Toward Hardware-Efficient Optical Neural Networks: Beyond FFT Architecture via Joint Learnability
IEEE Transactions on Computer-Aided Design of Integrated Circuits and Systems(2021)
摘要
As a promising neuromorphic framework, the optical neural network (ONN) demonstrates ultrahigh inference speed with low energy consumption. However, the previous ONN architectures have high area overhead which limits their practicality. In this article, we propose an area-efficient ONN architecture based on structured neural networks, leveraging optical fast Fourier transform for efficient computation. A two-phase software training flow with structured pruning is proposed to further reduce the optical component utilization. Experimental results demonstrate that the proposed architecture can achieve 2.2- 3.7× area cost improvement compared with the previous singular value decomposition-based architecture with comparable inference accuracy. A novel optical microdisk-based convolutional neural network architecture with joint learnability is proposed as an extension to move beyond Fourier transform and multilayer perception, enabling hardware-aware ONN design space exploration with lower area cost, higher power efficiency, and better noise-robustness.
更多查看译文
关键词
Hardware-efficient,nanophotonics,neural network hardware,optical computing,performance optimization
AI 理解论文
溯源树
样例
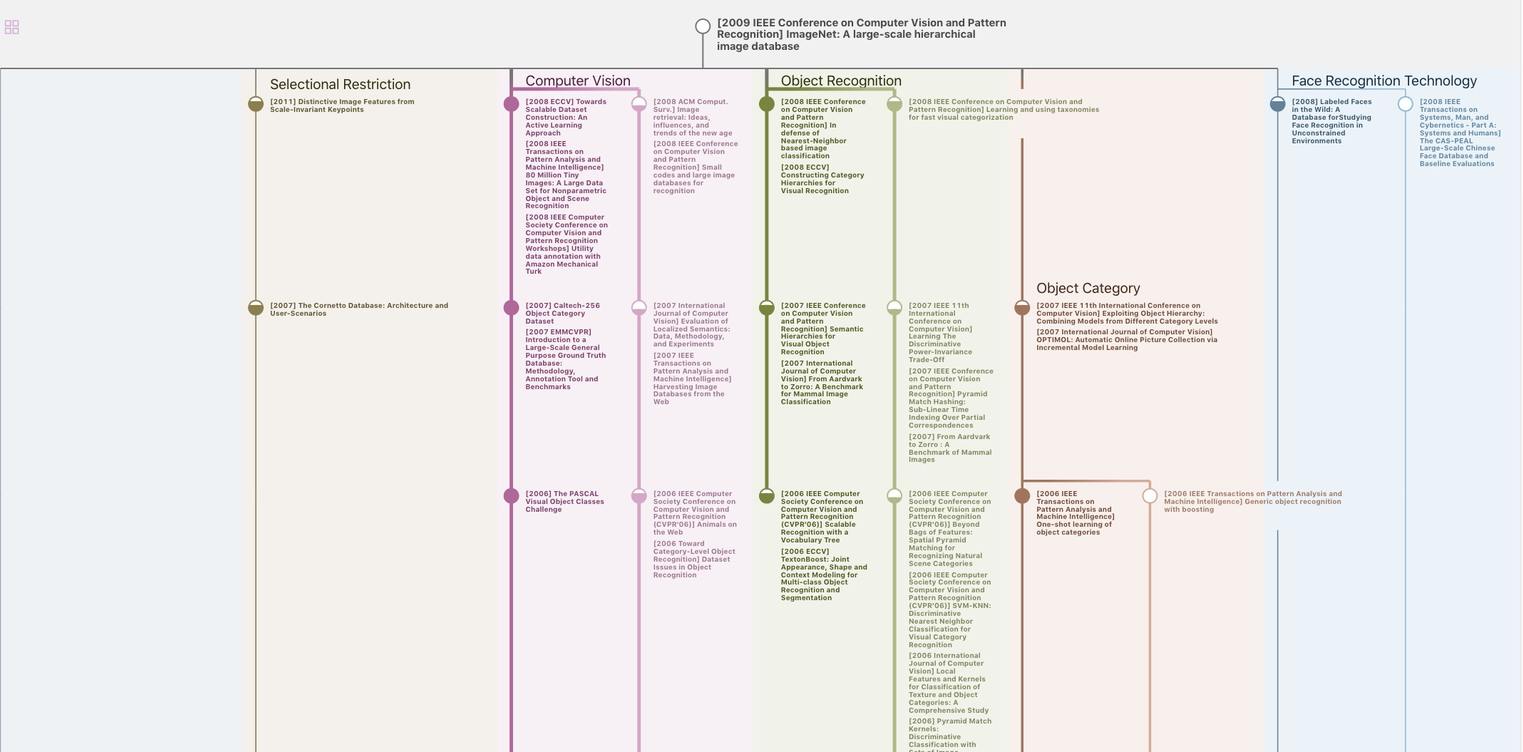
生成溯源树,研究论文发展脉络
Chat Paper
正在生成论文摘要