Land cover classification from remote sensing images based on multi-scale fully convolutional network
GEO-SPATIAL INFORMATION SCIENCE(2022)
摘要
Although the Convolutional Neural Network (CNN) has shown great potential for land cover classification, the frequently used single-scale convolution kernel limits the scope of information extraction. Therefore, we propose a Multi-Scale Fully Convolutional Network (MSFCN) with a multi-scale convolutional kernel as well as a Channel Attention Block (CAB) and a Global Pooling Module (GPM) in this paper to exploit discriminative representations from two-dimensional (2D) satellite images. Meanwhile, to explore the ability of the proposed MSFCN for spatio-temporal images, we expand our MSFCN to three-dimension using three-dimensional (3D) CNN, capable of harnessing each land cover category's time series interaction from the reshaped spatio-temporal remote sensing images. To verify the effectiveness of the proposed MSFCN, we conduct experiments on two spatial datasets and two spatiotemporal datasets. The proposed MSFCN achieves 60.366% on the WHDLD dataset and 75.127% on the GID dataset in terms of mIoU index while the figures for two spatiotemporal datasets are 87.753% and 77.156%. Extensive comparative experiments and ablation studies demonstrate the effectiveness of the proposed MSFCN. Code will be available at https://github.com/lironui/MSFCN.
更多查看译文
关键词
Spatio-temporal remote sensing images,Multi-Scale Fully Convolutional Network,land cover classification
AI 理解论文
溯源树
样例
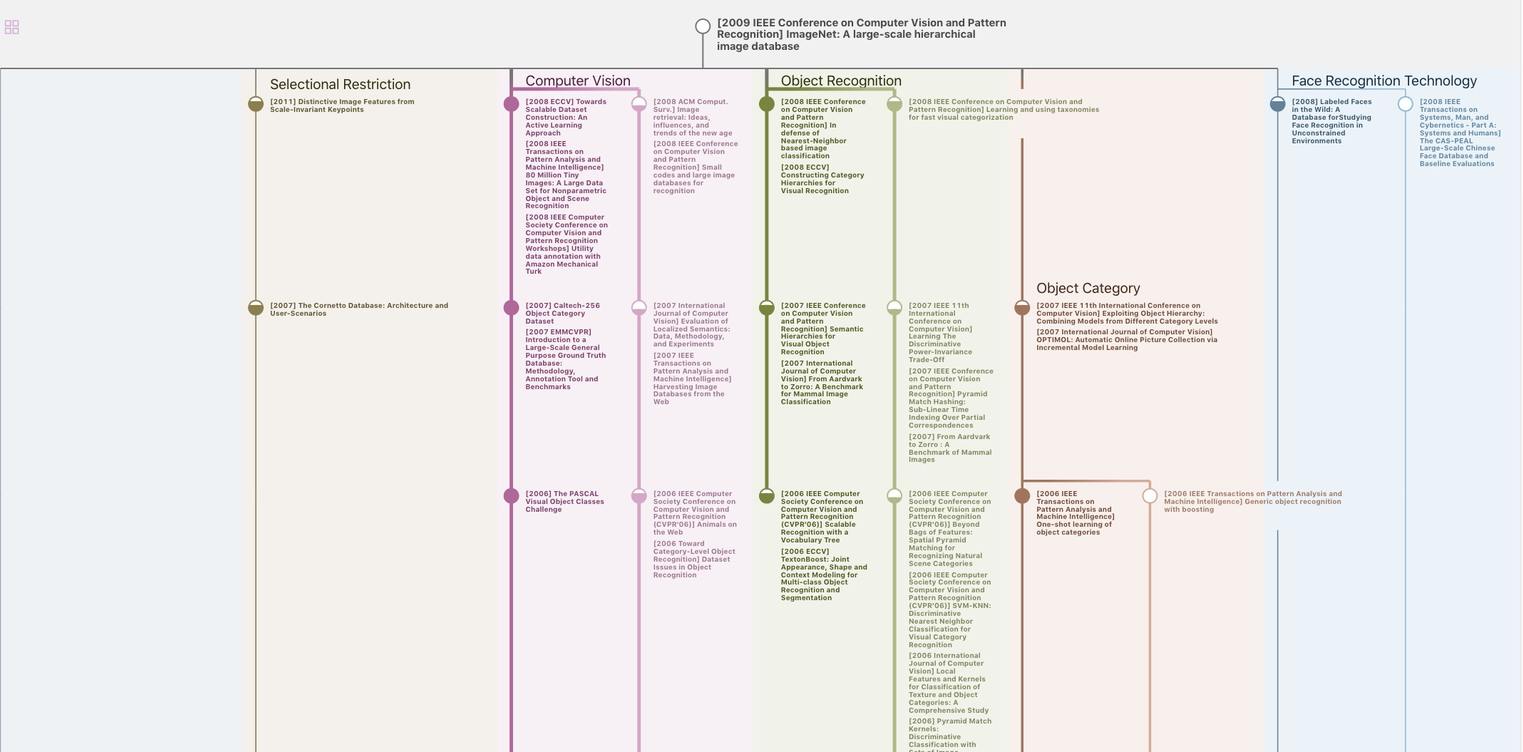
生成溯源树,研究论文发展脉络
Chat Paper
正在生成论文摘要