DDR-ID: dual deep reconstruction networks based image decomposition for anomaly detection
arxiv(2021)
摘要
Image based anomaly detection (AD) refers to the task of predicting an unseen image as the normal class (inliers) or the anomalous classes (outliers) by learning only from normal class training images. Such task is of significant value in real-world applications like monitoring anomalous event from surveillance cameras since most captured data reflect normal conditions but identification of anomalous data is required. One pivot challenge for image anomaly (AD) detection is to learn discriminative information only from normal class training images. Most image reconstruction based AD methods rely on the discriminative capability of reconstruction error. This is heuristic as image reconstruction is unsupervised without incorporating normal-class-specific information. In this paper, we propose an AD method called dual deep reconstruction networks based image decomposition (DDR-ID). The networks are trained by jointly optimizing for three losses: the one-class loss, the latent space constrain loss and the reconstruction loss. After training, DDR-ID can decompose an unseen image into its normal class and the residual components, respectively. Two anomaly scores are calculated to quantify the anomalous degree of the image in either normal class latent space or reconstruction image space. Thereby, anomaly detection can be performed via thresholding the anomaly score. The experiments demonstrate that DDR-ID outperforms multiple related benchmarking methods in image anomaly detection using MNIST, CIFAR-10 and Endosome datasets and adversarial attack detection using GTSRB dataset.
更多查看译文
关键词
Anomaly detection,Image decomposition,One-class classification
AI 理解论文
溯源树
样例
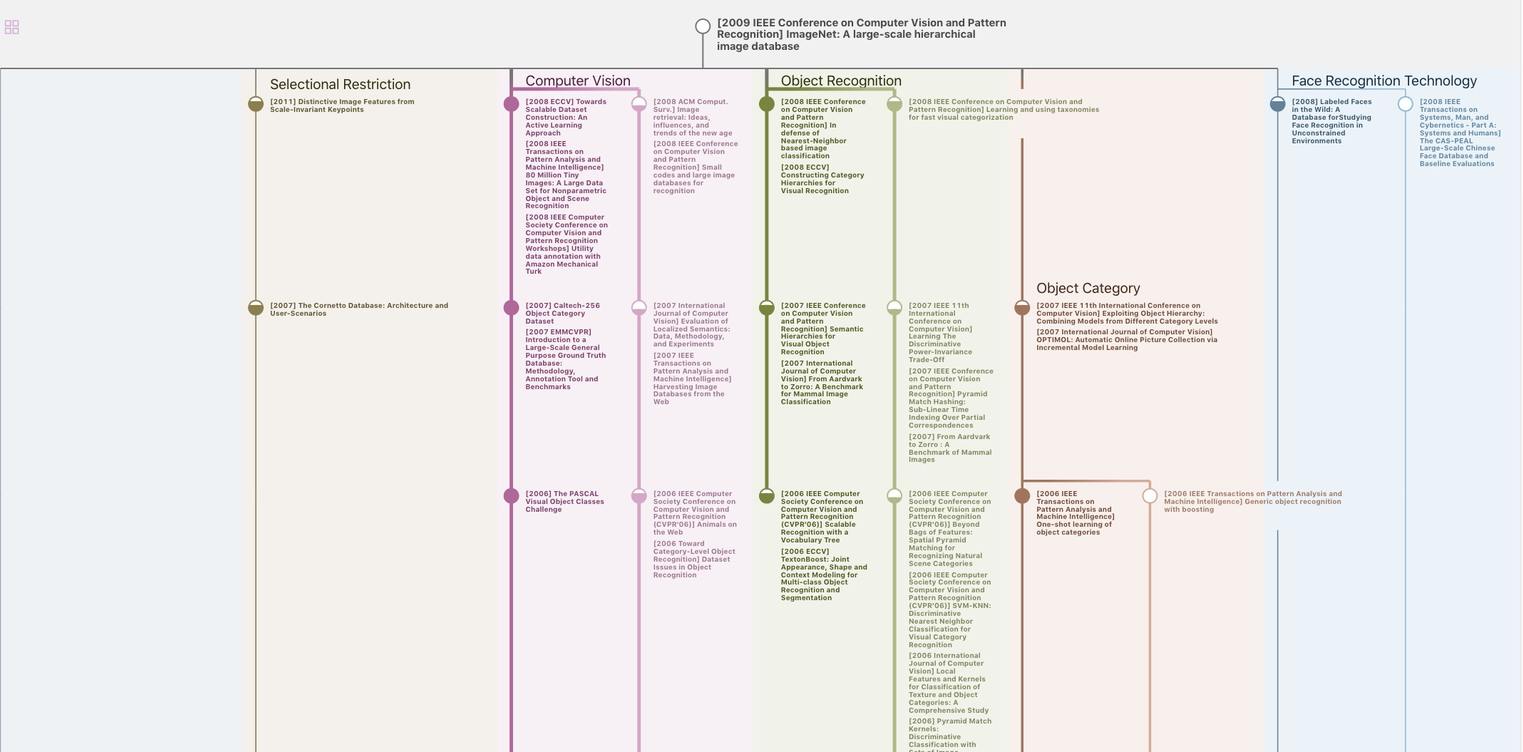
生成溯源树,研究论文发展脉络
Chat Paper
正在生成论文摘要