F2A2: Flexible Fully-decentralized Approximate Actor-critic for Cooperative Multi-agent Reinforcement Learning
JOURNAL OF MACHINE LEARNING RESEARCH(2023)
摘要
Traditional centralized multi-agent reinforcement learning (MARL) algorithms are some -times unpractical in complicated applications due to non-interactivity between agents, the curse of dimensionality, and computation complexity. Hence, several decentralized MARL algorithms are motivated. However, existing decentralized methods only handle the fully cooperative setting where massive information needs to be transmitted in training. The block coordinate gradient descent scheme they used for successive independent actor and critic steps can simplify the calculation, but it causes serious bias. This paper proposes a flexible fully decentralized actor-critic MARL framework, which can combine most of the actor-critic methods and handle large-scale general cooperative multi-agent settings. A primal-dual hybrid gradient descent type algorithm framework is designed to learn indi-
更多查看译文
AI 理解论文
溯源树
样例
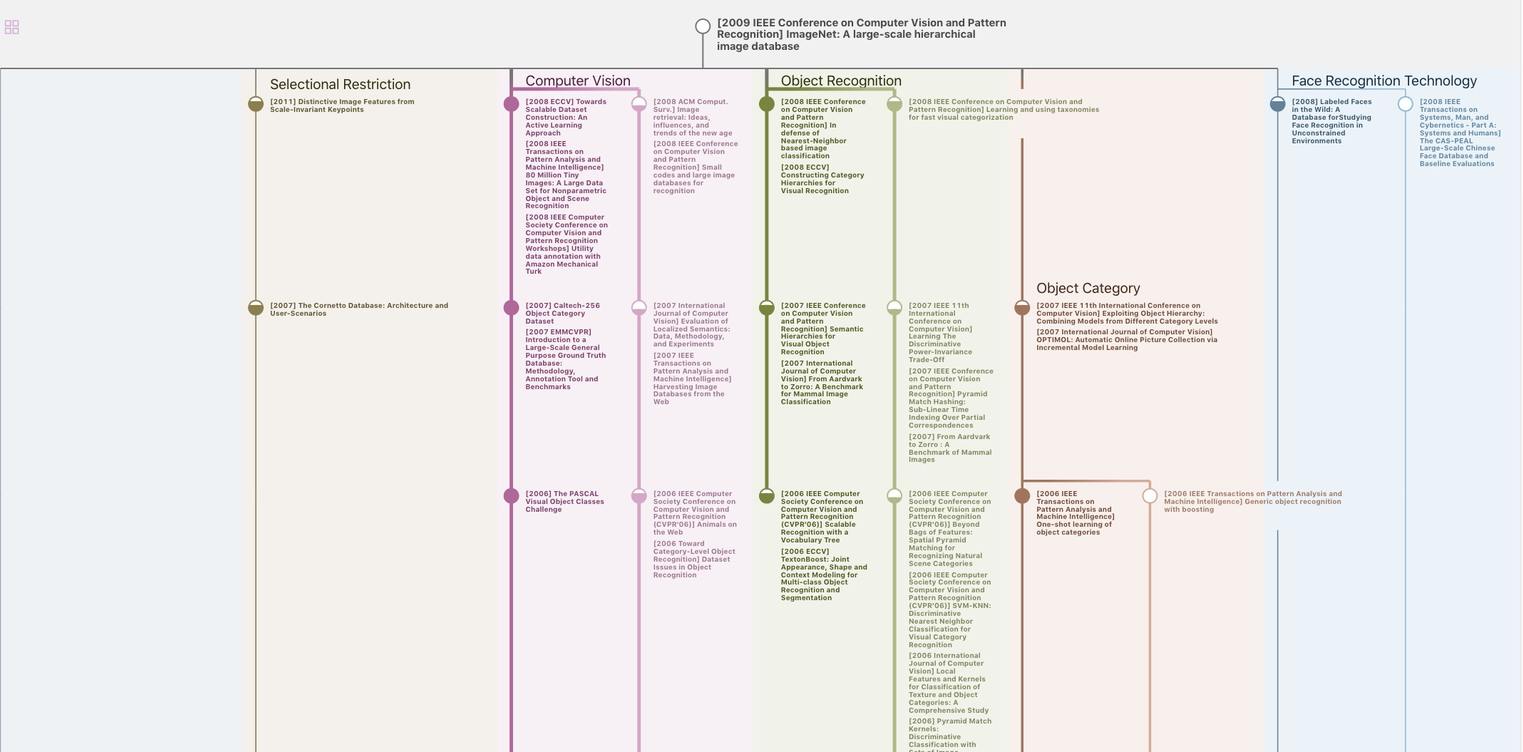
生成溯源树,研究论文发展脉络
Chat Paper
正在生成论文摘要