A High Throughput Hardware Cnn Accelerator Using A Novel Multi-Layer Convolution Processor
2020 28TH IRANIAN CONFERENCE ON ELECTRICAL ENGINEERING (ICEE)(2020)
摘要
Convolutional Neural Network (CNN) is the state-of-the-art deep learning approach used in various computer vision algorithms due to their high accuracy. To ensure programmable flexibility and shorten the development period, FPGA is an appropriate platform to implement CNN models. However, the limited on-chip storage and memory bandwidth are the bottlenecks. In this paper, two different architectures are presented to implement a same model structure. One performs traditional computing, layer by layer, and the other one performs multiple-layer computing in a pipeline structure using a Multi-Layer Convolution Processor (MLCP) accelerator. In the latter one, the required on-chip memory and memory bandwidth are reduced. Implementation results on a Xilinx Zynq XC7Z020 chip under a frequency of 200 MHz shows that the MLCP accelerator achieves 12.9 GOP/s that is 2.6x higher than that of Single-Layer Convolution Processor (SLCP).
更多查看译文
关键词
Convolution Neural Network (CNN), Hardware Implementation, FPGA pipeline, memory bandwidth
AI 理解论文
溯源树
样例
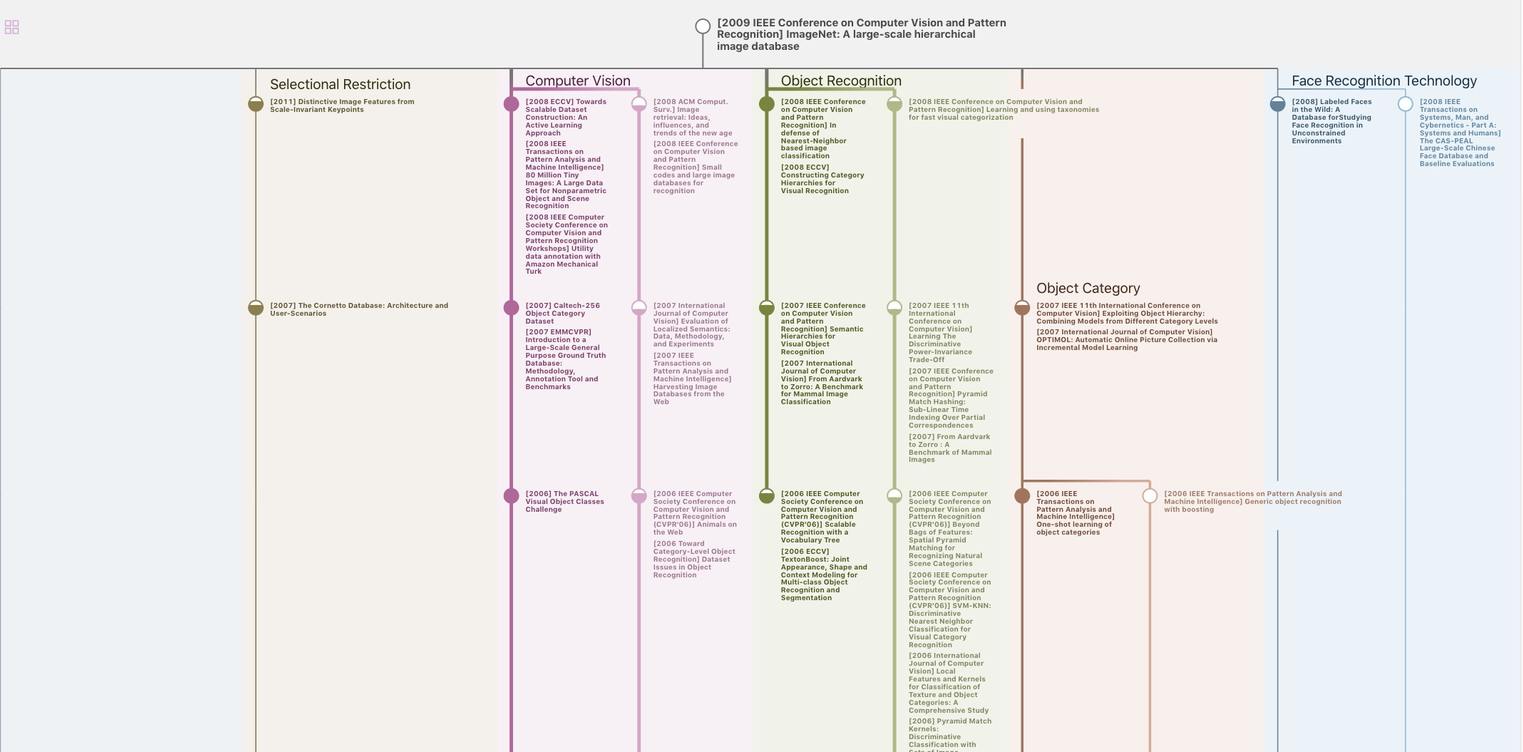
生成溯源树,研究论文发展脉络
Chat Paper
正在生成论文摘要