Cyber-guided Deep Neural Network for Malicious Repository Detection in GitHub
2020 IEEE International Conference on Knowledge Graph (ICKG)(2020)
摘要
As the largest source code repository, GitHub has played a vital role in modern social coding ecosystem to generate production software. Despite the apparent benefits of such social coding paradigm, its potential security risks have been largely overlooked (e.g., malicious codes or repositories could be easily embedded and distributed). To address this imminent issue, in this paper, we propose a novel framework (named GitCyber) to automate malicious repository detection in GitHub at the first attempt. In GitCyber, we first extract code contents from the repositories hosted in GitHub as the inputs for deep neural network (DNN), and then we incorporate cybersecurity domain knowledge modeled by heterogeneous information network (HIN) to design cyber-guided loss function in the learning objective of the DNN to assure the classification performance while preserving consistency with the observational domain knowledge. Comprehensive experiments based on the large-scale data collected from GitHub demonstrate that our proposed GitCyber outperforms the state-of-the-arts in malicious repository detection.
更多查看译文
关键词
Cyber-guided DNN,heterogeneous information network,malicious repository detection
AI 理解论文
溯源树
样例
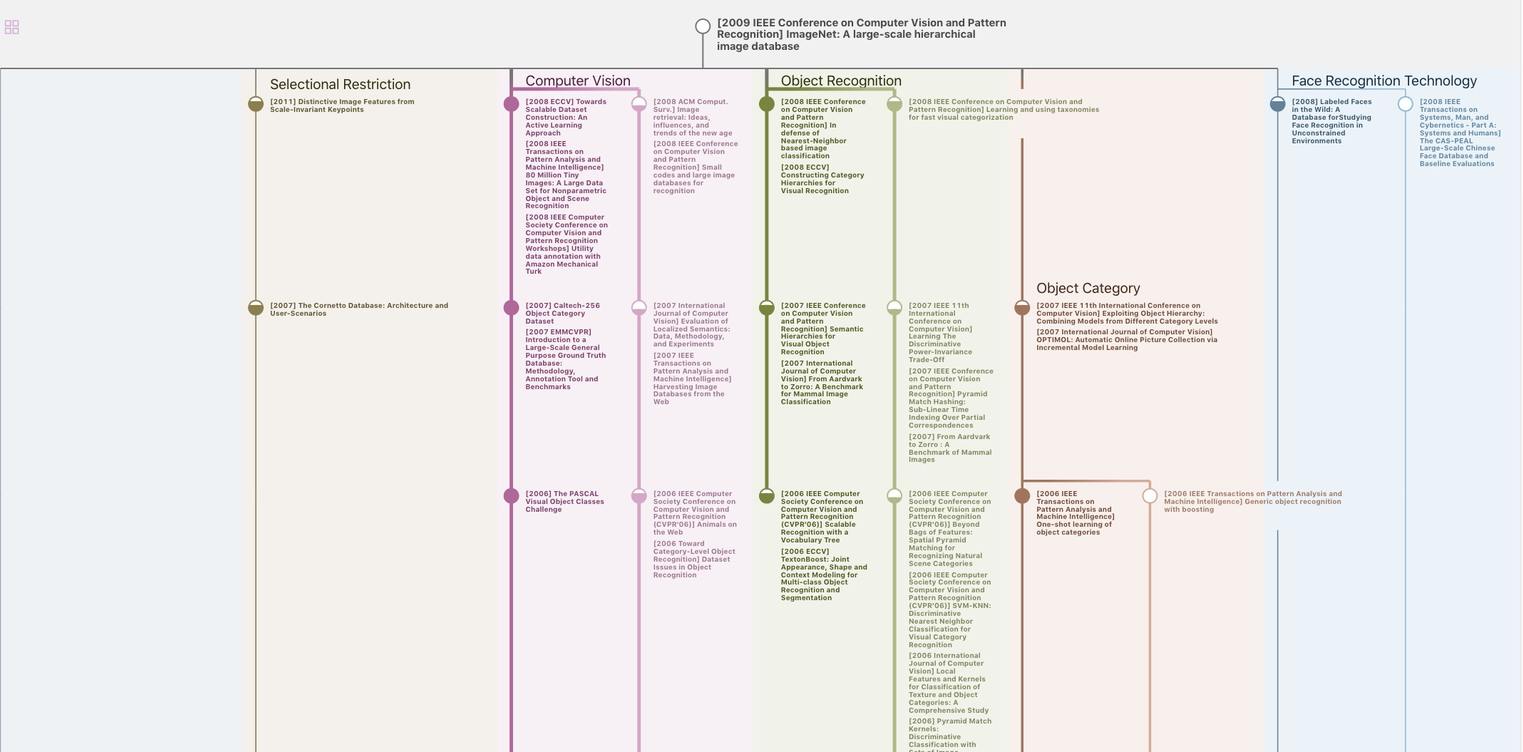
生成溯源树,研究论文发展脉络
Chat Paper
正在生成论文摘要