Analyzing Bin-width Effect on the Computed Entropy
AIP Conference Proceedings(2017)
摘要
The Shannon entropy is a mathematical expression for quantifying the amount of randomness which can be used to measure information content. It is used in objective function. Mutual Information (MI) uses Shannon entropy in order to determine shared information content of two images. The Shannon entropy, which was originally derived by Shannon in the context of lossless encoding of messages, is also used to define an optimum message length used in the Minimum Description Length (MDL) principle for groupwise registration. Majority of papers used histogram for computing MI, and hence the entropy. We therefore, aim to analyze the effect of bin-width on the computed entropy. We first derived the Shannon entropy from the integral of probability density function (pdf), and found that Gaussian has maximum entropy over all possible distribution. We also show that the entropy of the flat distribution is less than the entropy of the Gaussian distribution with the same variance. We then investigated the bin-width effect on the computed entropy, and analyzed the relationship between the computed entropy and the integral entropy when we vary bin-width, but fix variance and the number of samples. We then found that the value of the computed entropy lies within the theoretical predictions at small and large bin-widths. We also show two types of bias in entropy estimator.
更多查看译文
AI 理解论文
溯源树
样例
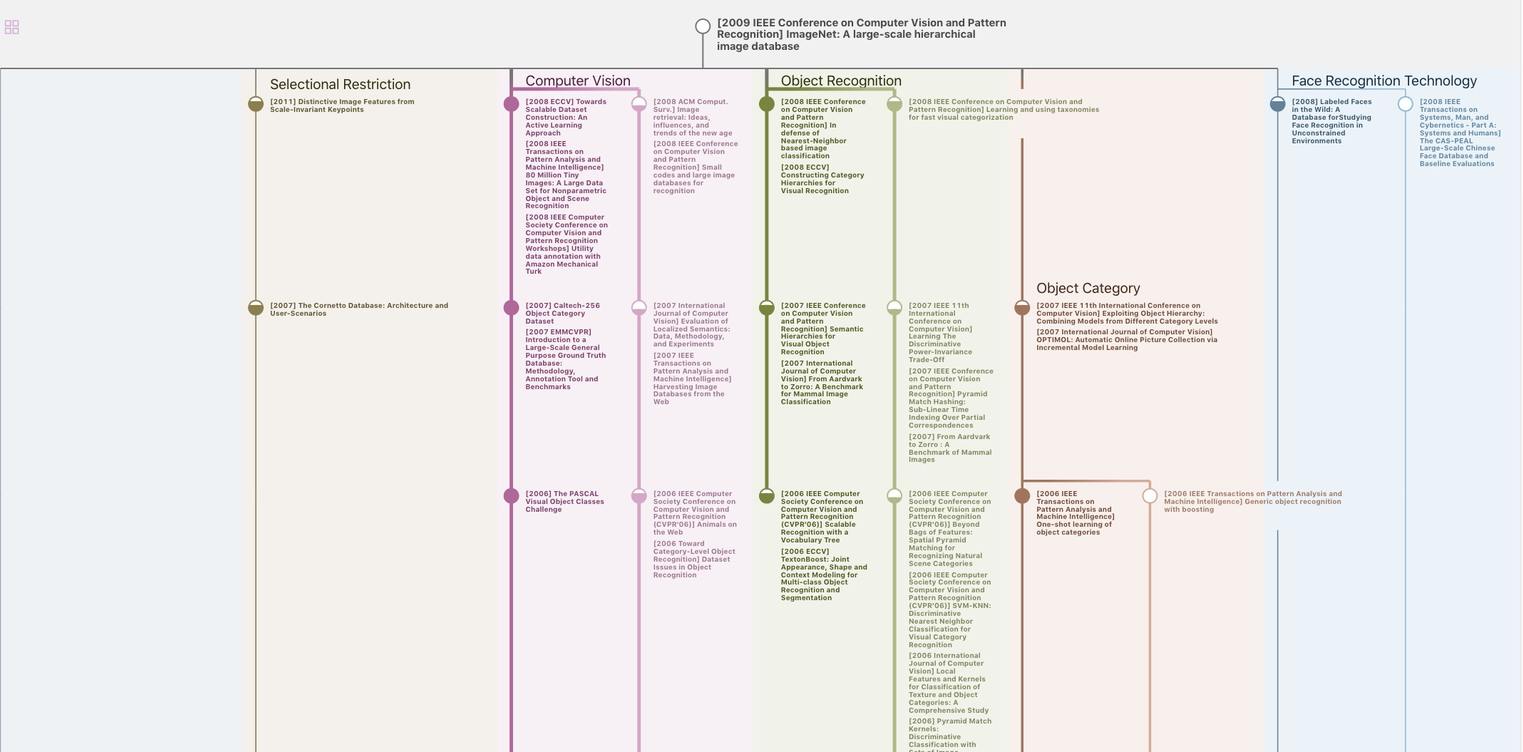
生成溯源树,研究论文发展脉络
Chat Paper
正在生成论文摘要