximately correct (PAC) learnability of the concept class of decision stumps. This classic result in machine learning theory derives a bound on error probabilities for a simple type of classifier. Though such a proof appears simple on paper, analytic and measure-theoretic subtleties arise when carrying it out fully formally. Our proof is structured so as to separate reasoning about deterministic properties of a learning function from proofs of measurability and analysis of probabilities.
更多查看译文
关键词
interactive theorem proving, probably approximately correct, decision stumps
AI 理解论文
溯源树
样例
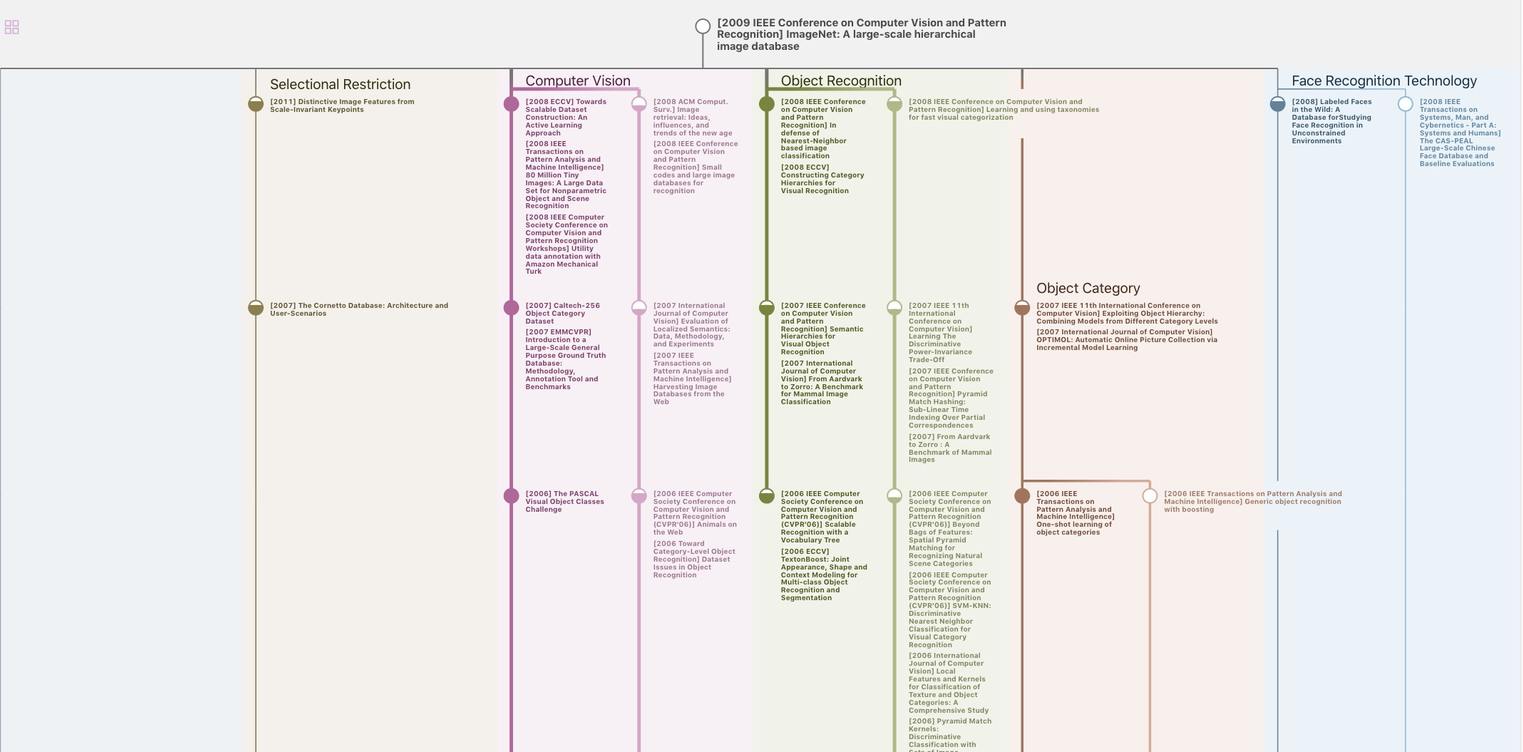
生成溯源树,研究论文发展脉络
Chat Paper
正在生成论文摘要