Modeling Preconditions in Text with a Crowd-sourced Dataset
EMNLP(2020)
摘要
Preconditions provide a form of logical connection between events that explains {why} some events occur together and information that is complementary to the more widely studied relations such as causation, temporal ordering, entailment, and discourse relations. Modeling preconditions in text has been hampered in part due to the lack of large scale labeled data grounded in text. This paper introduces PeKo, a crowd-sourced annotation of \emph{preconditions} between event pairs in newswire, an order of magnitude larger than prior text annotations. To complement this new corpus, we also introduce two challenge tasks aimed at modeling preconditions: (i) Precondition Identification -- a standard classification task defined over pairs of event mentions, and (ii) Precondition Generation -- a generative task aimed at testing a more general ability to reason about a given event. Evaluation on both tasks shows that modeling preconditions is challenging even for today's large language models (LM). This suggests that precondition knowledge is not easily accessible in LM-derived representations alone. Our generation results show that fine-tuning an LM on PeKo yields better conditional relations than when trained on raw text or temporally-ordered corpora.
更多查看译文
关键词
preconditions,text,crowd-sourced
AI 理解论文
溯源树
样例
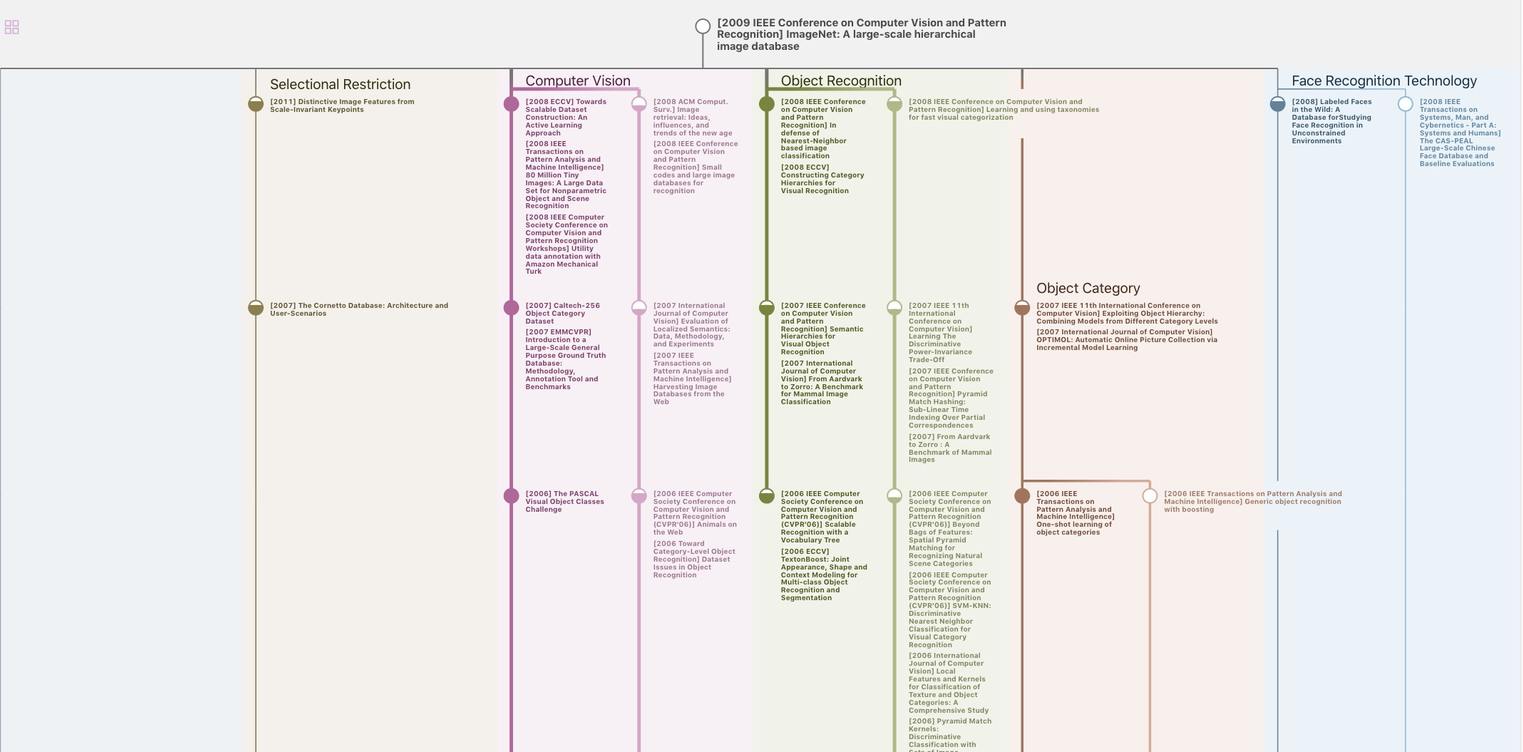
生成溯源树,研究论文发展脉络
Chat Paper
正在生成论文摘要