Semantically Consistent Hierarchical Text To Fashion Image Synthesis With An Enhanced-Attentional Generative Adversarial Network
2019 IEEE/CVF INTERNATIONAL CONFERENCE ON COMPUTER VISION WORKSHOPS (ICCVW)(2019)
摘要
In this paper, we present the enhanced Attentional Generative Adversarial Network (e-AttnGAN) with improved training stability for text-to-image synthesis. e-AttnGAN's integrated attention module utilizes both sentence and word context features and performs feature-wise linear modulation (FiLM) to fuse visual and natural language representations. In addition to multimodal similarity learning for text and image features of AttnGAN [28], cosine and feature matching losses of real and generated images are included while employing a classification loss for "significant attributes". In order to improve the stability of the training and solve the issue of model collapse, spectral normalization and two-time scale update for the discriminator are used together with instance noise. Our experiments show that e-AttnGAN outperforms state-of-the-art methods on the FashionGen and DeepFashion-Synthesis datasets.
更多查看译文
关键词
text to image,image synthesis,generative adversarial networks
AI 理解论文
溯源树
样例
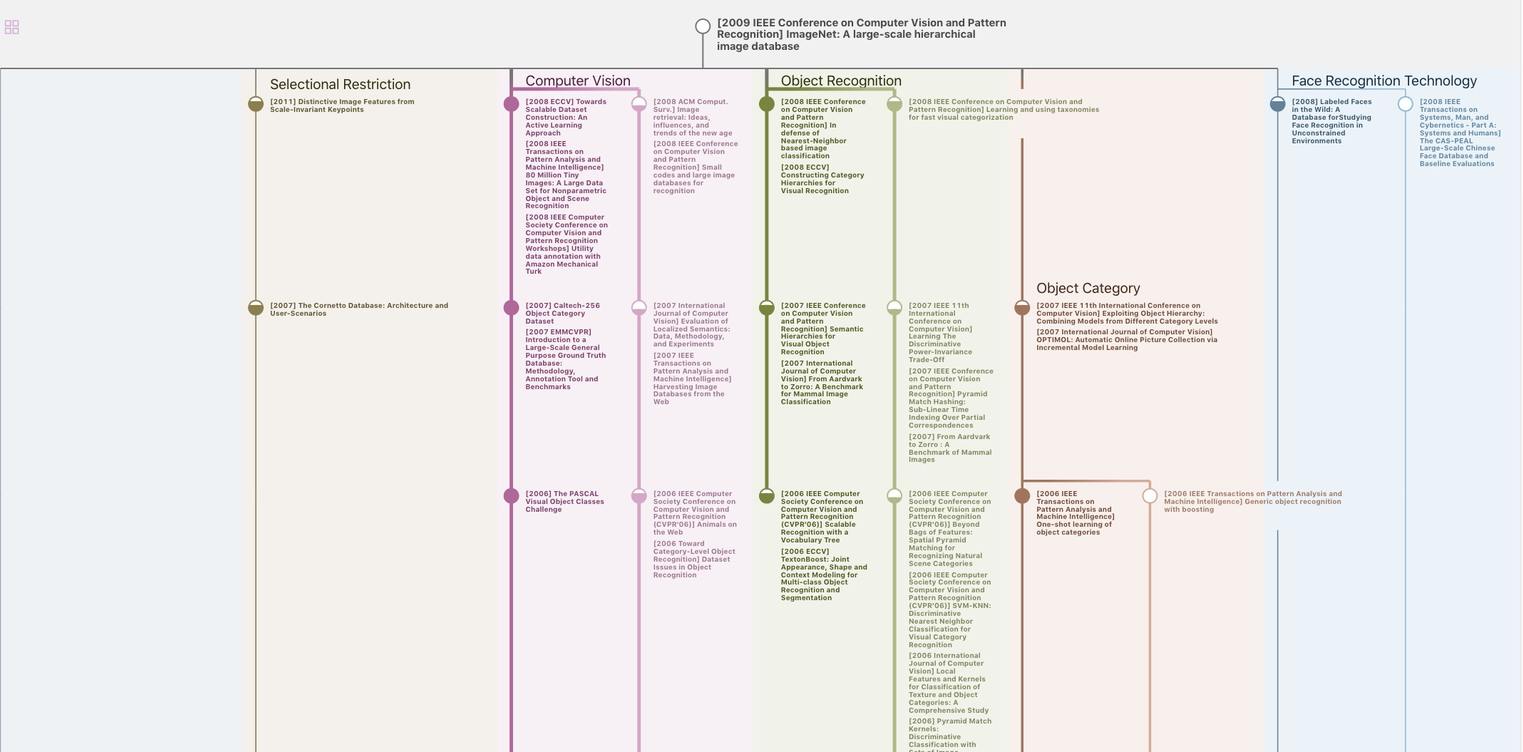
生成溯源树,研究论文发展脉络
Chat Paper
正在生成论文摘要