Reliable Off-Policy Evaluation for Reinforcement Learning
OPERATIONS RESEARCH(2024)
摘要
In a sequential decision-making problem, off-policy evaluation estimates the expected cumulative reward of a target policy using logged trajectory data generated from different behavior policy, without execution of the target policy. Reinforcement learning in high-stake environments, such as healthcare and education, is often limited to off-policy settings due to safety or ethical concerns or inability of exploration. Hence, it is imperative to quantify the uncertainty of the off-policy estimate before deployment of the target policy. In this paper, we propose a novel framework that provides robust and optimistic cumulative reward estimates using one or multiple logged trajectories data. Leveraging methodologies from distributionally robust optimization, we show that with proper selection of the size of the distributional uncertainty set, these estimates serve as confidence bounds with nonasymptotic and asymptotic guarantees under stochastic or adversarial environments. Our results are also generalized to batch reinforcement learning and are supported by empirical analysis.
更多查看译文
关键词
uncertainty quanti,reinforcement learning,Wasserstein robust optimization
AI 理解论文
溯源树
样例
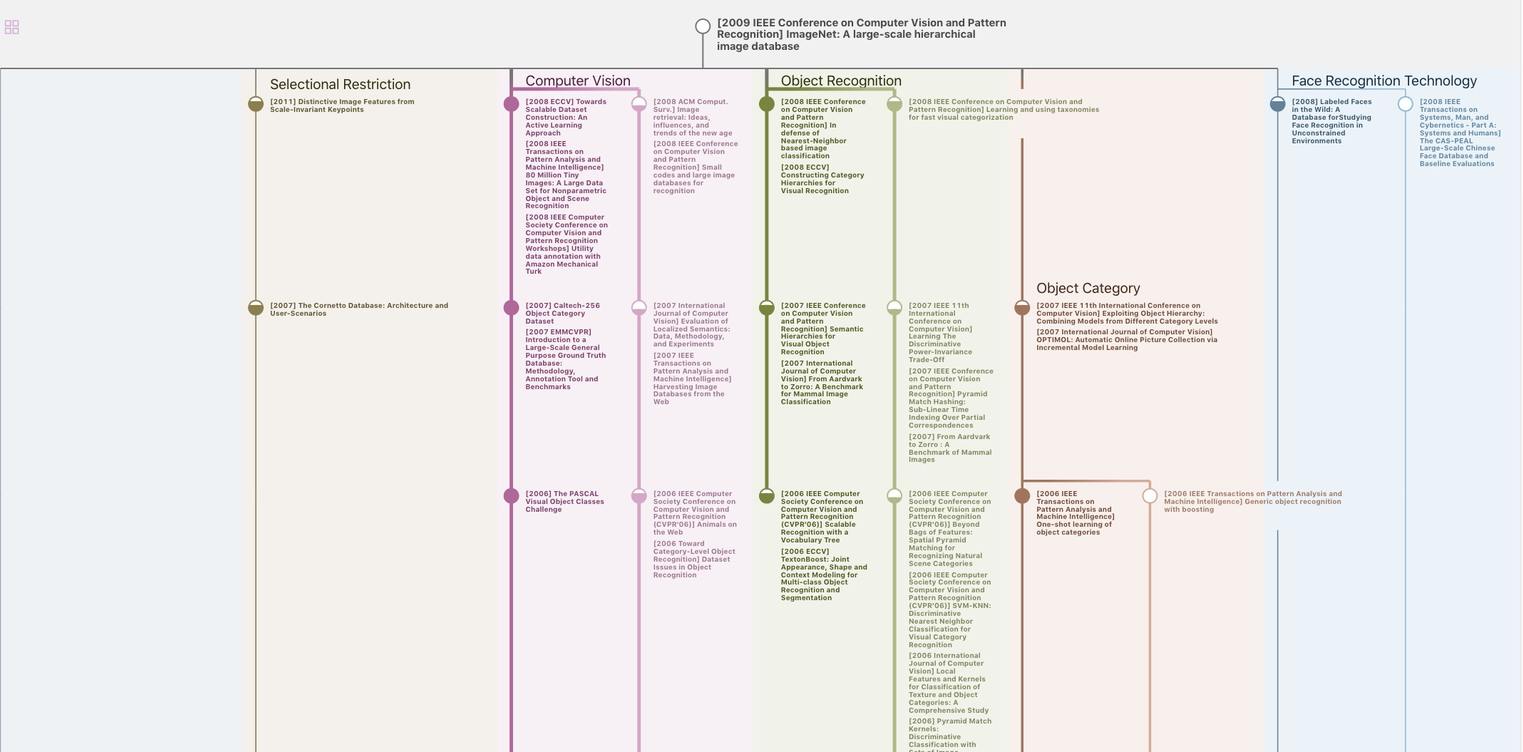
生成溯源树,研究论文发展脉络
Chat Paper
正在生成论文摘要