EqCo: Equivalent Rules for Self-supervised Contrastive Learning
arxiv(2023)
摘要
In this paper, we propose EqCo (Equivalent Rules for Contrastive Learning) to make self-supervised learning irrelevant to the number of negative samples in the contrastive learning framework. Inspired by the InfoMax principle, we point that the margin term in contrastive loss needs to be adaptively scaled according to the number of negative pairs in order to keep steady mutual information bound and gradient magnitude. EqCo bridges the performance gap among a wide range of negative sample sizes, so that for the first time, we can use only a few negative pairs (e.g., 16 per query) to perform self-supervised contrastive training on large-scale vision datasets like ImageNet, while with almost no accuracy drop. This is quite a contrast to the widely used large batch training or memory bank mechanism in current practices. Equipped with EqCo, our simplified MoCo (SiMo) achieves comparable accuracy with MoCov2 on ImageNet (linear evaluation protocol) while only involves 16 negative pairs per query instead of 65536, suggesting that large quantities of negative samples is not a critical factor in contrastive learning frameworks.
更多查看译文
AI 理解论文
溯源树
样例
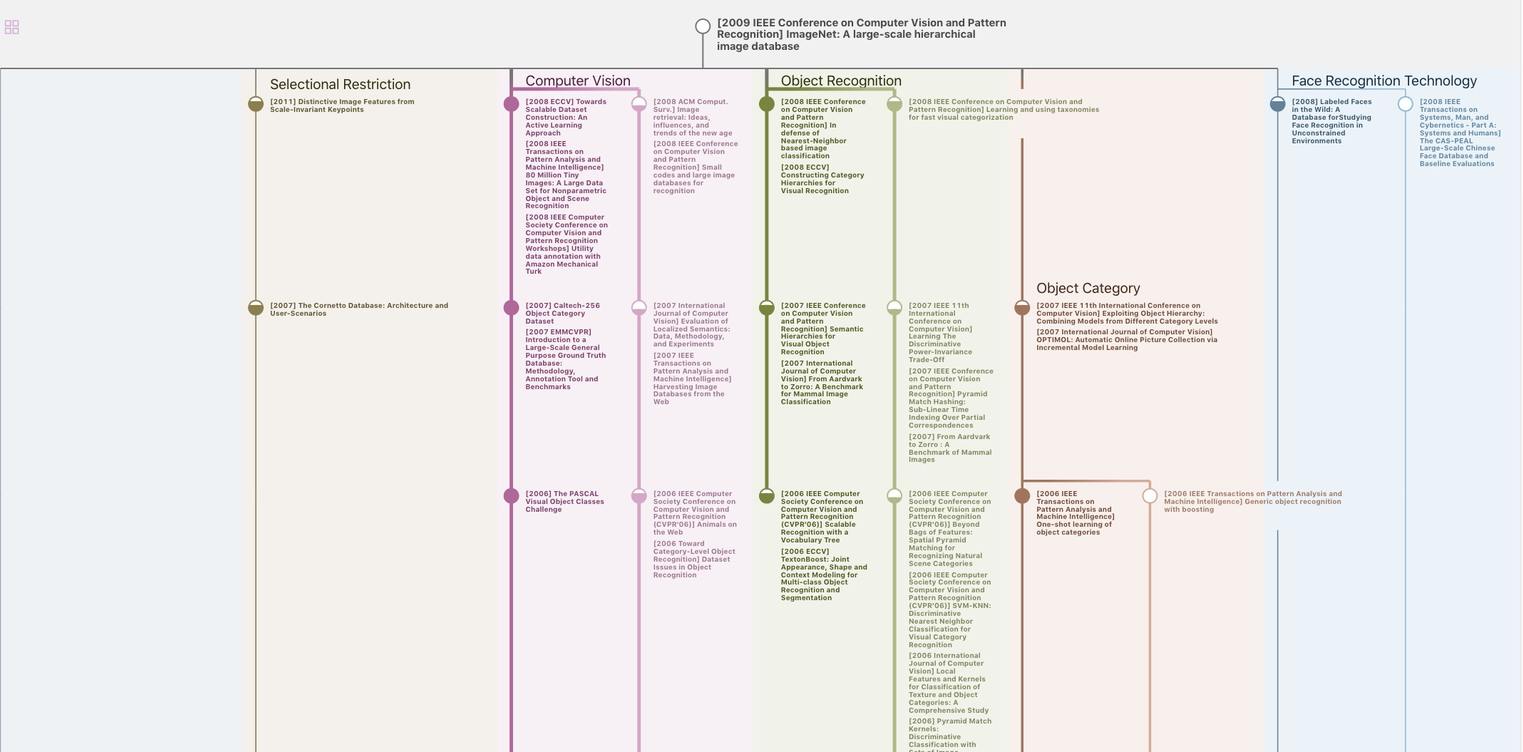
生成溯源树,研究论文发展脉络
Chat Paper
正在生成论文摘要