Empirical Research on Futures Trading Strategy Based on Time Series Algorithm.
CCIR(2020)
摘要
This article attempts to establish a trading strategy framework based on deep neural networks for the futures market, which consists of two parts: time series forecasting and trading strategies based on trading signals. In the time series forecasting task, we experimented with three types of methods with different entry points, namely recurrent neural networks with gate structure, networks combining time and frequency domain information, and network structures using attention mechanism. In the trading strategy part, the buying and selling signals and the corresponding trading volume are established according to the prediction results, and trading is conducted with the frequency of hours. In the empirical exploration part, we tested the prediction effect and strategic rate of return of various models on the copper contract. The data shows that in general, the best strategy can obtain a relatively stable income growth that has nothing to do with market fluctuations, but lacks countermeasures for rare external events with greater impact.
更多查看译文
关键词
futures trading strategy,time series
AI 理解论文
溯源树
样例
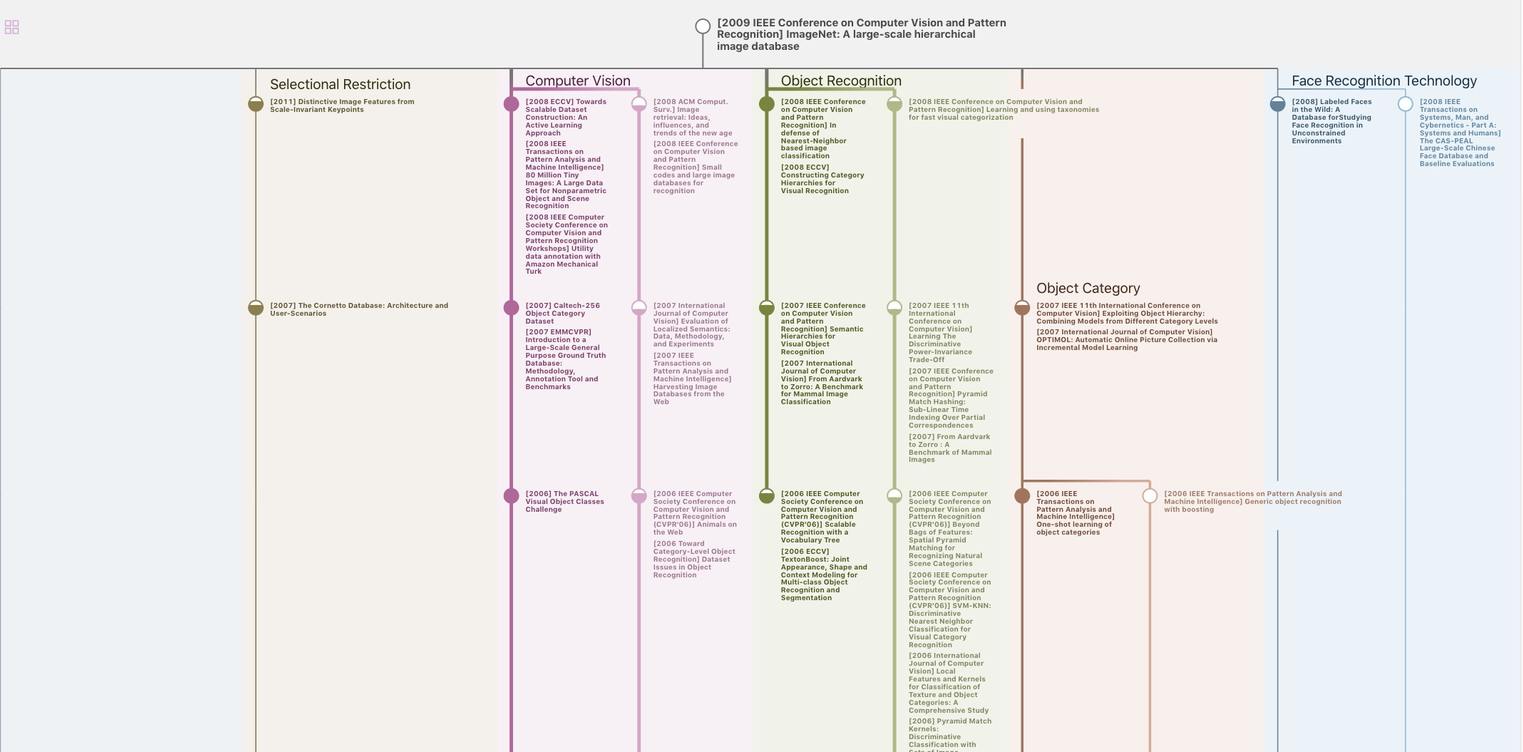
生成溯源树,研究论文发展脉络
Chat Paper
正在生成论文摘要