Downlink Power Control In Dense 5g Radio Access Networks Through Deep Reinforcement Learning
ICC 2020 - 2020 IEEE INTERNATIONAL CONFERENCE ON COMMUNICATIONS (ICC)(2020)
摘要
During the past decades, a myriad of inter/intracell interference mitigation techniques has been suggested for different wireless technologies. Nevertheless, the concept of downlink power control for interference mitigation has yet to be explored in 5G radio access networks. In this paper, we propose a data-driven approach based on deep reinforcement learning for downlink power control in dense 5G networks. The solution builds upon the well-known DQN algorithm and its recent extensions, aiming to maximize user rates. Using a 5Gcompliant system-level simulator, we compare the performance of our proposed method to fixed power allocation approaches. Test results show that the proposed method is successful at improving data rates at the cell-edge while reducing total transmitted power compared to the baseline.
更多查看译文
关键词
Downlink power control, interference mitigation, deep reinforcement learning, radio resource management
AI 理解论文
溯源树
样例
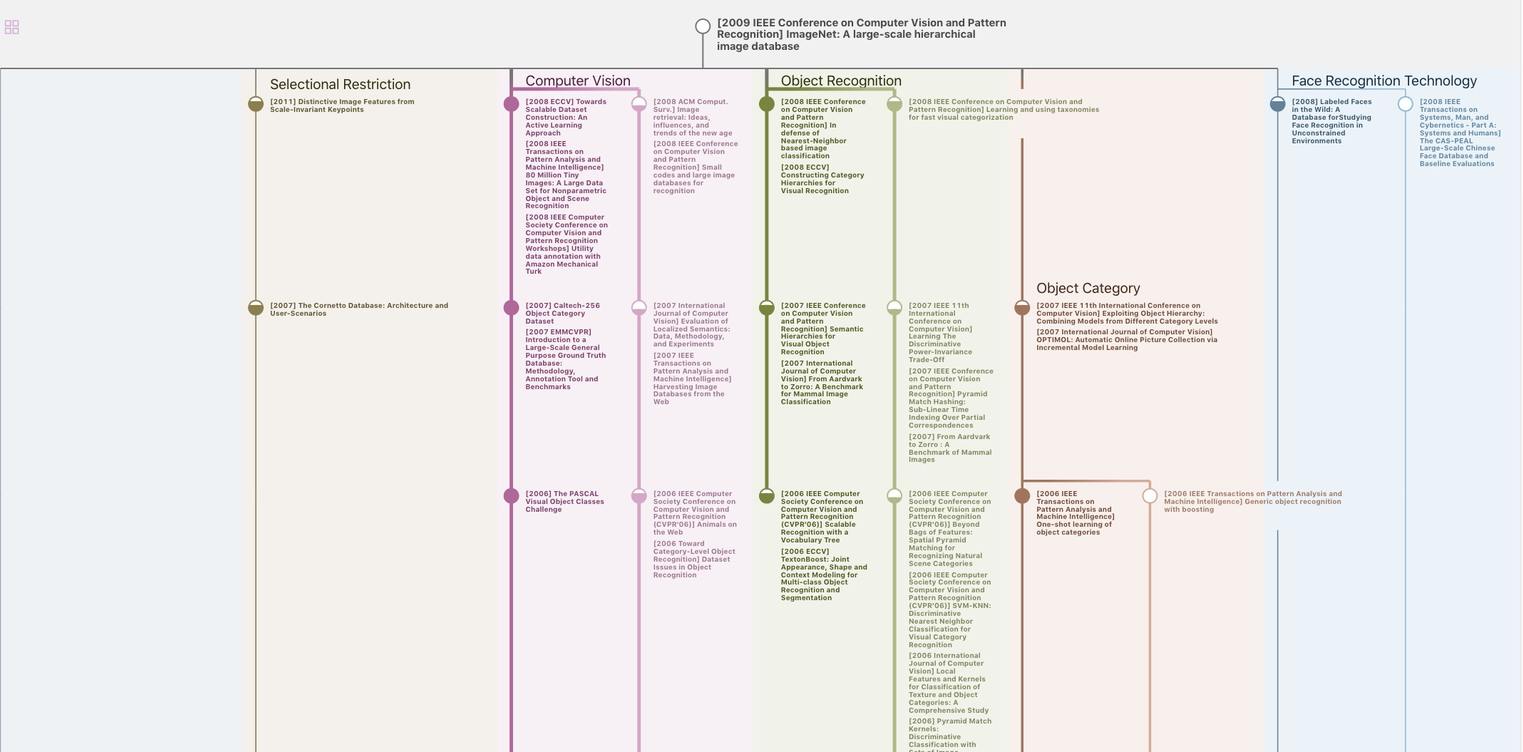
生成溯源树,研究论文发展脉络
Chat Paper
正在生成论文摘要