Active Learning of Relationship-Based Access Control Policies
SACMAT '20: The 25th ACM Symposium on Access Control Models and Technologies Barcelona Spain June, 2020(2020)
摘要
Understanding access control policies is essential in understanding the security behavior of systems. However, often times, a complete and accurate specification of the enforced access control policy in a system is not available. In fact, scale and complexity of a system, or unavailability of its source code, may prevent users and even its developers from having access to such accurate specification. In this paper, we propose a novel, systematic approach for learning access control policies where target systems are treated as black boxes. In particular, we show how we can construct a deterministic finite automaton (DFA) characterizing the relationship-based access control (ReBAC) policy of a system by interacting with its access control engine using minimal number of access requests. Our experiments on realistic application scenarios and their promising results demonstrate the feasibility, scalability and efficiency of our learning approach.
更多查看译文
关键词
relationship-based access control, authorization, black box, model learning, active learning
AI 理解论文
溯源树
样例
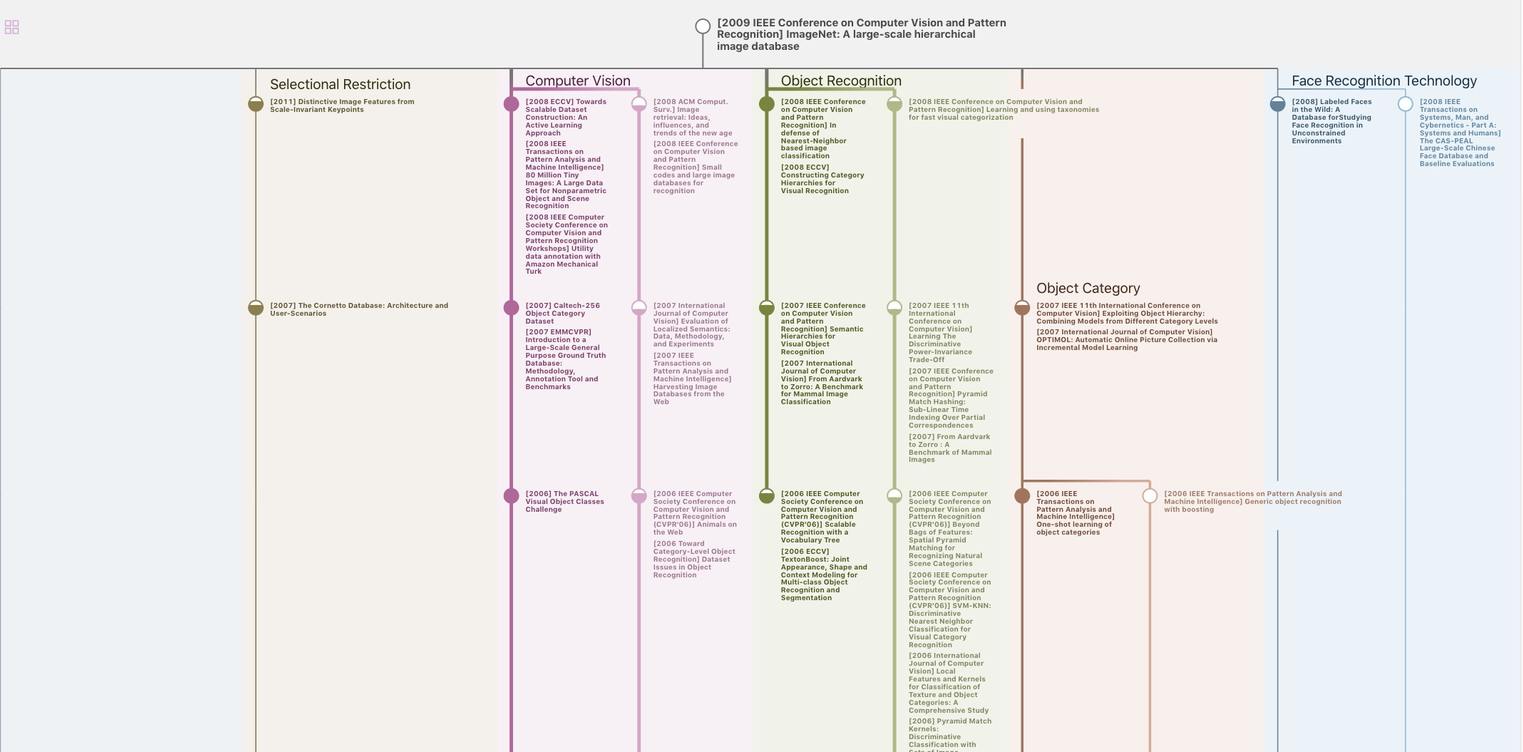
生成溯源树,研究论文发展脉络
Chat Paper
正在生成论文摘要