The 2013 sesame multimedia event detection and recounting system
Proceedings of TRECVID Workshop(2013)
摘要
The SESAME (video SEarch with Speed and Accuracy for Multimedia Events) team submitted six runs as a full participant in the Multimedia Event Detection (MED) and Multimedia Event Recounting (MER) evaluations. The SESAME system combines low-level visual, audio, and motion features; high-level semantic concepts for visual objects, scenes, persons, sounds, and actions; automatic speech recognition (ASR); and video optical character recognition (OCR). These three types of features and five types of concepts were used in eight event classifiers. One of the event classifiers, VideoStory, is a new approach that exploits the relationship between semantic concepts and imagery in a large training corpus. The SESAME system uses a total of over 18,000 concepts. We combined the event-detection results for these classifiers using a log-likelihood ratio (LLR) late-fusion method, which uses logistic regression to learn combination weights for event-detection scores from multiple classifiers originating from different data types. The SESAME system generated event recountings based on visual and action concepts, and on concepts recognized by ASR and OCR. Training data included the MED Research dataset, ImageNet, a video dataset from YouTube, the UCF101 and HMDB51 action datasets, the NIST SIN dataset, and Wikipedia. The components that contributed most significantly to event-detection performance were the low-and high-level visual features, low-level motion features, and VideoStory. The LLR late-fusion method significantly improved performance over the best individual classifier for 100Ex and 010Ex. For the Semantic Query …
更多查看译文
AI 理解论文
溯源树
样例
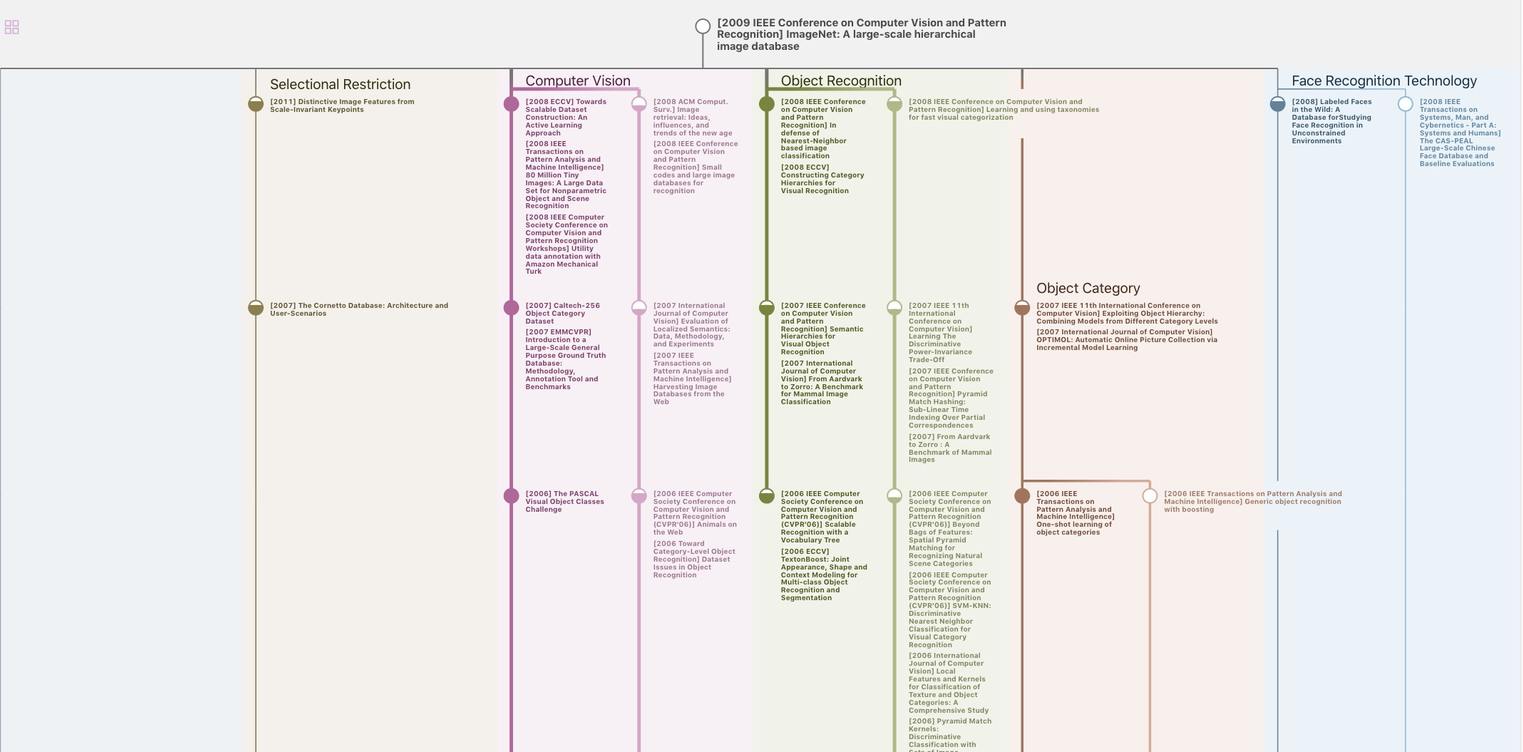
生成溯源树,研究论文发展脉络
Chat Paper
正在生成论文摘要