Block-level double JPEG compression detection for image forgery localization
Multimedia Tools and Applications(2024)
摘要
Forged images in JPEG format have a ubiquitous presence in today’s world due to the ease of availability of image manipulation tools. This paper presents a deep learning-based system that utilizes the inherent relationship between histograms of quantized DCT coefficients and corresponding quantization step sizes to distinguish between original and forged regions in a JPEG image, based on detecting single and double compressed blocks, without fully decompressing the JPEG image. In this direction, we propose a novel combination of raw histograms of the quantized DCT coefficients and corresponding quantization step sizes and use it as input to the counterpart standard CNN architectures designed to handle the proposed input representation for single vs. double JPEG compression detection. The proposed input is shown to have a minimal effect on performance with various standard CNN architectures that are designed to handle the proposed input for the task at hand. Specifically, we have utilized DenseNet to extract the compression-specific artifacts from the proposed input for additional experiments reported in this work. We considered a publicly available dataset generated with a diverse set of 1,120 quantization matrices. Using the proposed input to learn the compression artifacts outperforms the baseline methods for the blocks of sizes 256 × 256 , 128 × 128 , and 64 × 64 . Furthermore, in the case of test blocks compressed with completely different quantization matrices than matrices used in training, the proposed method outperforms the baseline methods. Consequently, improved forgery localization performances are obtained for forged JPEG images.
更多查看译文
关键词
Image forensics,Compression artifacts,Quantization matrix,DenseNet,Unseen compression
AI 理解论文
溯源树
样例
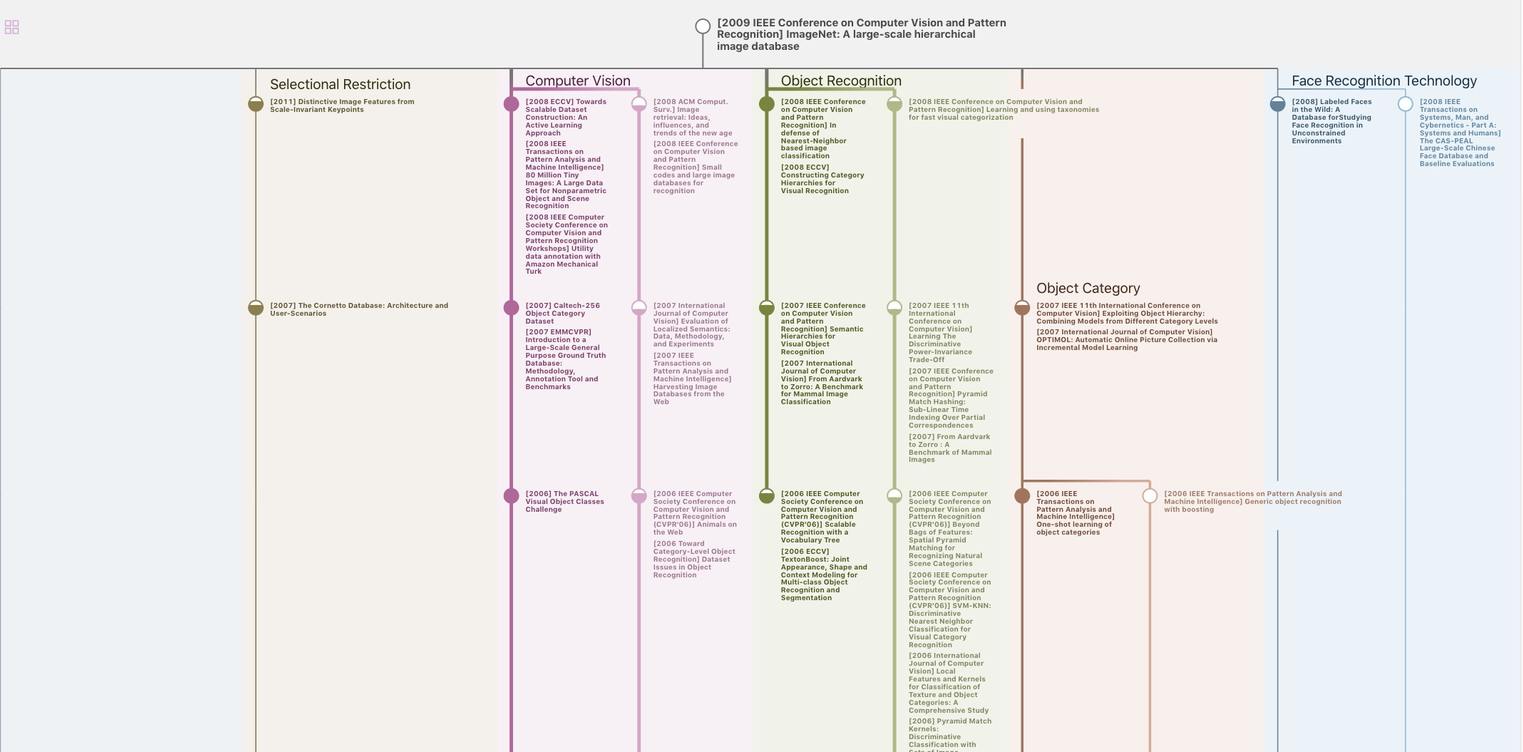
生成溯源树,研究论文发展脉络
Chat Paper
正在生成论文摘要