A Spiking Neural Architecture for Vector Quantization and Clustering.
ICONIP (2)(2020)
摘要
Although a couple of spiking neural network (SNN) architectures have been developed to perform vector quantization, good performances remains hard to attain. Moreover these architectures make use of rate codes that require an unplausible high number of spikes and consequently a high energetical cost. This paper presents for the first time a SNN architecture that uses temporal codes, more precisely first-spike latency code, while performing competitively with respect to the state-of-the-art visual coding methods. We developed a novel spike-timing-dependent plasticity (STDP) rule able to efficiently learn first-spike latency codes. This event-based rule is integrated in a two-layer SNN architecture of leaky integrate-and-fire (LIF) neurons. The first layer encodes a real-valued input vector in a spatio-temporal spike pattern, thus producing a temporal code. The second layer implements a distance-dependent lateral interaction profile making competitive and cooperative processes able to operate. The STDP rule operates between those two layers so as to learn the inputs by adapting the synaptic weights. State-of-the art performances are demonstrated on the MNIST and natural image datasets.
更多查看译文
关键词
spiking neural architecture,vector quantization
AI 理解论文
溯源树
样例
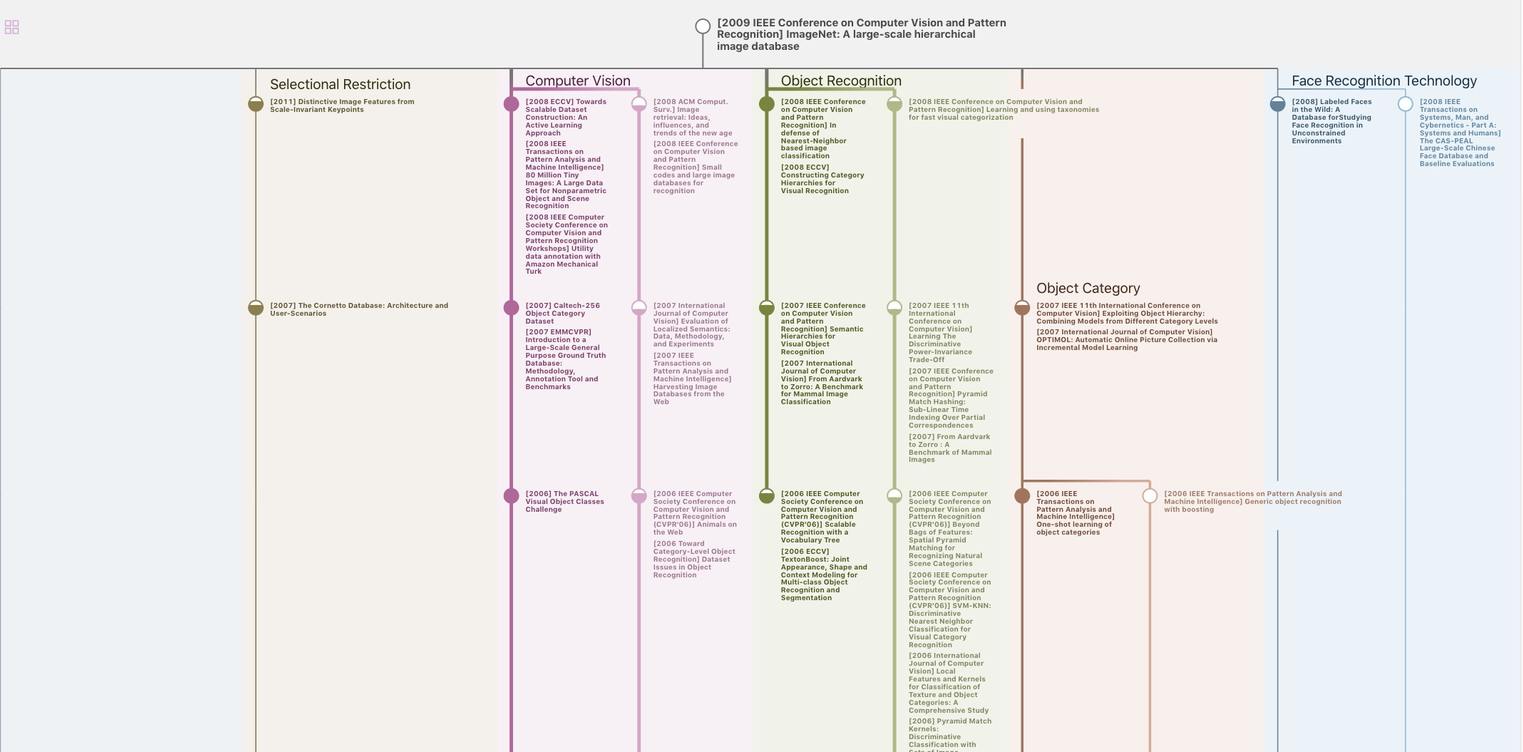
生成溯源树,研究论文发展脉络
Chat Paper
正在生成论文摘要