A data-driven approach to multi-stage stochastic linear optimization
MANAGEMENT SCIENCE(2020)
摘要
We propose a new data-driven approach for addressing multi-stage stochastic linear optimization problems with unknown distributions. The approach consists of solving a robust optimization problem that is constructed from sample paths of the underlying stochastic process. As more sample paths are obtained, we prove that the optimal cost of the robust problem converges to that of the underlying stochastic problem. To the best of our knowledge, this is the first data-driven approach for multi-stage stochastic linear optimization which is asymptotically optimal when uncertainty is arbitrarily correlated across time. Finally, we develop approximation algorithms for the proposed approach by extending techniques from the robust optimization literature, and demonstrate their practical value through numerical experiments on stylized data-driven inventory management problems.
更多查看译文
关键词
stochastic programming,robust optimization,sample-path approximations
AI 理解论文
溯源树
样例
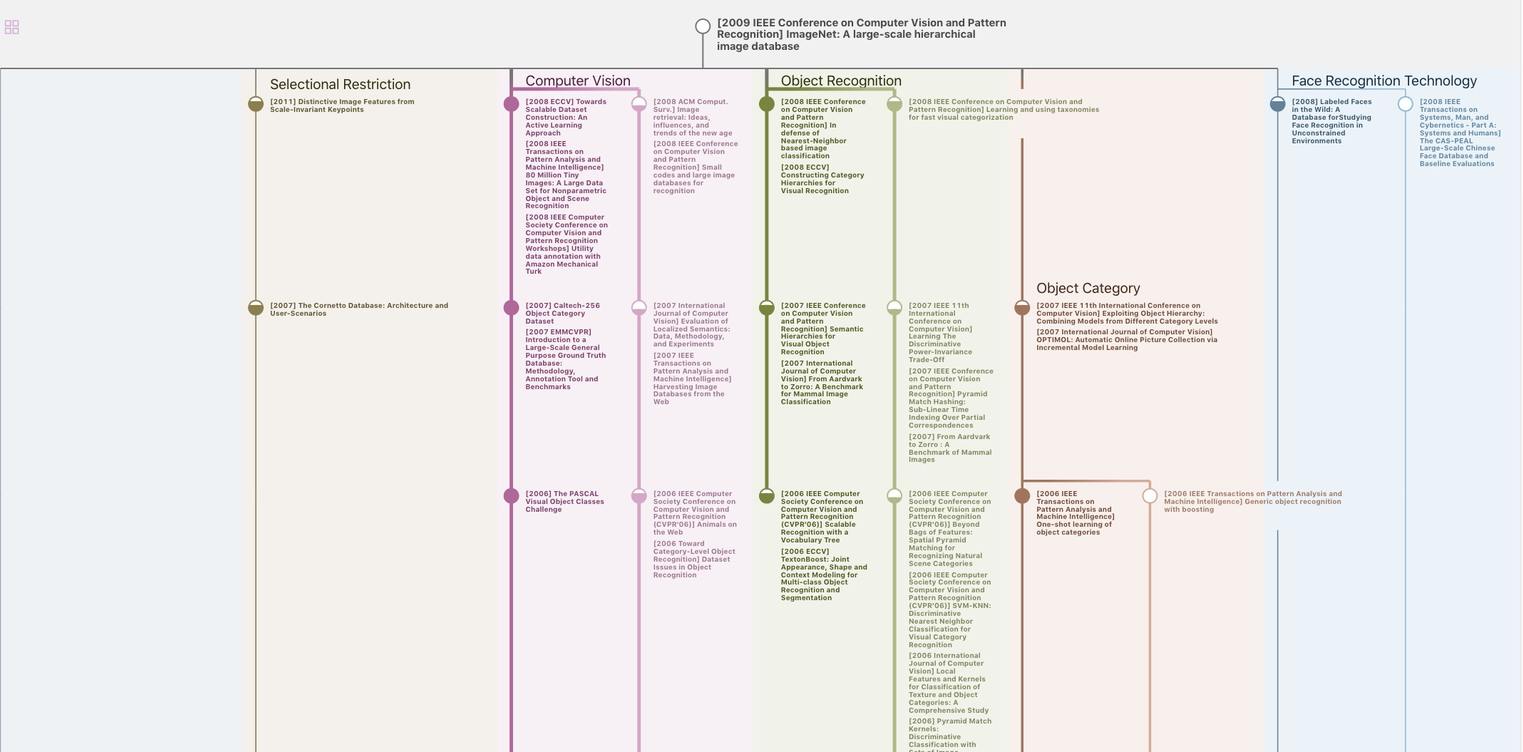
生成溯源树,研究论文发展脉络
Chat Paper
正在生成论文摘要