Dynamic IR-Drop ECO Optimization by Cell Movement with Current Waveform Staggering and Machine Learning Guidance.
ICCAD(2020)
摘要
Excessive dynamic IR-drop degrades the circuit performance and may lead to functional failure. Existing IR-drop fixing techniques at the placement stage do not consider the time-variant property and thus cannot handle dynamic IR-drop hotspots well. In current practice, designers perform Engineer Change Order (ECO) to move out these hotspot cells based on their experience. In this paper, we present a novel dynamic IR-drop ECO optimization and prediction framework by wise cell movement. We first spread high demand current cells in a global view to stagger their current waveforms. Then, we further move IR hotspot cells close to power/ground (PG) vias for minimizing the resistance from PG pads to their PG pins. Moreover, we propose an accurate machine learning-based dynamic IR-drop prediction model to guide the final cell movement. The features of our model capture power ground network characteristics, timing information, and cumulative current drawn by cells, thus leading to a general model applicable to ECO. Experimental results show that our proposed model precisely predicts dynamic IR-drop after cell movement, and our optimization scheme can substantially alleviate dynamic IR-drop without timing degradation.
更多查看译文
关键词
IR-drop,detailed placement,machine learning,ECO
AI 理解论文
溯源树
样例
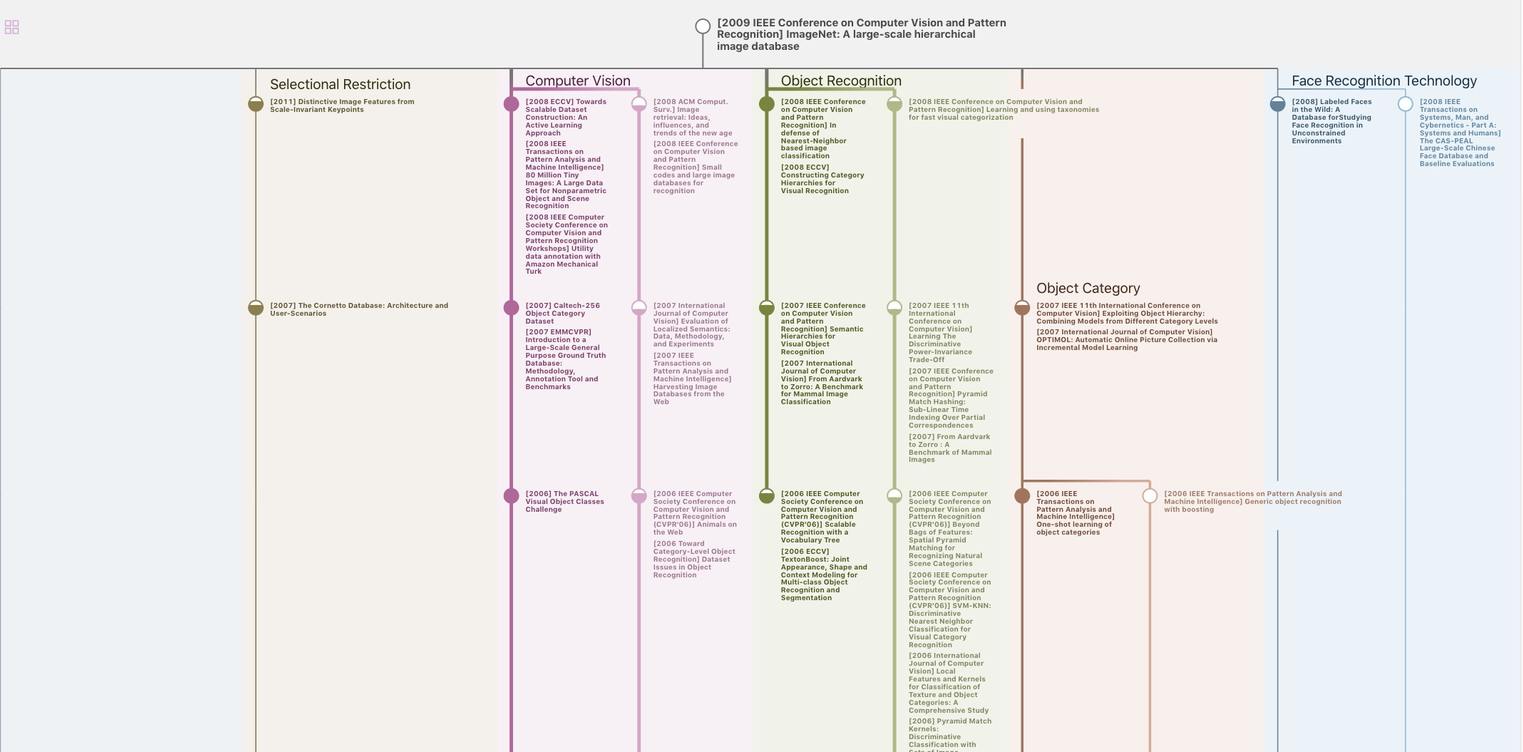
生成溯源树,研究论文发展脉络
Chat Paper
正在生成论文摘要