Autonomous Warehouse-Scale Computers
PROCEEDINGS OF THE 2020 57TH ACM/EDAC/IEEE DESIGN AUTOMATION CONFERENCE (DAC)(2020)
摘要
Modern Warehouse-Scale Computers (WSCs), composed of many generations of servers and a myriad of domain specific accelerators, are becoming increasingly heterogeneous. Meanwhile, WSC workloads are also becoming incredibly diverse with different communication patterns, latency requirements, and service level objectives (SLOs). Insufficient understanding of the interactions between workload characteristics and the underlying machine architecture leads to resource over-provisioning, thereby significantly impacting the utilization of WSCs.We present Autonomous Warehouse-Scale Computers, a new WSC design that leverages machine learning techniques and automation to improve job scheduling, resource management, and hardware-software co-optimization to address the increasing heterogeneity in WSC hardware and workloads. Our new design introduces two new layers in the WSC stack, namely: (a) a Software-Defined Server (SDS) Abstraction Layer which redefines the hardware-software boundary and provides greater control of the hardware to higher layers of the software stack through stable abstractions; and (b) a WSC Efficiency Layer which regularly monitors the resource usage of workloads on different hardware types, autonomously quantifies the performance sensitivity of workloads to key system configurations, and continuously improves scheduling decisions and hardware resource QoS policies to maximize cluster level performance. Our new WSC design has been successfully deployed across all WSCs at Google for several years now. The new WSC design improves throughput of workloads (by 7-10%, on average), increases utilization of hardware resources (up to 2x), and reduces performance variance for critical workloads (up to 25%).
更多查看译文
关键词
WSC, heterogeneity, machine learning, automation
AI 理解论文
溯源树
样例
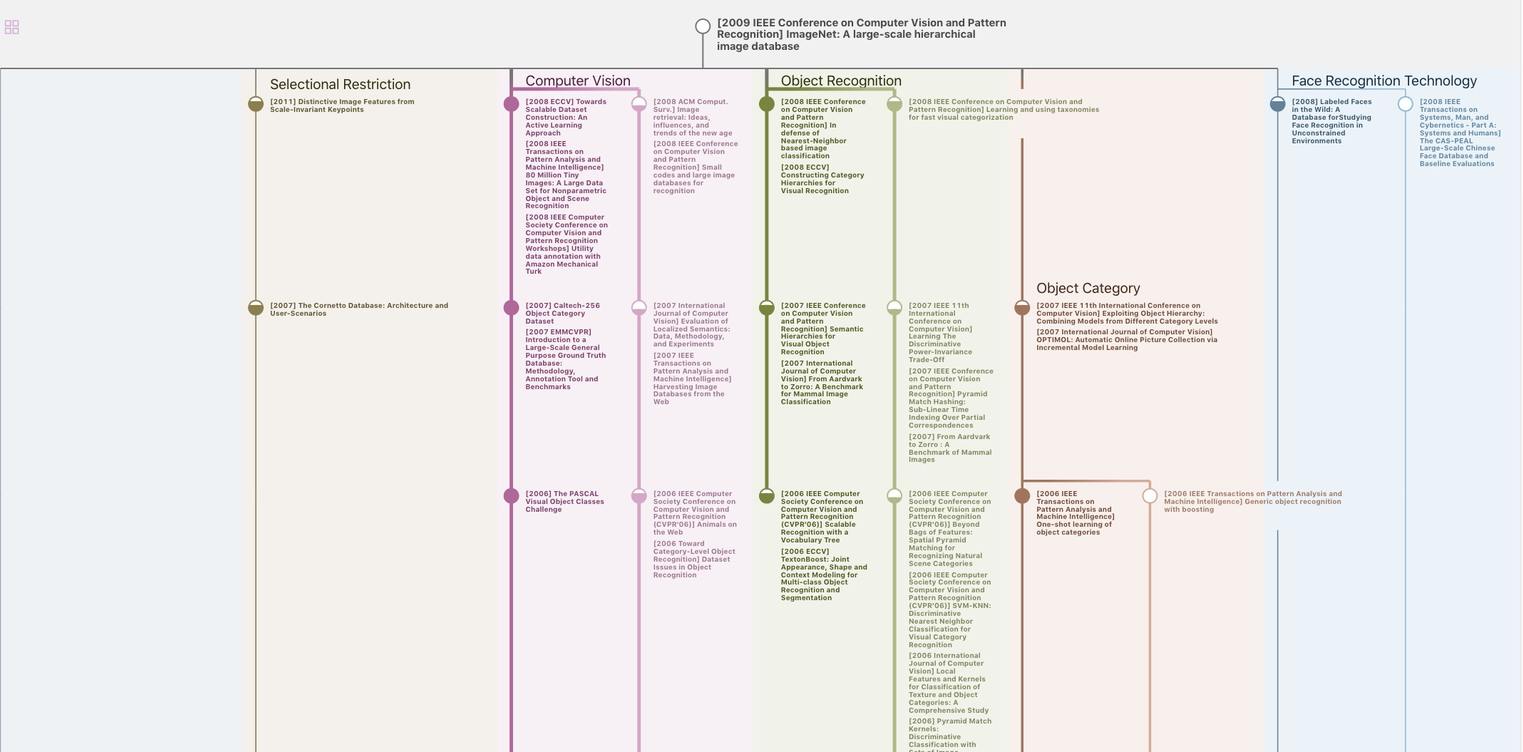
生成溯源树,研究论文发展脉络
Chat Paper
正在生成论文摘要