Layering-MCMC for Structure Learning in Bayesian Networks.
UAI(2020)
摘要
Bayesian inference of the Bayesian network structure requires averaging over all possible directed acyclic graphs, DAGs, each weighted by its posterior probability. For approximate averaging, the most popular method has been Markov chain Monte Carlo, MCMC. It was recently shown that collapsing the sampling space from DAGs to suitably defined ordered partitions of the nodes substantially expedites the chain’s convergence; this partition-MCMC is similar to order-MCMC on node orderings, but it avoids biasing the sampling distribution. Here, we further collapse the state space by merging some number of adjacent members of a partition into layers. This renders the computation of the (unnormalized) posterior probability of a state, called layering, more involved, for which task we give an efficient dynamic programming algorithm. Our empirical studies suggest that the resulting layering-MCMC is superior to partition-MCMC in terms of mixing time and estimation accuracy.
更多查看译文
关键词
bayesian networks,structure learning,layering-mcmc
AI 理解论文
溯源树
样例
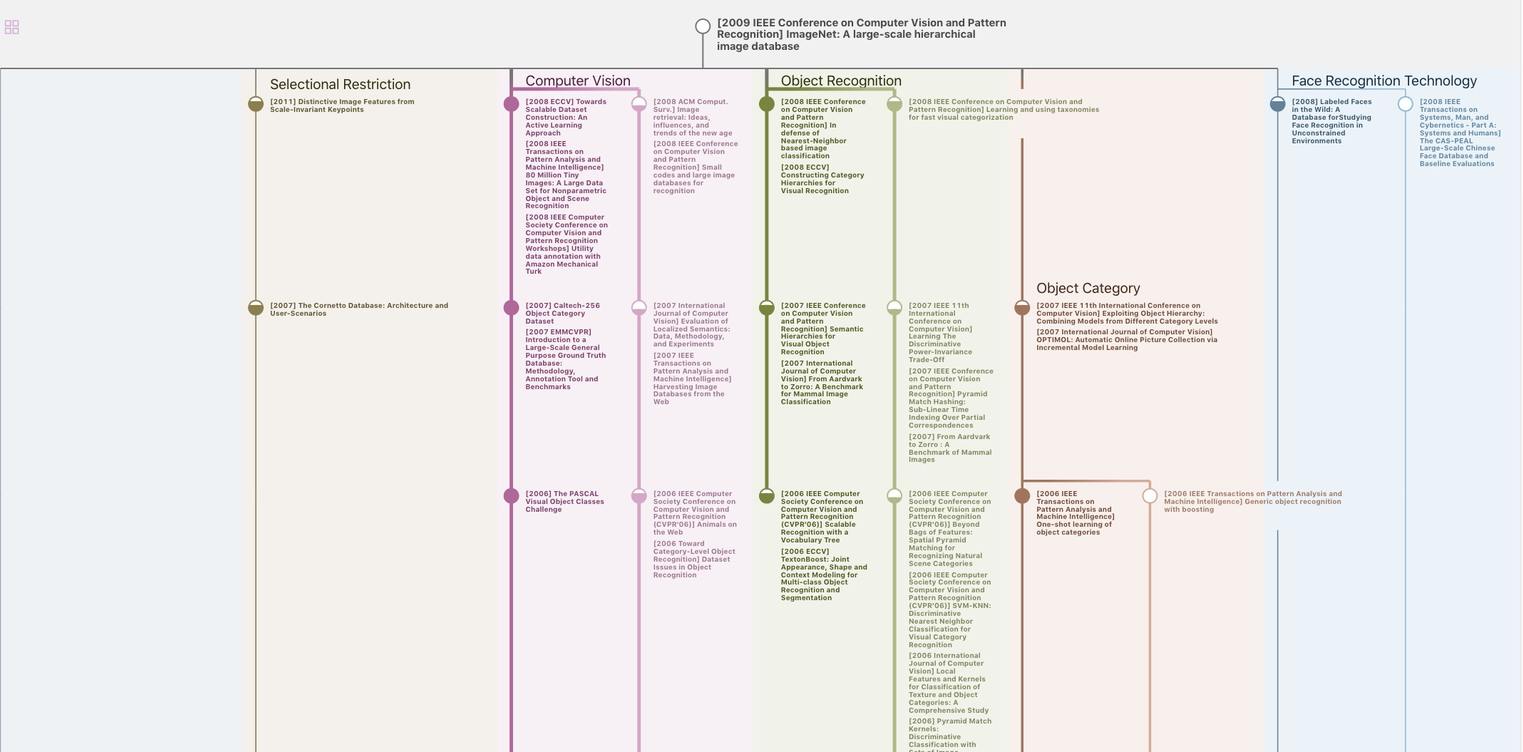
生成溯源树,研究论文发展脉络
Chat Paper
正在生成论文摘要