Better Safe Than Sorry: Risk-Aware Nonlinear Bayesian Estimation
2020 IEEE INTERNATIONAL CONFERENCE ON ACOUSTICS, SPEECH, AND SIGNAL PROCESSING(2020)
摘要
Despite the simplicity and intuitive interpretation of minimum mean squared error (MMSE) estimators, their effectiveness in certain scenarios is questionable. Indeed, minimizing squared errors on average does not provide any form of stability, as the volatility of the estimation error is left unconstrained. When this volatility is statistically significant, the difference between the average and realized performance of the MMSE estimator can be drastically different. To address this issue, we introduce a new risk-aware MMSE formulation which trades between mean performance and risk by explicitly constraining the expected predictive variance of the involved squared error. We show that, under mild moment boundedness conditions, the corresponding risk-aware optimal solution can be evaluated explicitly, and has the form of an appropriately biased nonlinear MMSE estimator. We further illustrate the effectiveness of our approach via several numerical examples, which also showcase the advantages of risk-aware against risk-neutral MMSE estimation, especially in models involving skewed, heavy-tailed distributions.
更多查看译文
关键词
MMSE Estimation, Constrained Bayesian Estimation, Risk-Aware Optimization, Risk Measures
AI 理解论文
溯源树
样例
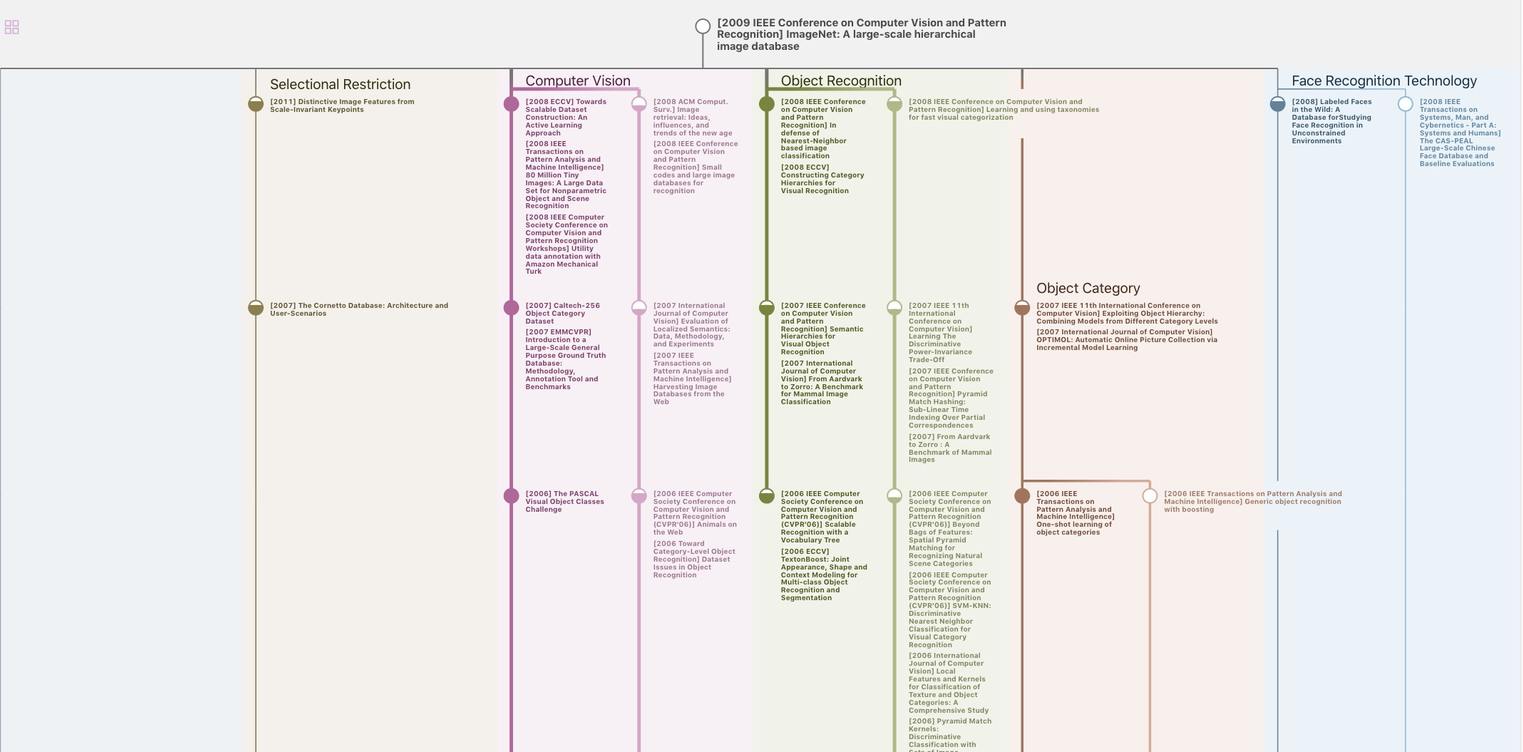
生成溯源树,研究论文发展脉络
Chat Paper
正在生成论文摘要