Metrizing Weak Convergence with Maximum Mean Discrepancies
JOURNAL OF MACHINE LEARNING RESEARCH(2023)
摘要
This paper characterizes the maximum mean discrepancies (MMD) that metrize the weak convergence of probability measures for a wide class of kernels. More precisely, we prove that, on a locally compact, non-compact, Hausdorff space, the MMD of a bounded continuous Borel measurable kernel k, whose RKHS-functions vanish at infinity (i.e., Hk subset of C0), metrizes the weak convergence of probability measures if and only if k is continuous and integrally strictly positive definite (f s.p.d.) over all signed, finite, regular Borel measures. We also there exist both bounded continuous f s.p.d. kernels that do not metrize weak convergence correct a prior result of Simon-Gabriel and Scholkopf (JMLR 2018, Thm. 12) by showing that and bounded continuous non -f s.p.d. kernels that do metrize it.
更多查看译文
关键词
Maximum Mean Discrepancy,Metrization of weak convergence,Kernel mean embeddings,Characteristic kernels,Integrally strictly positive definite kernels
AI 理解论文
溯源树
样例
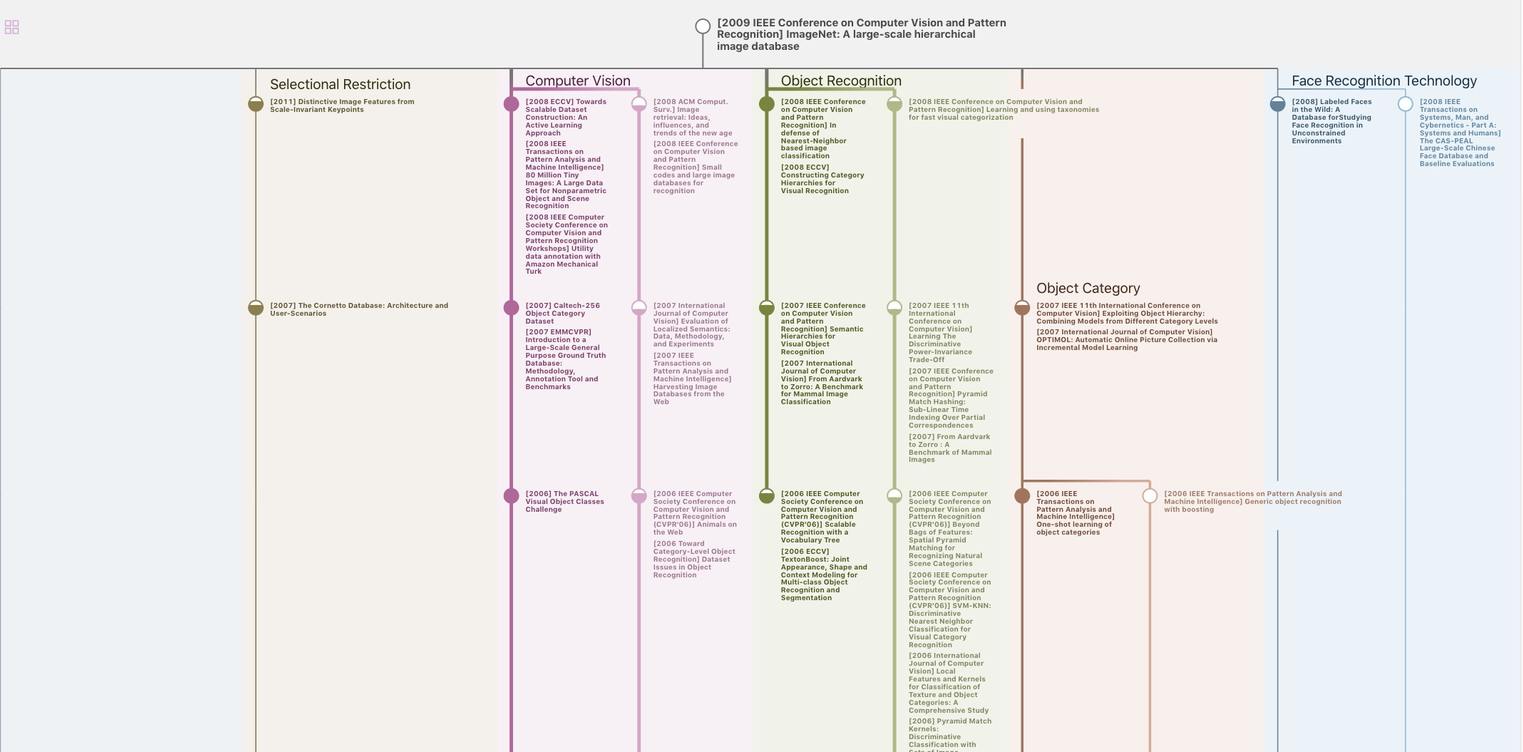
生成溯源树,研究论文发展脉络
Chat Paper
正在生成论文摘要