Regularized K-means through hard-thresholding
arxiv(2020)
摘要
We study a framework of regularized $K$-means methods based on direct penalization of the size of the cluster centers. Different penalization strategies are considered and compared through simulation and theoretical analysis. Based on the results, we propose HT $K$-means, which uses an $\ell_0$ penalty to induce sparsity in the variables. Different techniques for selecting the tuning parameter are discussed and compared. The proposed method stacks up favorably with the most popular regularized $K$-means methods in an extensive simulation study. Finally, HT $K$-means is applied to several real data examples. Graphical displays are presented and used in these examples to gain more insight into the datasets.
更多查看译文
关键词
k-means,hard-thresholding
AI 理解论文
溯源树
样例
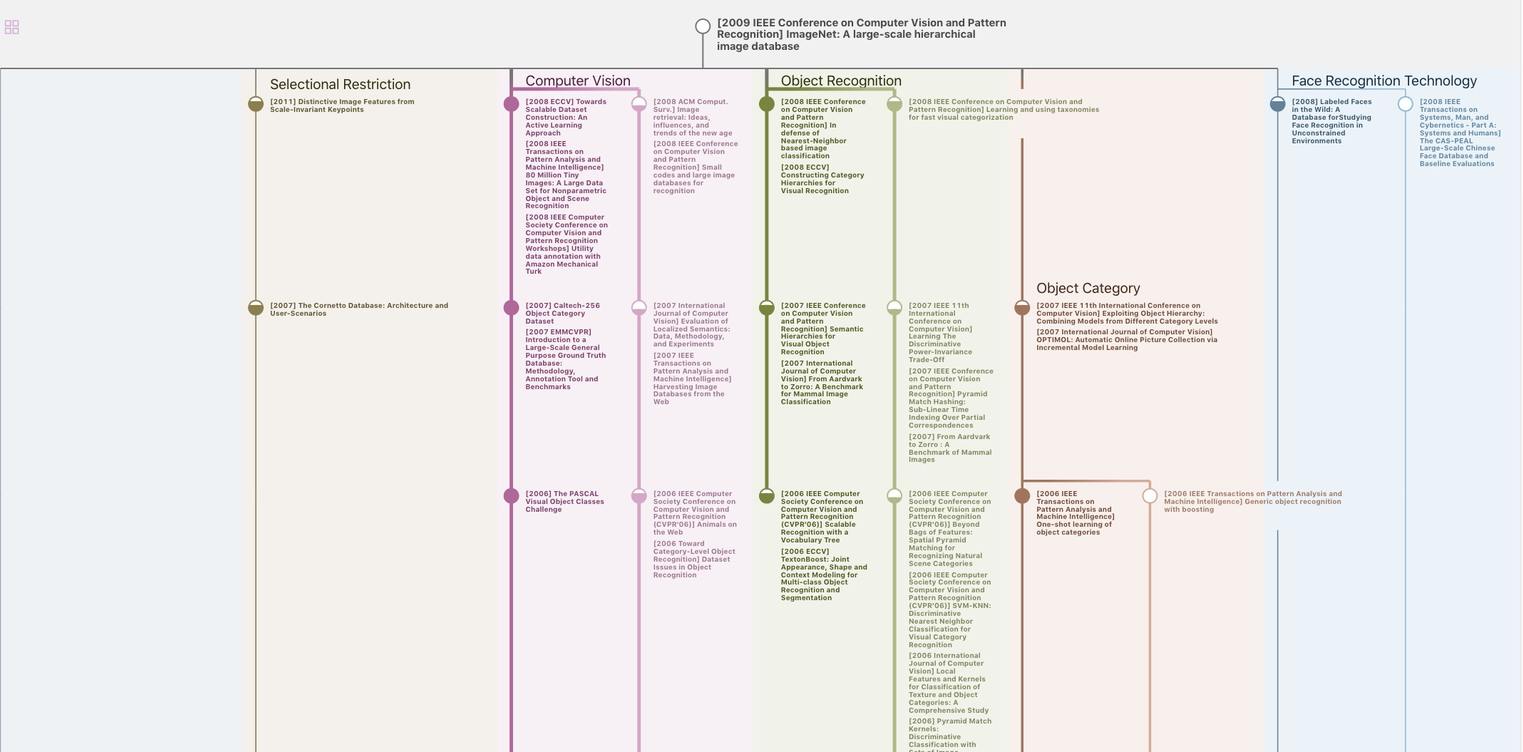
生成溯源树,研究论文发展脉络
Chat Paper
正在生成论文摘要