XtarNet: Learning to Extract Task-Adaptive Representation for Incremental Few-Shot Learning
ICML(2020)
摘要
Learning novel concepts while preserving prior knowledge is a long-standing challenge in machine learning. The challenge gets greater when a novel task is given with only a few labeled examples, a problem known as incremental few-shot learning. We propose XtarNet, which learns to extract task-adaptive representation (TAR) for facilitating incremental few-shot learning. The method utilizes a backbone network pretrained on a set of base categories while also employing additional modules that are meta-trained across episodes. Given a new task, the novel feature extracted from the meta-trained modules is mixed with the base feature obtained from the pretrained model. The process of combining two different features provides TAR and is also controlled by meta-trained modules. The TAR contains effective information for classifying both novel and base categories. The base and novel classifiers quickly adapt to a given task by utilizing the TAR. Experiments on standard image datasets indicate that XtarNet achieves state-of-the-art incremental few-shot learning performance. The concept of TAR can also be used in conjunction with existing incremental few-shot learning methods; extensive simulation results in fact show that applying TAR enhances the known methods significantly.
更多查看译文
关键词
learning,representation,task-adaptive,few-shot
AI 理解论文
溯源树
样例
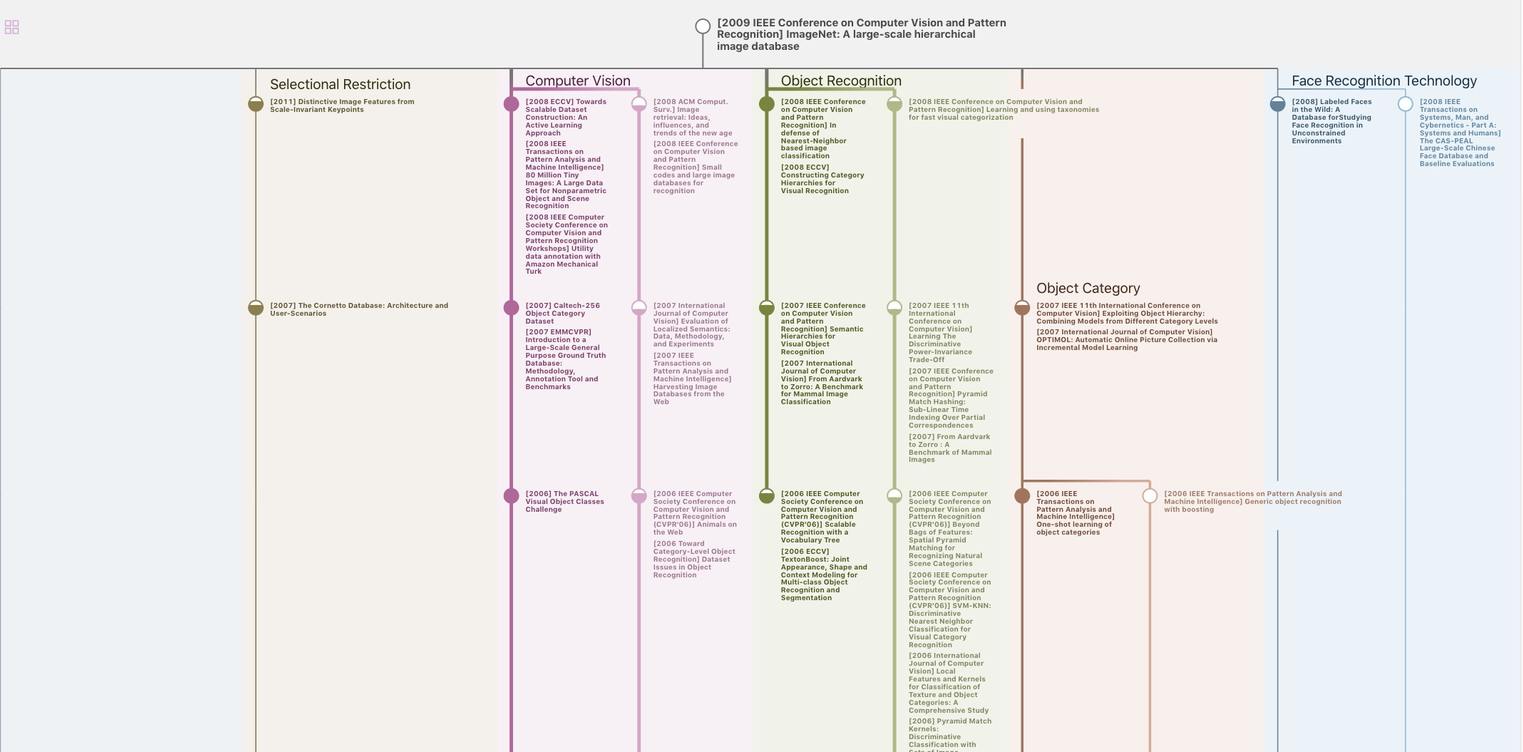
生成溯源树,研究论文发展脉络
Chat Paper
正在生成论文摘要