Data Dieting in GAN Training
arxiv(2020)
摘要
We investigate training Generative Adversarial Networks, GANs, with less data. Subsets of the training dataset can express empirical sample diversity while reducing training resource requirements, e.g. time and memory. We ask how much data reduction impacts generator performance and gauge the additive value of generator ensembles. In addition to considering stand-alone GAN training and ensembles of generator models, we also consider reduced data training on an evolutionary GAN training framework named Redux-Lipizzaner. Redux-Lipizzaner makes GAN training more robust and accurate by exploiting overlapping neighborhood-based training on a spatial 2D grid. We conduct empirical experiments on Redux-Lipizzaner using the MNIST and CelebA data sets.
更多查看译文
关键词
gan,data dieting,training
AI 理解论文
溯源树
样例
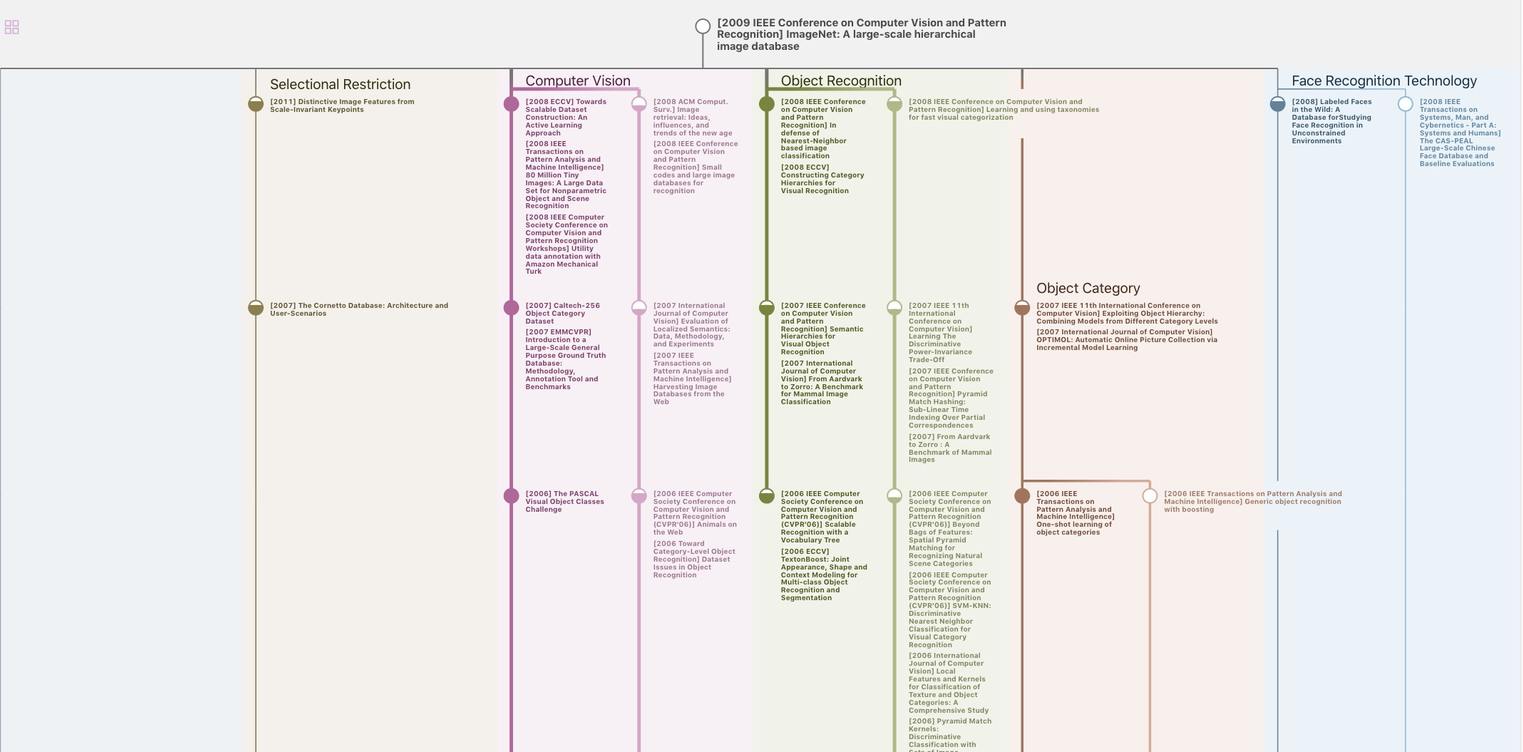
生成溯源树,研究论文发展脉络
Chat Paper
正在生成论文摘要