Speeding up Collaborative Filtering with Parametrized Preprocessing
semanticscholar(2015)
摘要
Collaborative filtering (CF) aims at producing recommendations for a user based on other users of similar taste, their k-neighbors. Since the computation of the neighborhood dominates the complexity of CF for a large number of users and ratings, this is done off-line in most commercial systems. As more and more systems allow users to continuously rate resources, neighborhoods are rapidly outdated and lose accuracy. Hence, neighborhoods have to be updated more often but traditional approaches do not meet the speed requirements. Our major contribution in this paper is to present a technique to split the computation of the neighborhood into an off-line and on-line task. This enables the system to speed up the on-line computation time up to 97% in relation to the time required by the state-of-theart approach, as our experiments on the MovieLens dataset demonstrate.
更多查看译文
AI 理解论文
溯源树
样例
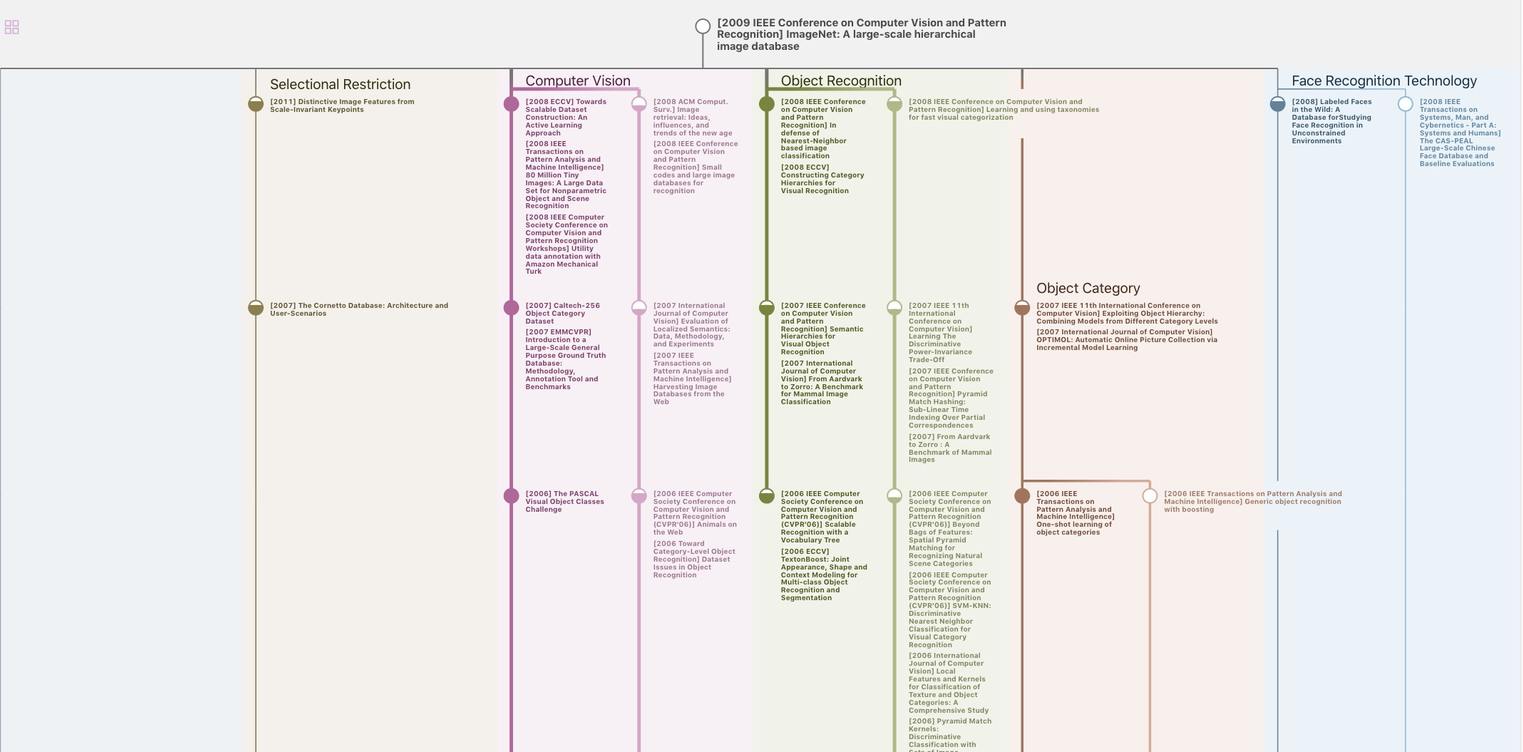
生成溯源树,研究论文发展脉络
Chat Paper
正在生成论文摘要