Regret Analysis of a Markov Policy Gradient Algorithm for Multiarm Bandits.
arxiv(2023)
摘要
We consider a policy gradient algorithm applied to a finite-arm bandit problem with Bernoulli rewards. We allow learning rates to depend on the current state of the algorithm rather than using a deterministic time-decreasing learning rate. The state of the algorithm forms a Markov chain on the probability simplex. We apply Foster-Lyapunov techniques to analyze the stability of this Markov chain. We prove that, if learning rates are well-chosen, then the policy gradient algorithm is a transient Markov chain, and the state of the chain converges on the optimal arm with logarithmic or polylogarithmic regret.
更多查看译文
关键词
regret, policy gradient, multiarm bandit, Markov chains, Foster
AI 理解论文
溯源树
样例
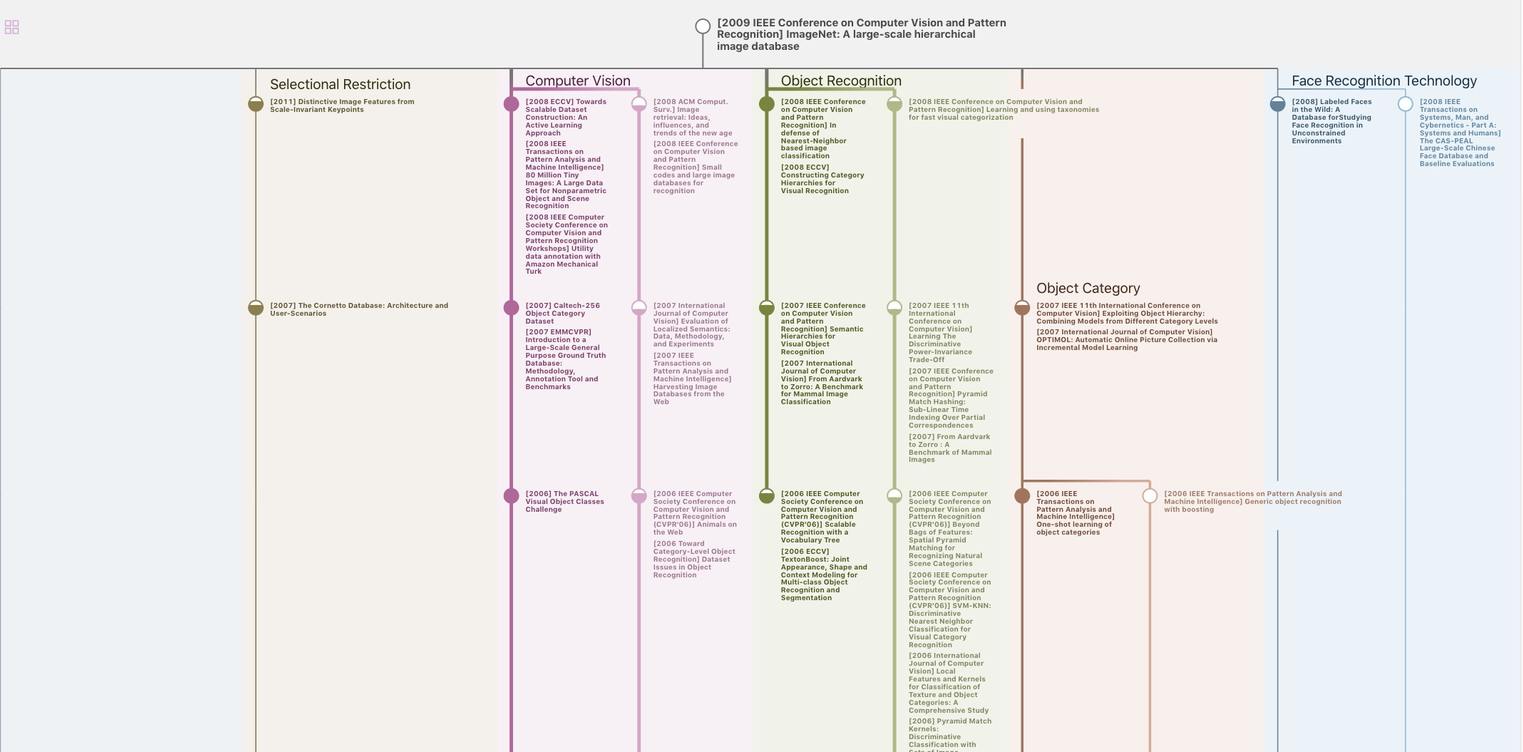
生成溯源树,研究论文发展脉络
Chat Paper
正在生成论文摘要