Causal Transfer Random Forest: Combining Logged Data and Randomized Experiments for Robust Prediction
WSDM(2021)
摘要
ABSTRACTIt is often critical for prediction models to be robust to distributional shifts between training and testing data. From a causal perspective, the challenge is to distinguish the stable causal relationships from the unstable spurious correlations across shifts. We describe a causal transfer random forest (CTRF) that combines existing training data with a small amount of data from a randomized experiment to train a model which is robust to the feature shifts and therefore transfers to a new targeting distribution. Theoretically, we justify the robustness of the approach against feature shifts with the knowledge from causal learning. Empirically, we evaluate the CTRF using both synthetic data experiments and real-world experiments in the Bing Ads platform, including a click prediction task and in the context of an end-to-end counterfactual optimization system. The proposed CTRF produces robust predictions and outperforms most baseline methods compared in the presence of feature shifts.
更多查看译文
关键词
Random forest,Causal learning,Transfer learning,Robust prediction models,Covariate shifts
AI 理解论文
溯源树
样例
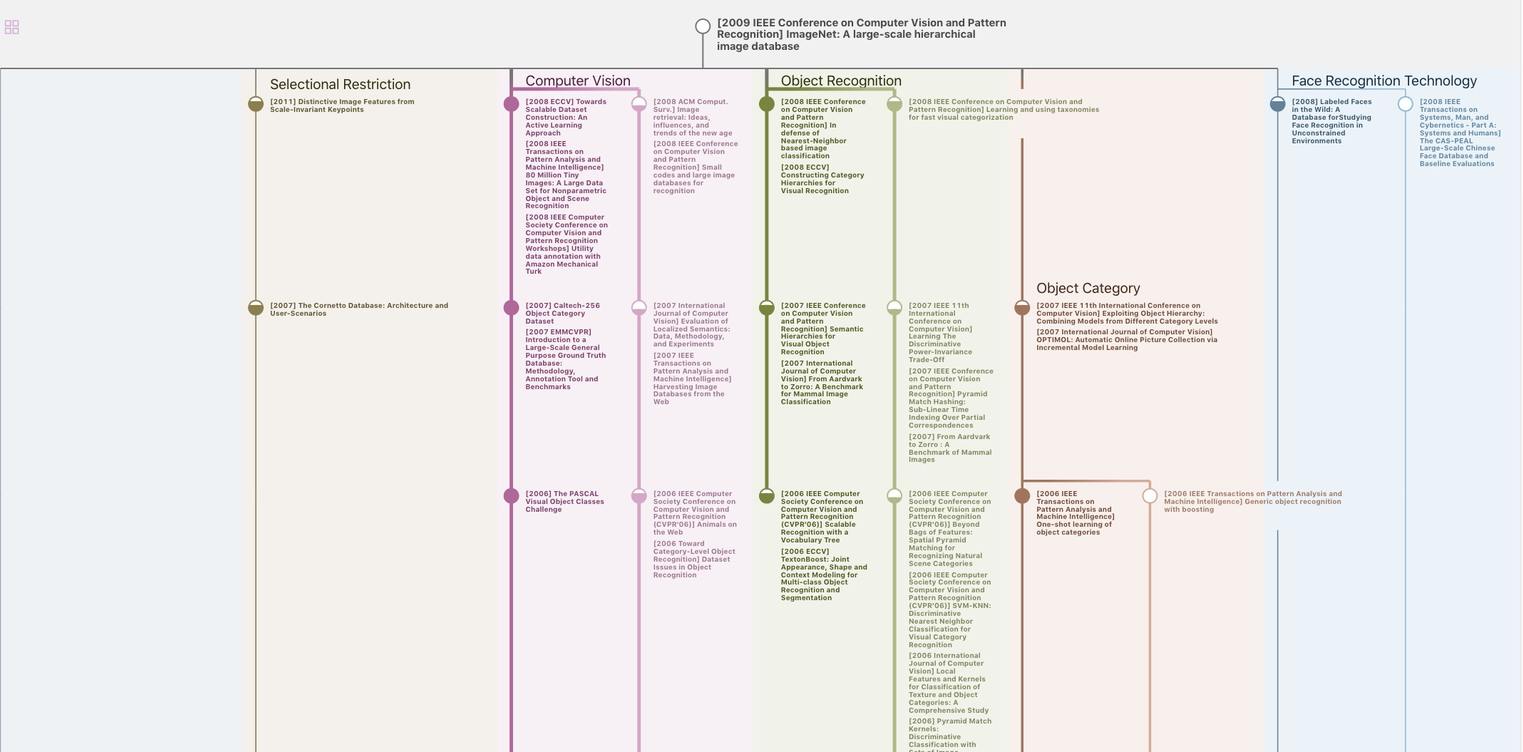
生成溯源树,研究论文发展脉络
Chat Paper
正在生成论文摘要