ExhaleSense: Detecting High Fidelity Forced Exhalations to Estimate Lung Obstruction on Smartphones
2020 IEEE International Conference on Pervasive Computing and Communications (PerCom)(2020)
摘要
Spirometry is the gold standard to measure lung functions by estimating the maximum air an individual can forcefully exhale as quickly as possible. It is used not only to diagnose lung diseases such as asthma, chronic obstructive pulmonary disease (COPD) but also to assess the severity of the pulmonary condition. However, spirometry requires a specialized device called spirometer, which is mostly available in clinical facilities and cumbersome to use. Recent works have shown the feasibility of using smartphone microphone to estimate lung functions from forced exhalation effort sounds. However, maintaining the fidelity of lung function estimation on smartphones becomes challenging in unsupervised field environment in presence of other sounds such as coughs, deep inhalation, regular breathing, and speech. In this paper, we present ExhaleSense that detects forced exhalation efforts on smartphones from audio time-series data, distinguishes high fidelity efforts from poor efforts, and estimates lung obstruction. By conducting three studies with 211 pulmonary patients and healthy subjects, we show that ExhaleSense can detect forced exhalation sounds with 96.74% F1-score and estimate lung obstruction with mean absolute error as low as 7.57%. ExhaleSense shifts the gear of smartphone spirometry research from feasibility to ensuring effort quality towards high fidelity lung function estimation in unsupervised field settings.
更多查看译文
关键词
mobile spirometry,pulmonary patient,audio,data quality
AI 理解论文
溯源树
样例
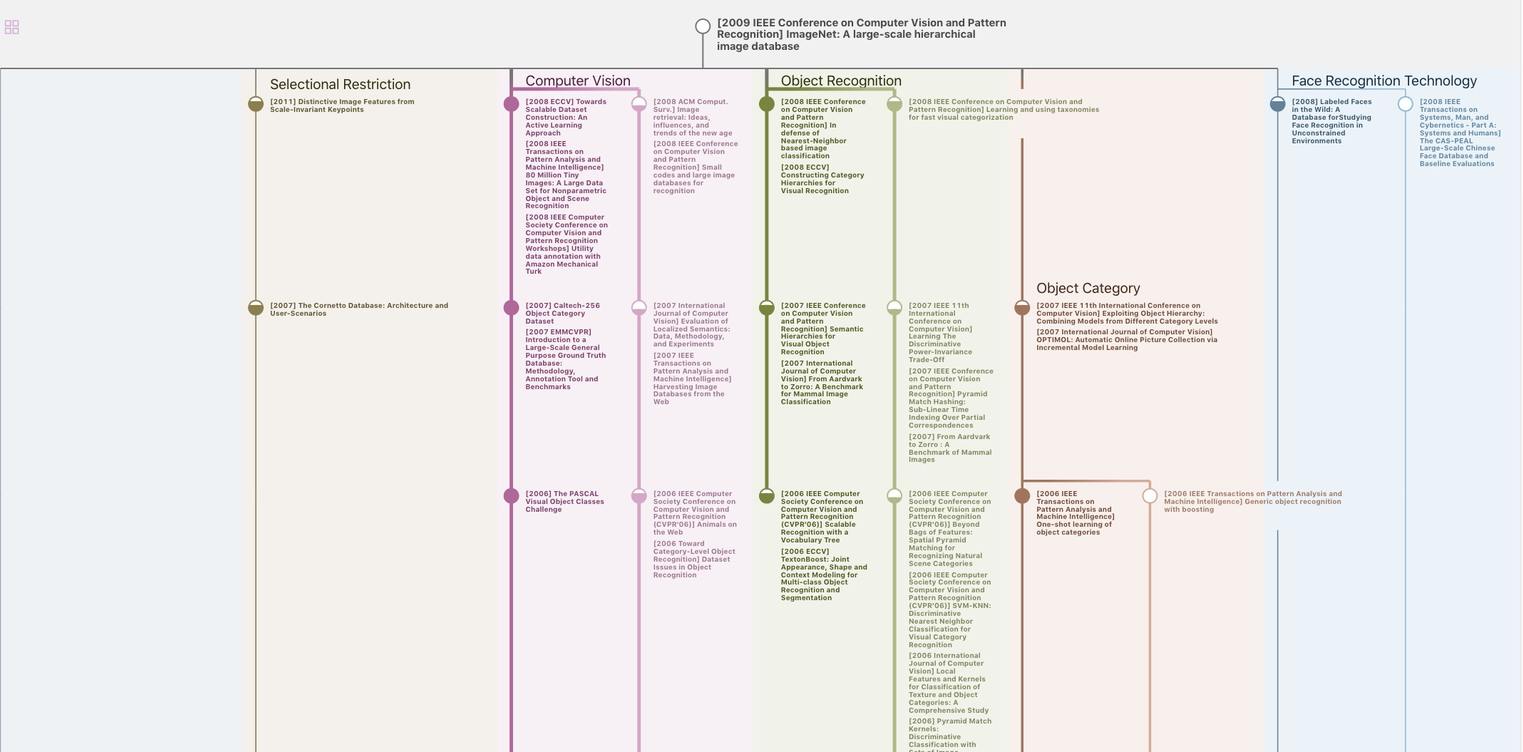
生成溯源树,研究论文发展脉络
Chat Paper
正在生成论文摘要