ML-AQP: Query-Driven Approximate Query Processing based on Machine Learning
arxiv(2020)
摘要
As more and more organizations rely on data-driven decision making, large-scale analytics become increasingly important. However, an analyst is often stuck waiting for an exact result. As such, organizations turn to Cloud providers that have infrastructure for efficiently analyzing large quantities of data. But, with increasing costs, organizations have to optimize their usage. Having a cheap alternative that provides speed and efficiency will go a long way. Concretely, we offer a solution that can provide approximate answers to aggregate queries, relying on Machine Learning (ML), which is able to work alongside Cloud systems. Our developed lightweight ML-led system can be stored on an analyst's local machine or deployed as a service to instantly answer analytic queries, having low response times and monetary/computational costs and energy footprint. To accomplish this we leverage the knowledge obtained by previously answered queries and build ML models that can estimate the result of new queries in an efficient and inexpensive manner. The capabilities of our system are demonstrated using extensive evaluation with both real and synthetic datasets/workloads and well known benchmarks.
更多查看译文
AI 理解论文
溯源树
样例
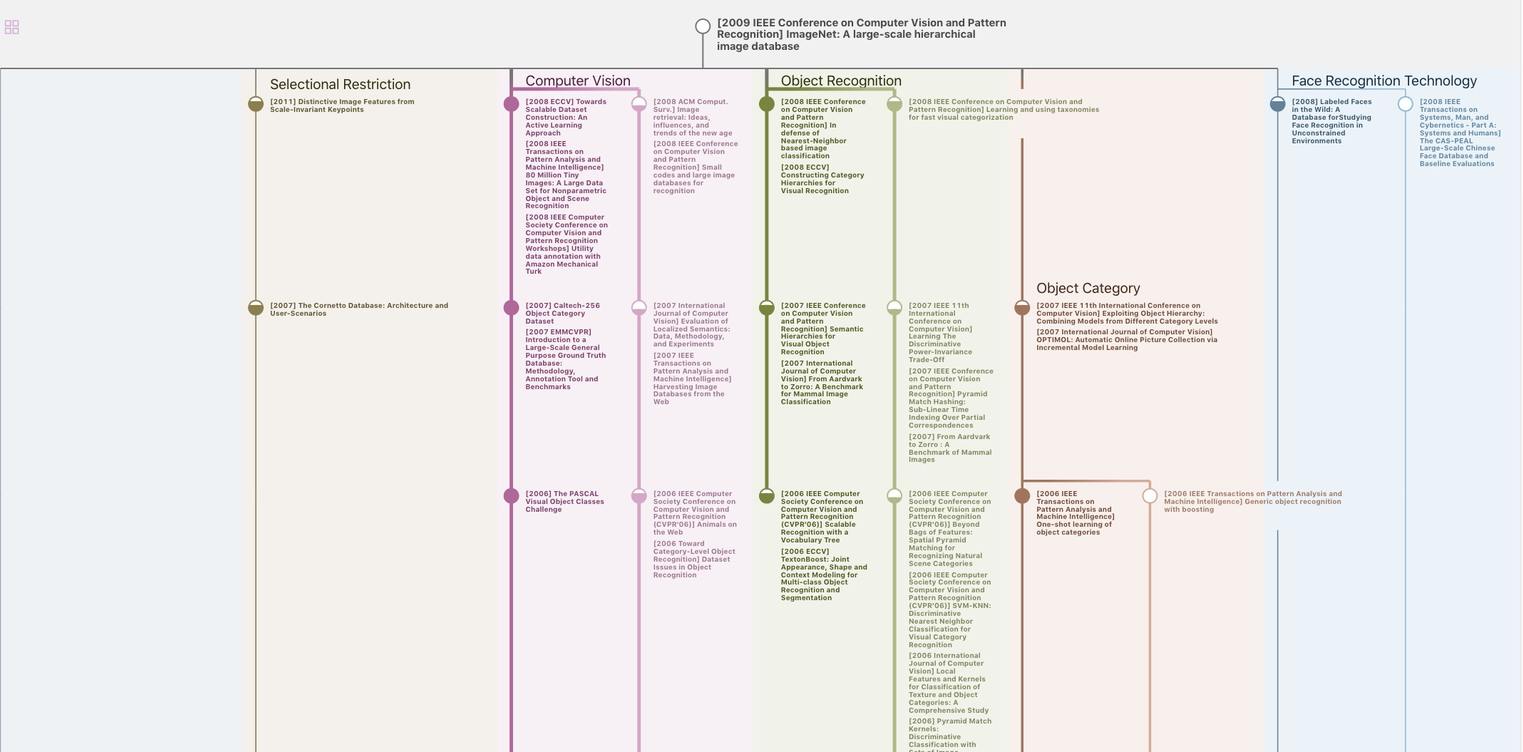
生成溯源树,研究论文发展脉络
Chat Paper
正在生成论文摘要