Spatial-Temporal Alignment Network for Action Recognition and Detection
arxiv(2020)
摘要
This paper studies how to introduce viewpoint-invariant feature representations that can help action recognition and detection. Although we have witnessed great progress of action recognition in the past decade, it remains challenging yet interesting how to efficiently model the geometric variations in large scale datasets. This paper proposes a novel Spatial-Temporal Alignment Network (STAN) that aims to learn geometric invariant representations for action recognition and action detection. The STAN model is very light-weighted and generic, which could be plugged into existing action recognition models like ResNet3D and the SlowFast with a very low extra computational cost. We test our STAN model extensively on AVA, Kinetics-400, AVA-Kinetics, Charades, and Charades-Ego datasets. The experimental results show that the STAN model can consistently improve the state of the arts in both action detection and action recognition tasks. We will release our data, models and code.
更多查看译文
关键词
action recognition,detection,spatial-temporal
AI 理解论文
溯源树
样例
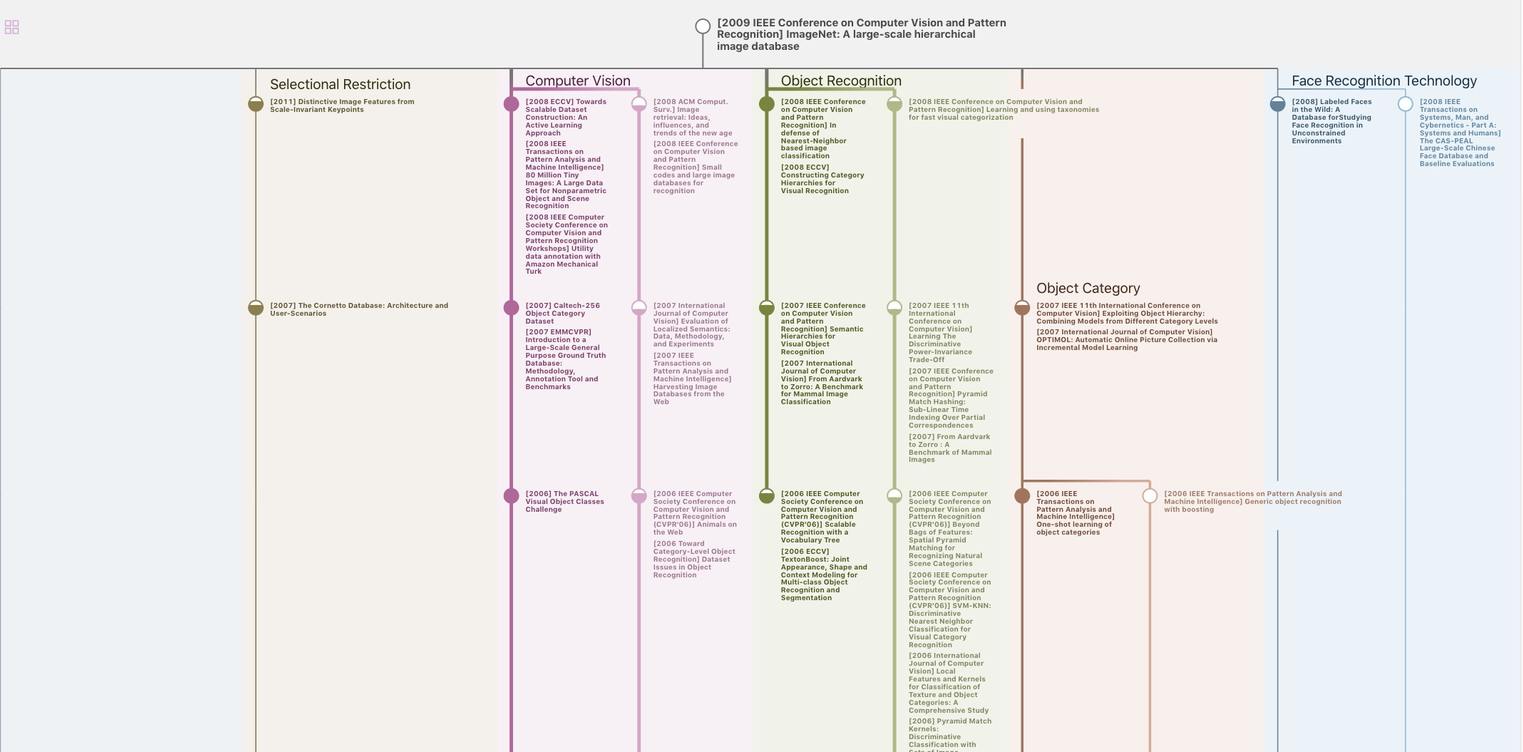
生成溯源树,研究论文发展脉络
Chat Paper
正在生成论文摘要